Ranking and Selection: A New Sequential Bayesian Procedure for Use with Common Random Numbers
ACM Transactions on Modeling and Computer Simulation(2019)
摘要
We want to select the best systems out of a given set of systems (or rank them) with respect to their expected performance. The systems allow random observations only and we assume that the joint observation of the systems has a multivariate normal distribution with unknown mean and covariance. We allow dependent marginal observations as they occur when common random numbers are used for the simulation of the systems. In particular, we focus on positively dependent observations as they might be expected in heuristic optimization where `systems' are different solutions to an optimization problem with common random inputs. In each iteration, we allocate a fixed budget of simulation runs to the solutions. We use a Bayesian setup and allocate the simulation effort according to the posterior covariances of the solutions until the ranking and selection decision is correct with a given high probability. Here, the complex posterior distributions are approximated only but we give extensive empirical evidence that the observed error probabilities are well below the given bounds in most cases. We also use a generalized scheme for the target of the ranking and selection that allows to bound the error probabilities with a Bonferroni approach. Our test results show that our procedure uses less simulations than comparable procedures from literature even in most of the cases where the observations are not positively correlated.
更多查看译文
关键词
Ranking and selection,Bayesian model,unknown covariance,approximate posterior,ordinal optimization,missing data
AI 理解论文
溯源树
样例
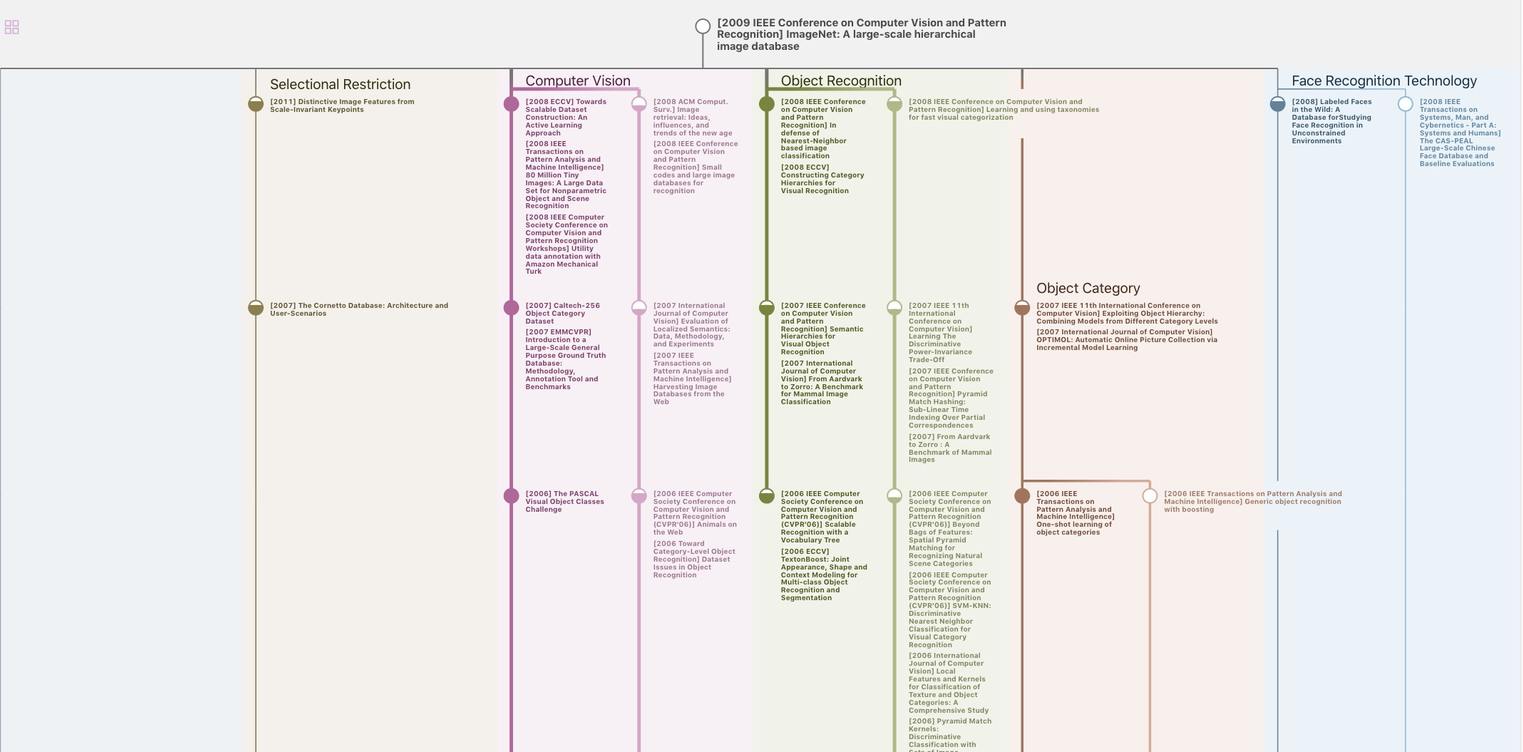
生成溯源树,研究论文发展脉络
Chat Paper
正在生成论文摘要