Multi-label semantic scene classification
msra(2003)
摘要
Abstract In classic pattern recognition problems, classes are mutually exclusive by denition. Classication errors occur when the classes overlap in the feature space. We examine a dieren t situation, occurring when the classes are, by denition, not mutually exclusive. Such problems arise in semantic scene and document classication and in medical diagnosis. We present a framework to handle such problems and apply it to the problem of semantic scene classication, where a natural scene may contain multiple objects such that the scene can be described by multiple class labels (e.g., a eld scene with a mountain in the background). Such a problem poses challenges to the classic pattern recognition paradigm and demands,a dieren t treatment. We discuss approaches for training and testing in this scenario and introduce new metrics for evaluating individual examples, class recall and precision, and overall accuracy. Experiments show that our methods are suitable for scene classication; furthermore, our work appears to generalize to other classication problems of the same nature. Keywords: pattern recognition, machine learning, image understanding, semantic scene classication, multi-label classication, multi-label training, multi-label evaluation, image organization, cross-training, Jaccard similarity
更多查看译文
关键词
cross-training,pattern recognition,image organization,multi-label evaluation,machine learning,multi-label classication,image understanding,jaccard similarity,semantic scene classication,multi-label training,medical diagnosis,feature space
AI 理解论文
溯源树
样例
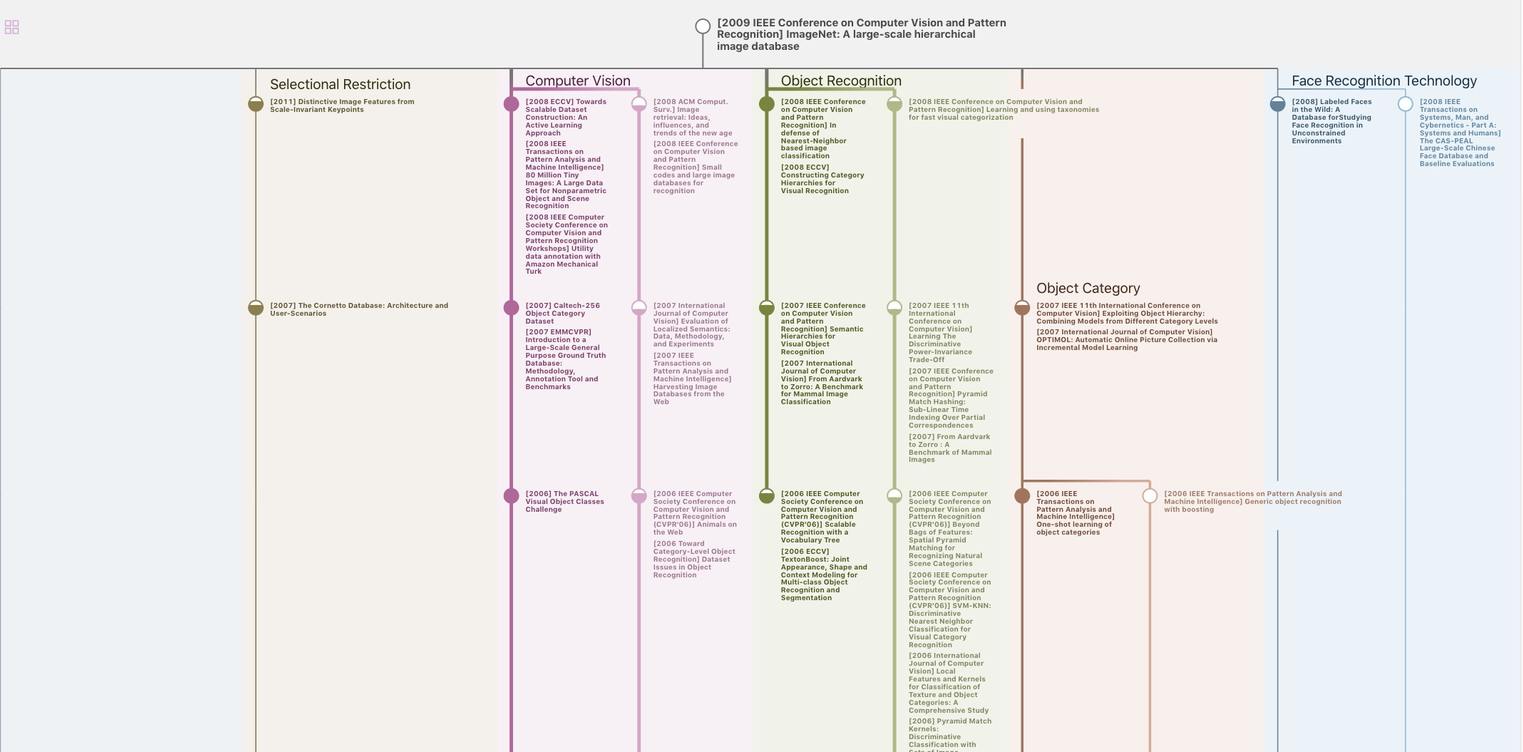
生成溯源树,研究论文发展脉络
Chat Paper
正在生成论文摘要