A testing based approach to the discovery of differentially correlated variable sets
ANNALS OF APPLIED STATISTICS(2018)
摘要
Given data obtained under two sampling conditions, it is often of interest to identify variables that behave differently in one condition than in the other. We introduce a method for differential analysis of second-order behavior called Differential Correlation Mining (DCM). The DCM method identifies differentially correlated sets of variables, with the property that the average pairwise correlation between variables in a set is higher under one sample condition than the other. DCM is based on an iterative search procedure that adaptively updates the size and elements of a candidate variable set. Updates are performed via hypothesis testing of individual variables, based on the asymptotic distribution of their average differential correlation. We investigate the performance of DCM by applying it to simulated data as well as to recent experimental datasets in genomics and brain imaging.
更多查看译文
关键词
Differential correlation mining,association mining,biostatistics,genomics,high-dimensional data
AI 理解论文
溯源树
样例
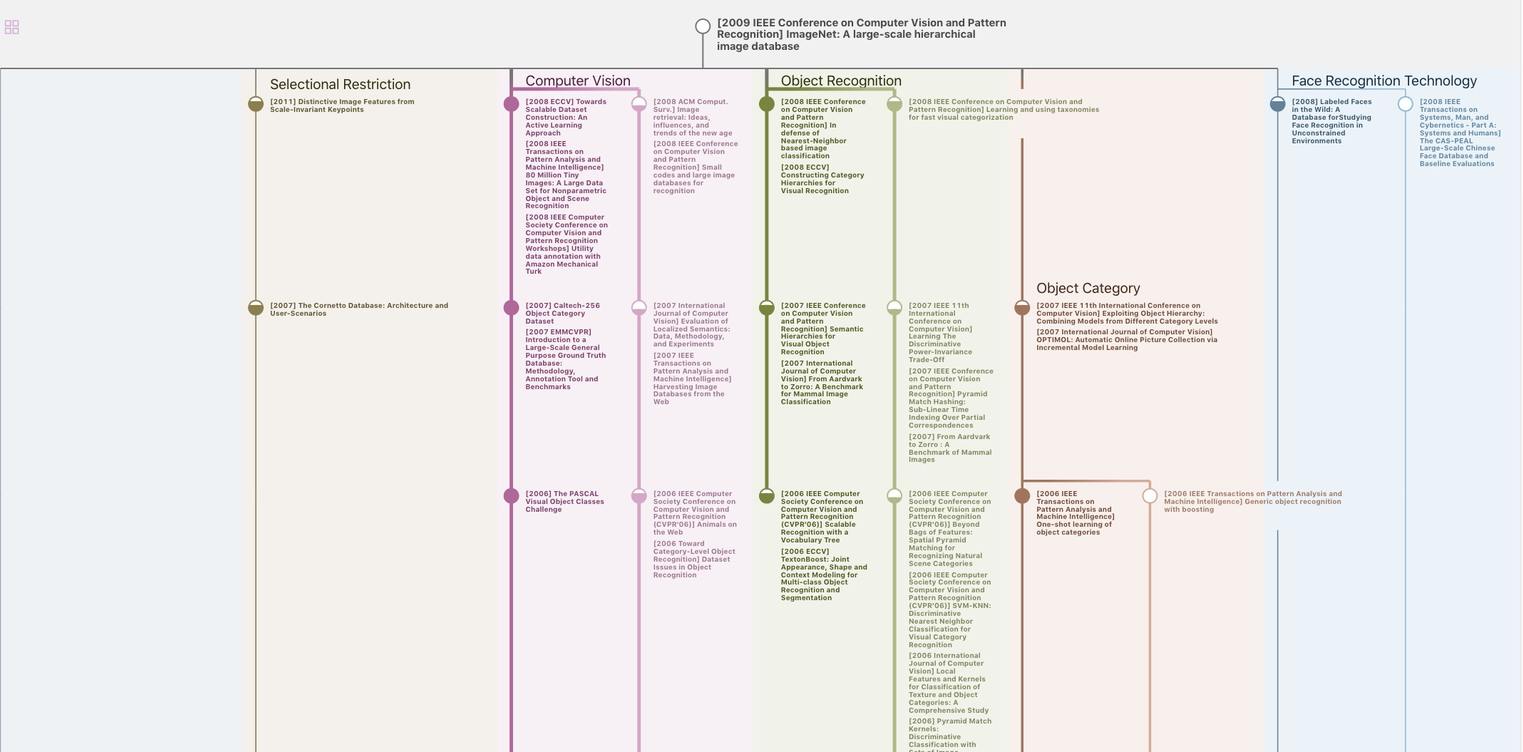
生成溯源树,研究论文发展脉络
Chat Paper
正在生成论文摘要