A Bayesian Spatiotemporal Model Of Panel Design Data: Airborne Particle Number Concentration In Brisbane, Australia
ENVIRONMETRICS(2019)
摘要
In environmental monitoring, the ability to obtain high-quality data across space and time is often limited by the cost of purchasing, deploying and maintaining a large collection of equipment, and the employment of personnel to perform these tasks. An ideal design for a monitoring campaign would be dense enough in time to capture short-range variation at each site, long enough in time to examine trends at each site and across all sites, and dense enough in space to allow modelling of the relationship between the means at each of the sites. This paper outlines a methodology for semiparametric spatiotemporal modelling of data that is dense in time but sparse in space, obtained from a split panel design, the most feasible approach to covering space and time with limited equipment. The data are hourly averaged particle number concentration (PNC) and were collected as part of the International Laboratory for Air Quality and Health's Ultrafine Particles from Traffic Emissions and Children's Health (UPTECH) project. The panel design comprises two weeks of continuous measurements taken at each of a number of government primary schools in the Brisbane Metropolitan Area, with each school visited sequentially. The school data are augmented by data from long-term monitoring stations at three locations in Brisbane, Australia. The temporal part of the model explains daily and weekly cycles in PNC at the schools. The temporal variation is modelled hierarchically with a penalised random walk term common to all sites and a similar term accounting for the remaining temporal trend at each site. The modelling of temporal trends requires an acknowledgement that the observations are correlated rather than independent. At each school and long-term monitoring site, peaks in PNC can be attributed to the morning and afternoon rush hour traffic and new particle formation events. The spatial component of the model describes the school-to-school variation in mean PNC at each school and within each school ground. The spatial term in the model is derived from a stochastic partial differential equation and approximates a Gaussian process with a Gaussian Markov Random field. Fitting the model helps describe spatial and temporal variability at a subset of the UPTECH schools and the long-term monitoring sites, which can be used to estimate the exposure of school children to ultrafine particles. Parameter estimates and their uncertainty are computed in a computationally efficient approximate Bayesian inference environment, R-INLA.
更多查看译文
关键词
air quality,Bayesian inference,environmental exposure,particle number concentration,semiparametric regression,spatiotemporal modelling,ultrafine particles
AI 理解论文
溯源树
样例
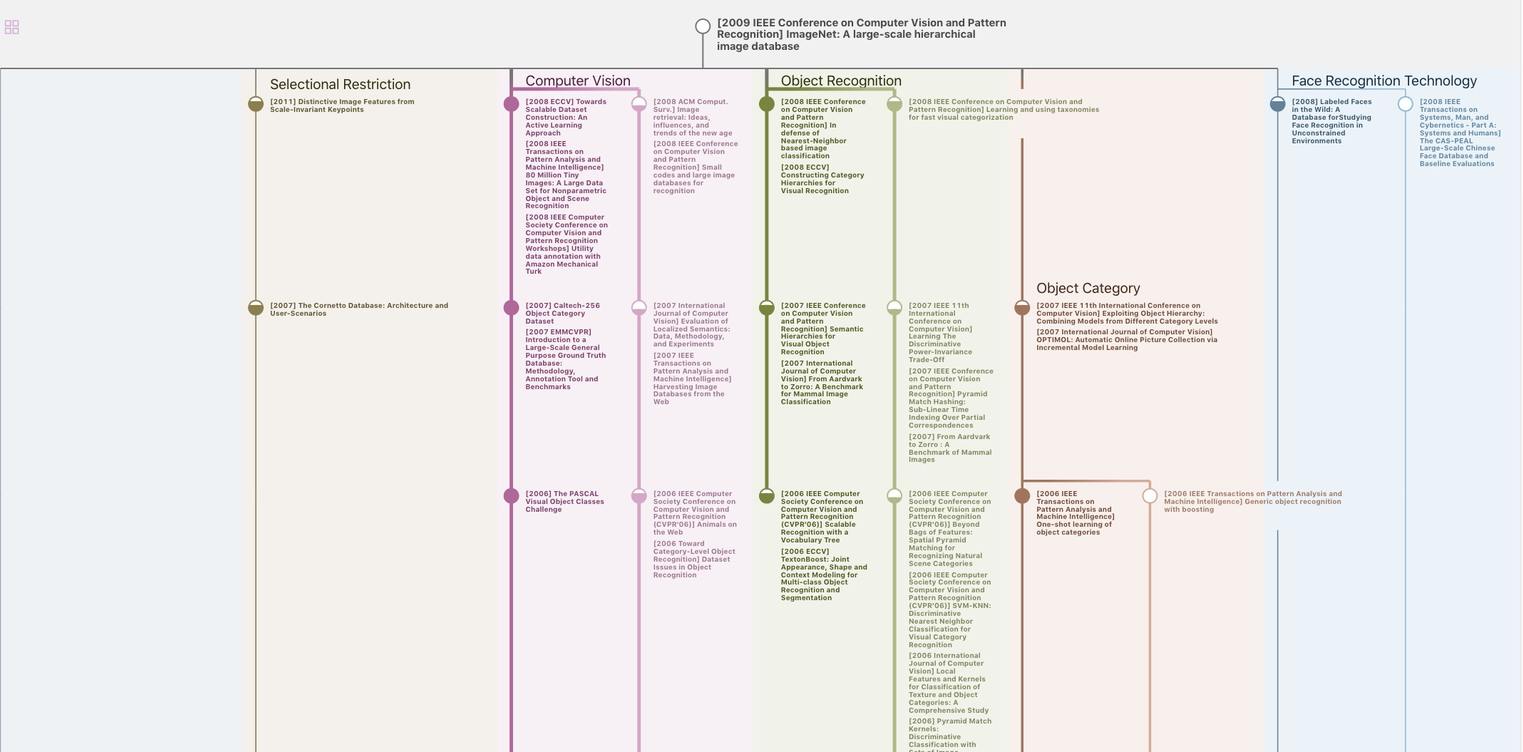
生成溯源树,研究论文发展脉络
Chat Paper
正在生成论文摘要