Short-Term Load Forecasting At The Local Level Using Smart Meter Data
2015 IEEE EINDHOVEN POWERTECH(2015)
摘要
Recent developments in active distribution networks, and the availability of smart meter data has led to much interest in Short-Term Load Forecasting (STLF) of electrical demand at the local level, e.g. estimation of loads at substations, feeders, and individual users. Local demand profiles are volatile and noisy, making STLF difficult as we move towards lower levels of load aggregation. This paper examines in detail the correlations between demand and the variables which influence it, at various levels of load disaggregation. The analysis investigates the forecasting capability of both linear and non-linear STLF approaches for forecasting local demands, and quantifies the forecast uncertainty for each level of load aggregation. The results demonstrate the limitations of several of the most commonly-used STLF approaches in this context. It is shown that, at the local level, standard STLF models may not be effective, and that simple load models created from historical smart meter data can give similar prediction accuracies. The analysis in the paper is carried out using two large smart meter data sets recorded at distribution networks in Denmark and in Ireland.
更多查看译文
关键词
Demand forecasting, smart grids, load management, forecast uncertainty, power demand
AI 理解论文
溯源树
样例
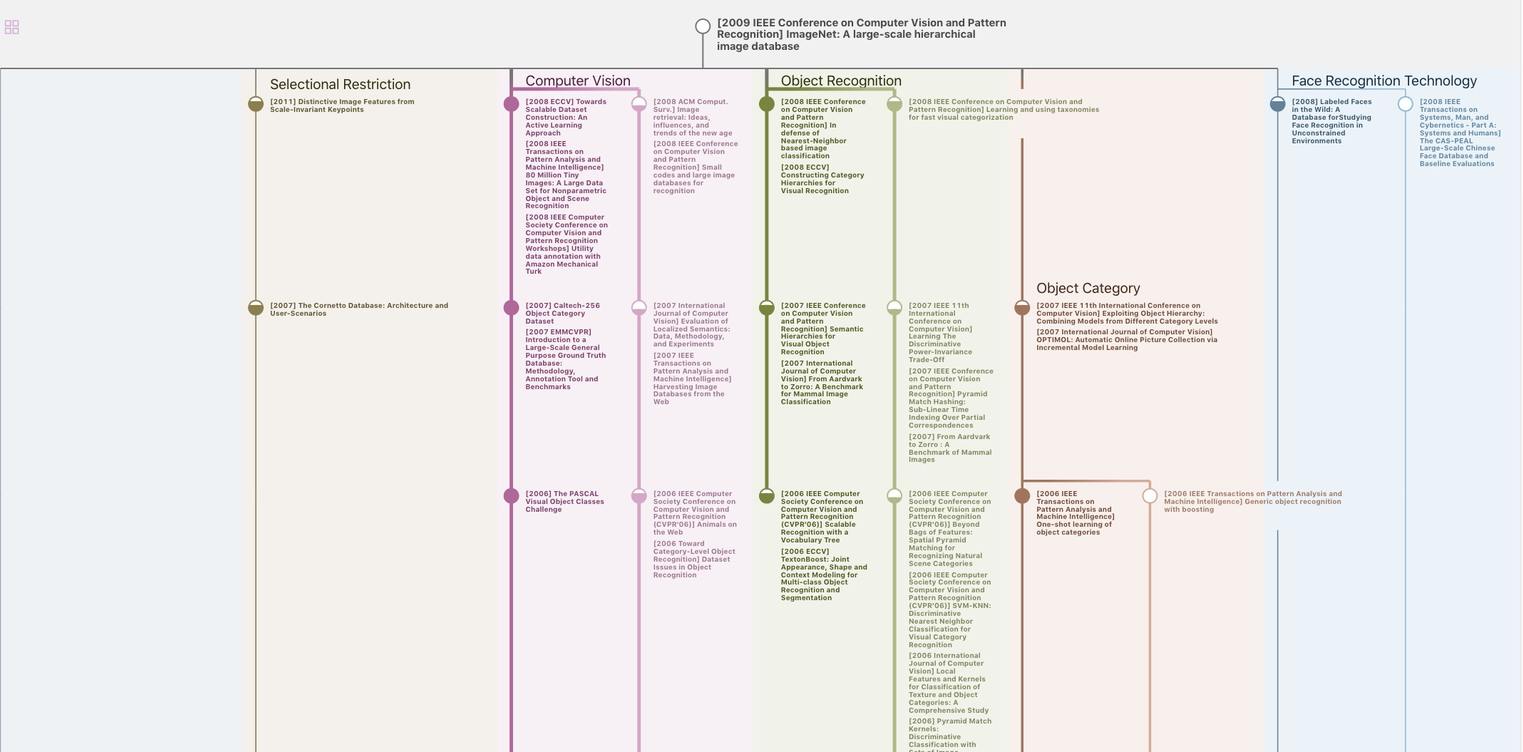
生成溯源树,研究论文发展脉络
Chat Paper
正在生成论文摘要