Predictor augmentation in random forests
STATISTICS AND ITS INTERFACE(2014)
摘要
Random forest (RF) methodology is an increasingly popular nonparametric methodology for prediction in both regression and classification problems. We describe a behavior of random forests (RFs) that may be unknown and surprising to many initial users of the methodology: out-of-sample prediction by RFs can be sometimes improved by augmenting the dataset with a new explanatory variable, independent of all variables in the original dataset. We explain this phenomenon with a simulated example, and show how independent variable augmentation can help RFs to decreases prediction variance and improve prediction performance in some cases. We also give real data examples for illustration, argue that this phenomenon is closely connected with overfitting, and suggest potential research for improving RFs.
更多查看译文
关键词
Classification,Machine learning,Prediction,Regression
AI 理解论文
溯源树
样例
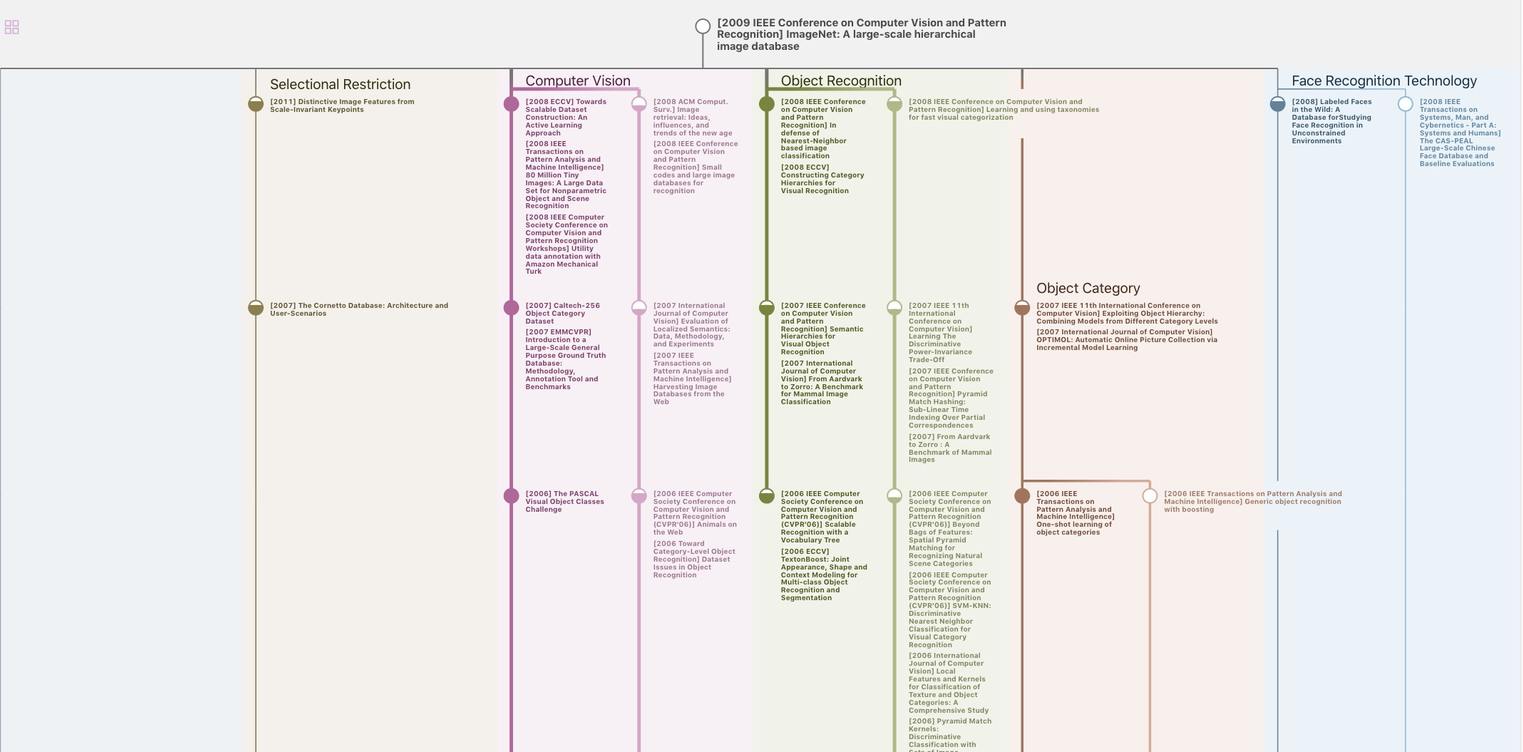
生成溯源树,研究论文发展脉络
Chat Paper
正在生成论文摘要