A new CAD approach for improving efficacy of cancer screening
Proceedings of SPIE(2015)
摘要
Since performance and clinical utility of current computer-aided detection (CAD) schemes of detecting and classifying soft tissue lesions (e.g., breast masses and lung nodules) is not satisfactory, many researchers in CAD field call for new CAD research ideas and approaches. The purpose of presenting this opinion paper is to share our vision and stimulate more discussions of how to overcome or compensate the limitation of current lesion-detection based CAD schemes in the CAD research community. Since based on our observation that analyzing global image information plays an important role in radiologists' decision making, we hypothesized that using the targeted quantitative image features computed from global images could also provide highly discriminatory power, which are supplementary to the lesion-based information. To test our hypothesis, we recently performed a number of independent studies. Based on our published preliminary study results, we demonstrated that global mammographic image features and background parenchymal enhancement of breast MR images carried useful information to (1) predict near-term breast cancer risk based on negative screening mammograms, (2) distinguish between true-and false-positive recalls in mammography screening examinations, and (3) classify between malignant and benign breast MR examinations. The global case-based CAD scheme only warns a risk level of the cases without cueing a large number of false-positive lesions. It can also be applied to guide lesion-based CAD cueing to reduce false-positives but enhance clinically relevant true-positive cueing. However, before such a new CAD approach is clinically acceptable, more work is needed to optimize not only the scheme performance but also how to integrate with lesion-based CAD schemes in the clinical practice.
更多查看译文
关键词
Computer-aided detection (CAD),Breast cancer screening,Cancer risk prediction,Reduction of false-positive recalls
AI 理解论文
溯源树
样例
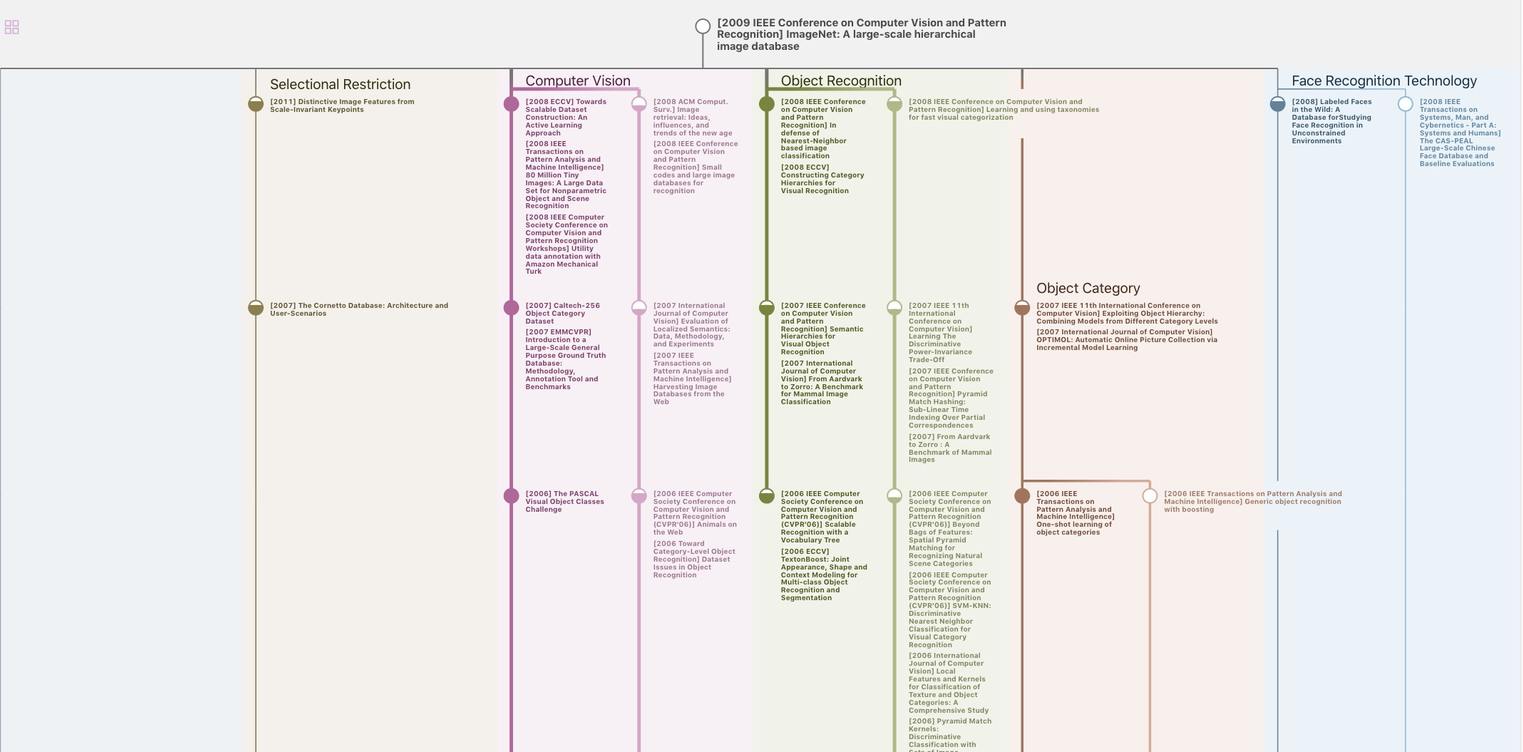
生成溯源树,研究论文发展脉络
Chat Paper
正在生成论文摘要