Natural language processing of admission notes to predict severe maternal morbidity during the delivery encounter.
American journal of obstetrics and gynecology(2022)
Abstract
BACKGROUND:Severe maternal morbidity and mortality remain public health priorities in the United States, given their high rates relative to other high-income countries and the notable racial and ethnic disparities that exist. In general, accurate risk stratification methods are needed to help patients, providers, hospitals, and health systems plan for and potentially avert adverse outcomes.
OBJECTIVE:Our objective was to understand if machine learning methods with natural language processing of history and physical notes could identify a group of patients at high risk of maternal morbidity on admission for delivery without relying on any additional patient information (eg, demographics and diagnosis codes).
STUDY DESIGN:This was a retrospective study of people admitted for delivery at 2 hospitals (hospitals A and B) in a single healthcare system between July 1, 2016, and June 30, 2020. The primary outcome was severe maternal morbidity, as defined by the Centers for Disease Control and Prevention; furthermore, we examined nontransfusion severe maternal morbidity. Clinician documents designated as history and physical notes were extracted from the electronic health record for processing and analysis. A bag-of-words approach was used for this natural language processing analysis (ie, each history or physical note was converted into a matrix of counts of individual words (or phrases) that occurred within the document). The least absolute shrinkage and selection operator models were used to generate prediction probabilities for severe maternal morbidity and nontransfusion severe maternal morbidity for each note. Model discrimination was assessed via the area under the receiver operating curve. Discrimination was compared between models using the DeLong test. Calibration plots were generated to assess model calibration. Moreover, the natural language processing models with history and physical note texts were compared with validated obstetrical comorbidity risk scores based on diagnosis codes.
RESULTS:There were 13,572 delivery encounters with history and physical notes from hospital A, split between training (Atrain, n=10,250) and testing (Atest, n=3,322) datasets for model derivation and internal validation. There were 23,397 delivery encounters with history and physical notes from hospital B (Bvalid) used for external validation. For the outcome of severe maternal morbidity, the natural language processing model had an area under the receiver operating curve of 0.67 (95% confidence interval, 0.63-0.72) and 0.72 (95% confidence interval, 0.70-0.74) in the Atest and Bvalid datasets, respectively. For the outcome of nontransfusion severe maternal morbidity, the area under the receiver operating curve was 0.72 (95% confidence interval, 0.65-0.80) and 0.76 (95% confidence interval, 0.73-0.79) in the Atest and Bvalid datasets, respectively. The calibration plots demonstrated the bag-of-words model's ability to distinguish a group of individuals at a substantially higher risk of severe maternal morbidity and nontransfusion severe maternal morbidity, notably those in the top deciles of predicted risk. Areas under the receiver operating curve in the natural language processing-based models were similar to those generated using a validated, retrospectively derived, diagnosis code-based comorbidity score.
CONCLUSION:In this practical application of machine learning, we demonstrated the capabilities of natural language processing for the prediction of severe maternal morbidity based on provider documentation inherently generated at the time of admission. This work should serve as a catalyst for providers, hospitals, and electronic health record systems to explore ways that artificial intelligence can be incorporated into clinical practice and evaluated rigorously for their ability to improve health.
MoreTranslated text
AI Read Science
Must-Reading Tree
Example
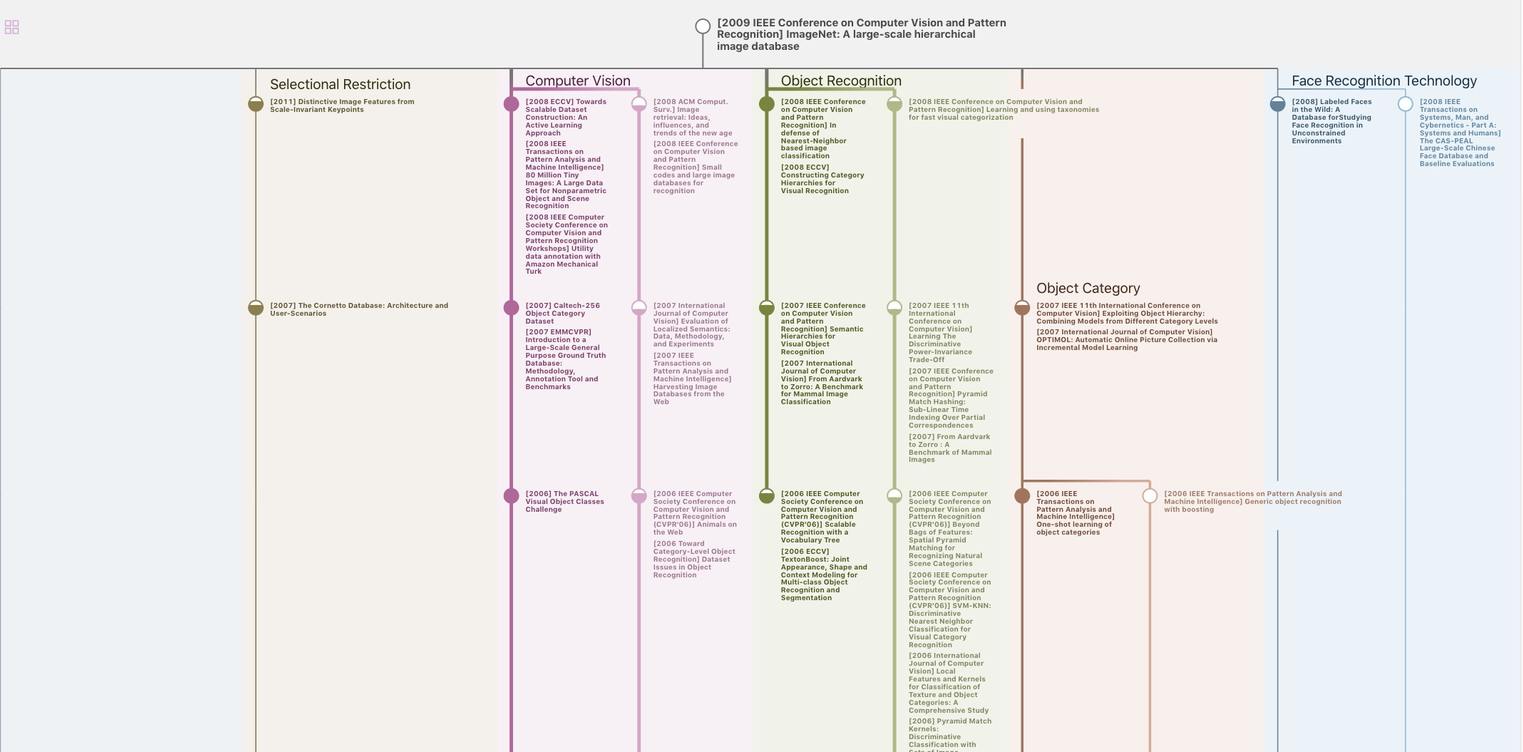
Generate MRT to find the research sequence of this paper
Chat Paper
Summary is being generated by the instructions you defined