Estimating Features with Missing Values and Outliers: A Bregman-proximal Point Algorithm for Robust Non-negative Matrix Factorization with Application to Gene Expression Analysis
arXiv (Cornell University)(2015)
摘要
To extract the relevant features in a given dataset is a difficult task, recently resolved in the non-negative data case with the Non-negative Matrix factorization (NMF) method. The objective of this research work is to extend this method to the case of missing and/or corrupted data due to outliers. To do so, data are denoised, missing values are imputed, and outliers are detected while performing a low-rank non-negative matrix factorization of the recovered matrix. To achieve this goal, a mixture of Bregman proximal methods and of the Augmented Lagrangian scheme are used, in a similar way to the so-called Alternating Direction of Multipliers method. An application to the analysis of gene expression data of patients with bladder cancer is finally proposed.
更多查看译文
关键词
gene expression analysis,factorization,bregman proximal admm,outliers,bregman-proximal,non-negative
AI 理解论文
溯源树
样例
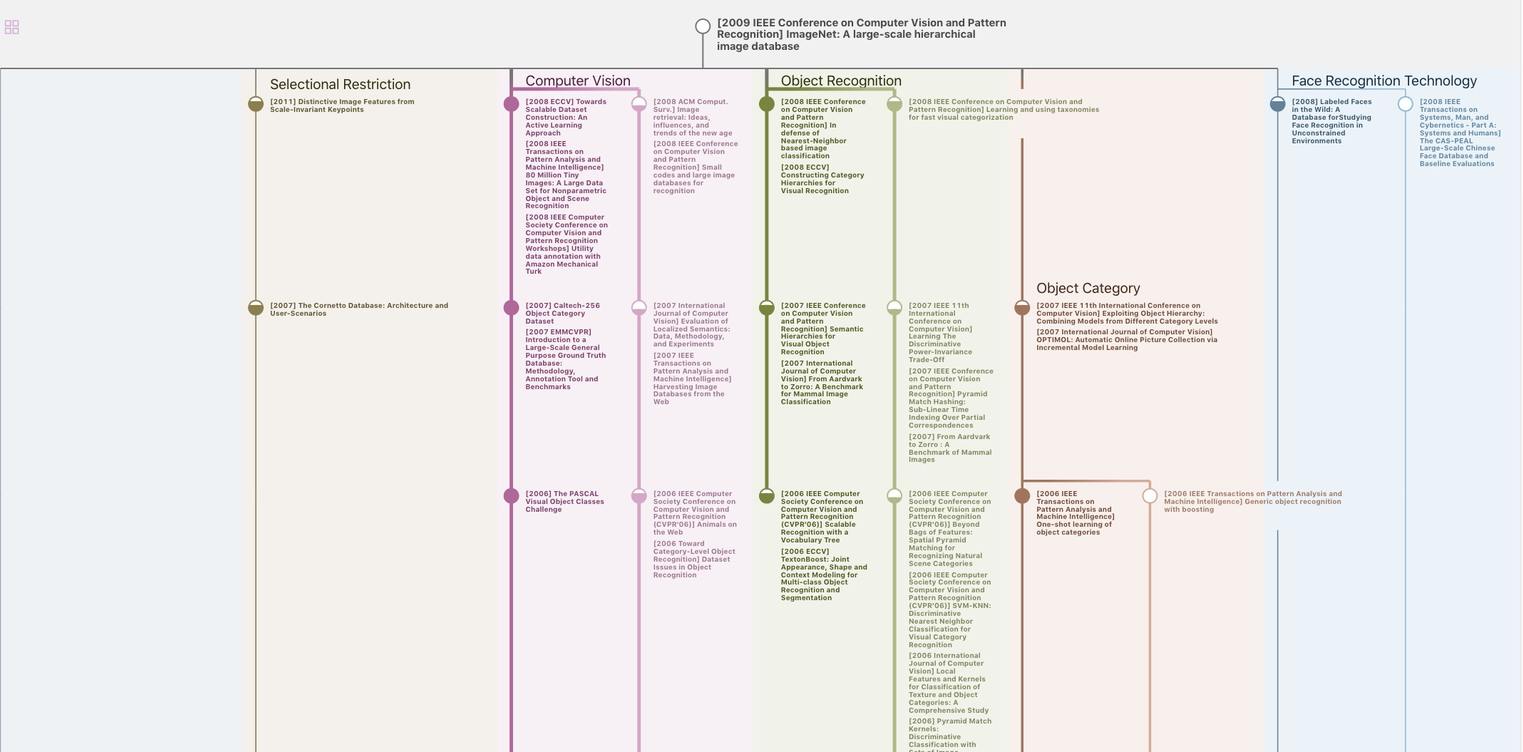
生成溯源树,研究论文发展脉络
Chat Paper
正在生成论文摘要