Domain Adaptation for Opinion Classification: A Self-Training Approach
Journal of Information Science Theory and Practice(2013)
摘要
Domain transfer is a widely recognized problem for machine learning algorithms because models built upon one data domain generally do not perform well in another data domain. This is especially a challenge for tasks such as opinion classification, which often has to deal with insufficient quantities of labeled data. This study investigates the feasibility of self-training in dealing with the domain transfer problem in opinion classification via leveraging labeled data in non-target data domain(s) and unlabeled data in the target-domain. Specifically, self-training is evaluated for effectiveness in sparse data situations and feasibility for domain adaptation in opinion classification. Three types of Web content are tested: edited news articles, semi-structured movie reviews, and the informal and unstructured content of the blogosphere. Findings of this study suggest that, when there are limited labeled data, self-training is a promising approach for opinion classification, although the contributions vary across data domains. Significant improvement was demonstrated for the most challenging data domain-the blogosphere-when a domain transfer-based self-training strategy was implemented.
更多查看译文
关键词
Domain adaptation,Opinion classification,Self-training,Semi-supervised learning,Sentiment analysis,Machine learning
AI 理解论文
溯源树
样例
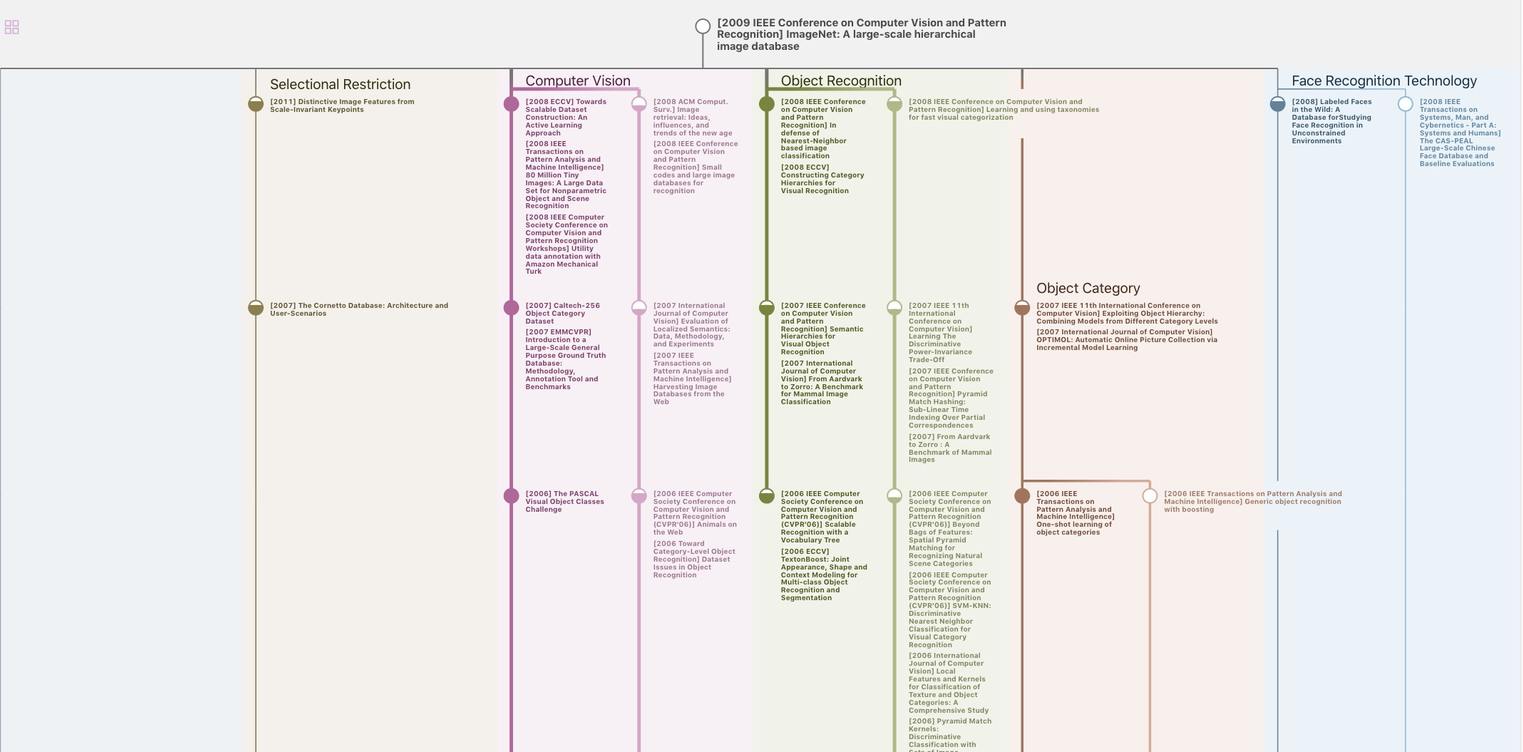
生成溯源树,研究论文发展脉络
Chat Paper
正在生成论文摘要