Harmful Communication Detection of Toxic Language and Threats on Swedish
PROCEEDINGS OF THE 2023 IEEE/ACM INTERNATIONAL CONFERENCE ON ADVANCES IN SOCIAL NETWORKS ANALYSIS AND MINING, ASONAM 2023(2023)
Abstract
Harmful communication, such as toxic language and threats directed toward individuals or groups, is a common problem on most social media platforms and online spaces. While several approaches exist for detecting toxic language and threats in English, few attempts have detected such communication in Swedish. Thus, we used transfer learning and BERT to train two machine learning models: one that detects toxic language and one that detects threats in Swedish. We also examined the intersection between toxicity and threat. The models are trained on data from several different sources, with authentic social media posts and data translated from English. Our models perform well on test data with an F1-score above 0.94 for detecting toxic language and 0.86 for detecting threats. However, the models' performance decreases significantly when they are applied to new unseen social media data. Examining the intersection between toxic language and threats, we found that 20% of the threats on social media are not toxic, which means that they would not be detected using only methods for detecting toxic language. Our finding highlights the difficulties with harmful language and the need to use different methods to detect different kinds of harmful language.
MoreTranslated text
AI Read Science
Must-Reading Tree
Example
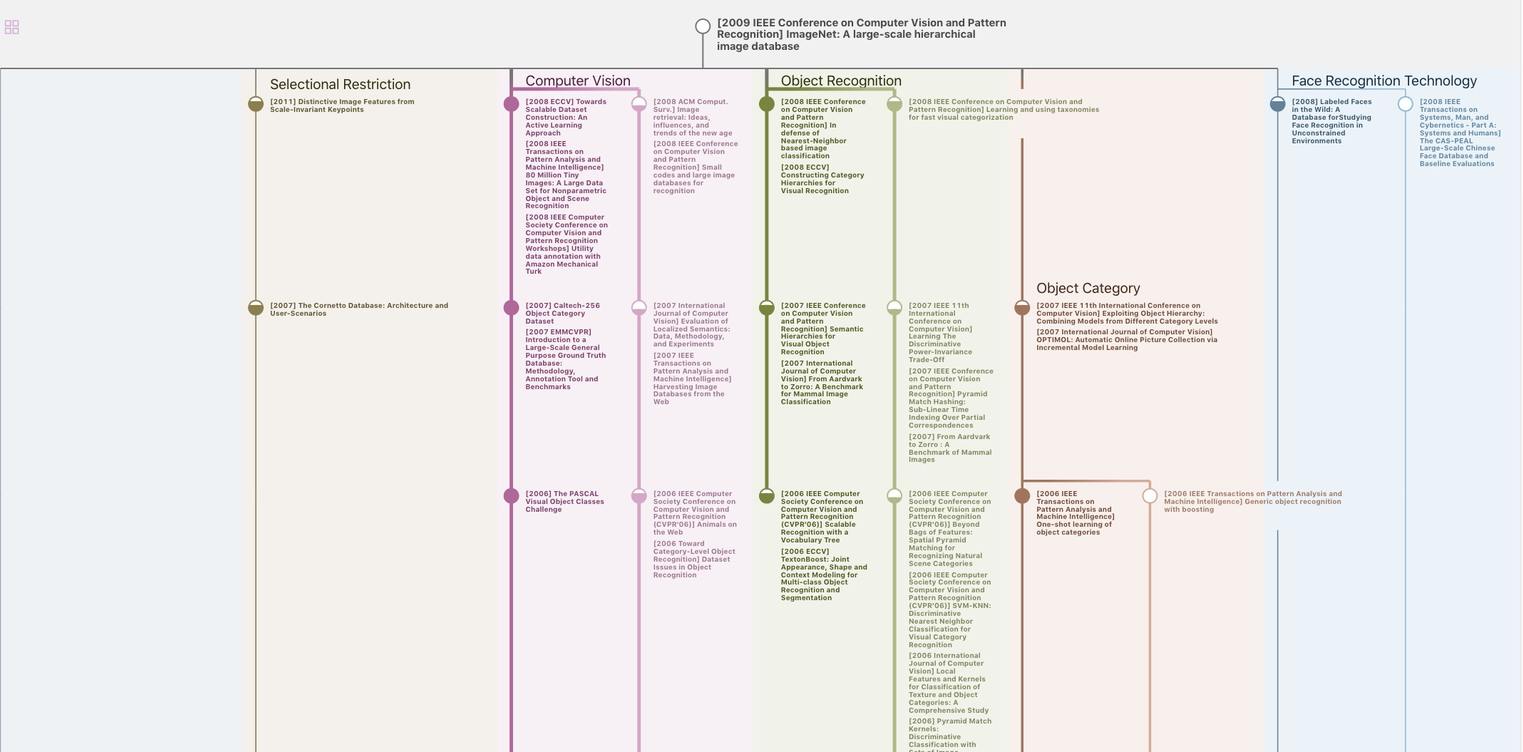
Generate MRT to find the research sequence of this paper
Chat Paper
Summary is being generated by the instructions you defined