HMCDA: a novel method based on the heterogeneous graph neural network and metapath for circRNA-disease associations prediction
BMC bioinformatics(2023)
Abstract
Circular RNA (CircRNA) is a type of non-coding RNAs in which both ends are covalently linked. Researchers have demonstrated that many circRNAs can act as biomarkers of diseases. However, traditional experimental methods for circRNA-disease associations identification are labor-intensive. In this work, we propose a novel method based on the heterogeneous graph neural network and metapaths for circRNA-disease associations prediction termed as HMCDA. First, a heterogeneous graph consisting of circRNA-disease associations, circRNA-miRNA associations, miRNA-disease associations and disease-disease associations are constructed. Then, six metapaths are defined and generated according to the biomedical pathways. Afterwards, the entity content transformation, intra-metapath and inter-metapath aggregation are implemented to learn the embeddings of circRNA and disease entities. Finally, the learned embeddings are used to predict novel circRNA-disase associations. In particular, the result of extensive experiments demonstrates that HMCDA outperforms four state-of-the-art models in fivefold cross validation. In addition, our case study indicates that HMCDA has the ability to identify novel circRNA-disease associations.
MoreTranslated text
Key words
Heterogeneous graph neural network, Metapath, CircRNA, Disease
AI Read Science
Must-Reading Tree
Example
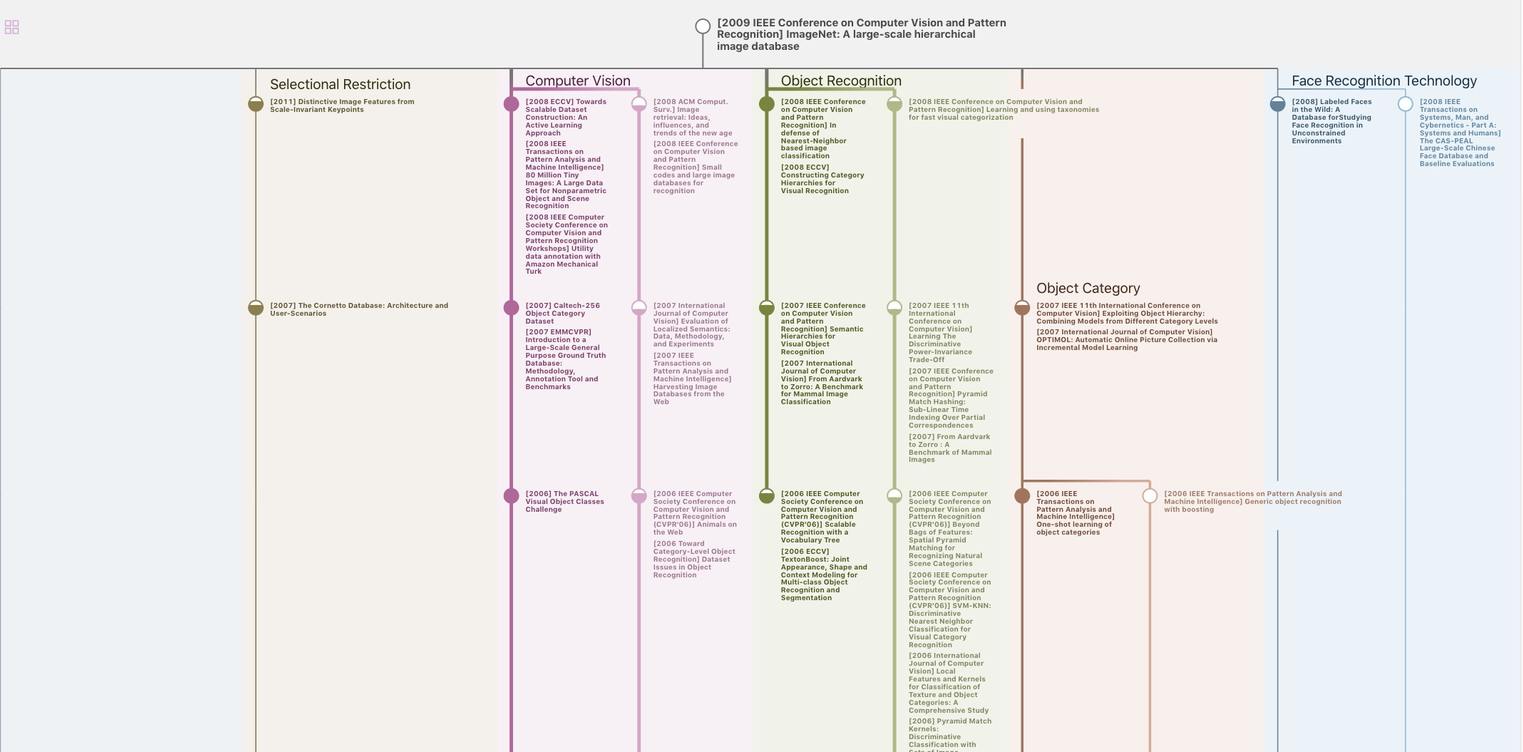
Generate MRT to find the research sequence of this paper
Chat Paper
Summary is being generated by the instructions you defined