Robust vessel detection and segmentation in ultrasound images by a data-driven approach
Proceedings of SPIE(2014)
摘要
This paper presents a learning based vessel detection and segmentation method in real-patient ultrasound (US) liver images. We aim at detecting multiple shaped vessels robustly and automatically, including vessels with weak and ambiguous boundaries. Firstly, vessel candidate regions are detected by a data-driven approach. Multi-channel vessel enhancement maps with complement performances are generated and aggregated under a Conditional Random Field (CRF) framework. Vessel candidates are obtained by thresholding the saliency map Secondly, regional features are extracted and the probability of each region being a vessel is modeled by random forest regression Finally, a fast levelset method is developed to refine vessel boundaries. Experiments have been carried out on an US liver dataset with 98 patients. The dataset contains both normal and abnormal liver images. The proposed method in this paper is compared with a traditional Hessian based method, and the average precision is promoted by 56 percents and 7.8 percents for vessel detection and classification, respectively. This improvement shows that our method is more robust to noise, therefore has a better performance than the Hessian based method for the detection of vessels with weak and ambiguous boundaries.
更多查看译文
关键词
Vessel detection,vessel segmentation,vessel enhancement,saliency detection,liver vessel,ultrasound liver
AI 理解论文
溯源树
样例
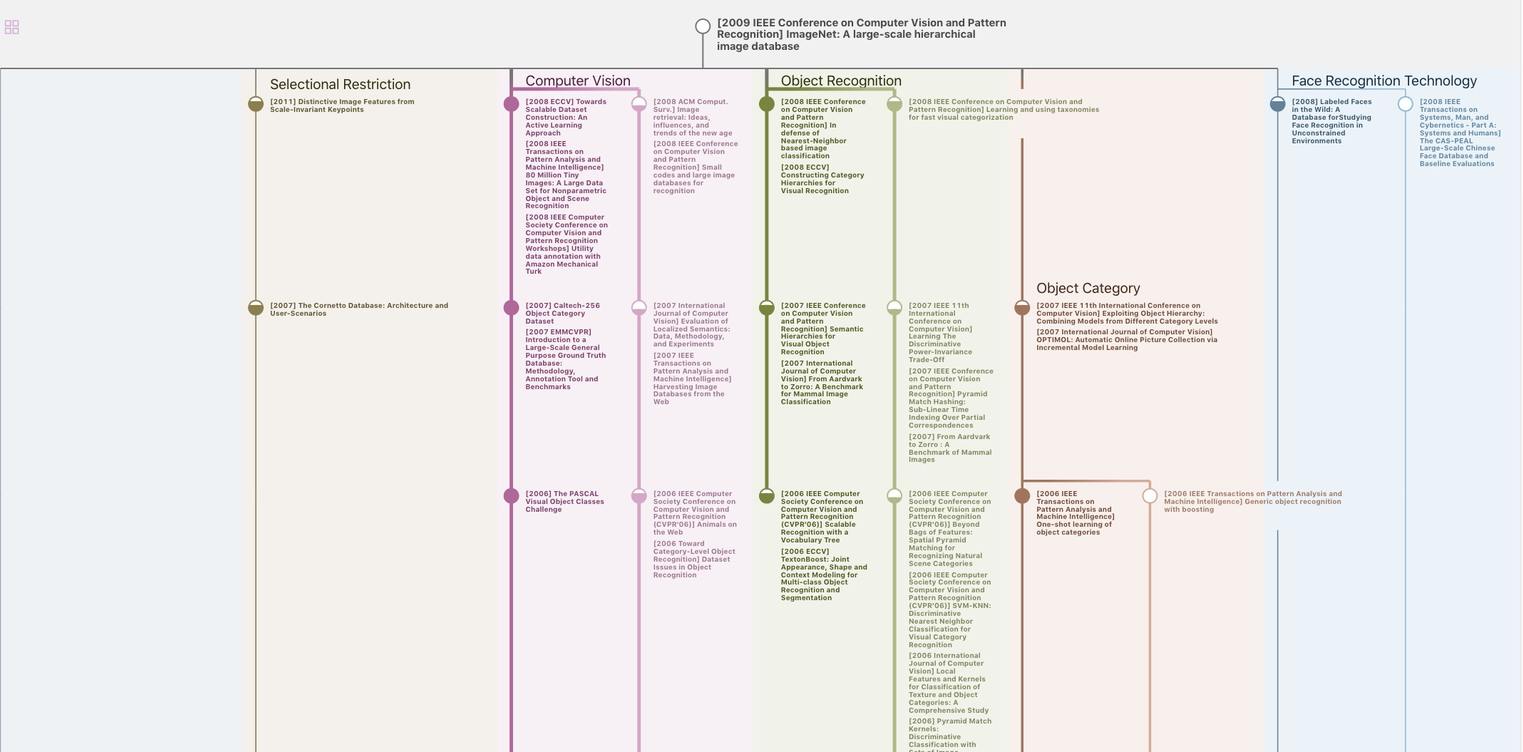
生成溯源树,研究论文发展脉络
Chat Paper
正在生成论文摘要