Model-based outliers treatment in image-based systems biology: filtering vs. noise model
european conference on mathematical and theoretical biology(2014)
摘要
Background: Biological tissues are dynamical systems with a complex spatial organization. To study these systems, imaging methods are used and constantly improved to allow for a quantitative assessment. Image-based modeling aims at systematically using this imaging data to create spatio-temporal models of the underlying biological processes and to understand their working modes. It is however not clear how imaging artifacts, e.g. outliers, can be treated in the context of image-based modeling. Methods: We illustrate the process of data-driven tissue-scale modeling imaging data containing outliers using the example of gradient formation for dendritic cell guidance towards the lymphoid vessels. The elementary steps are model development, parameter estimation and uncertainty analysis. To account for outliers, we developed a model-based approach relying on a reformulated likelihood function for the parameter estimation. This approach has been compared to common image filtering methods using synthetic data. We found that the model-based approach outperforms available methods and significantly reduces bias and variance in the estimated model parameters. Results and Conclusion : We applied the developed method to staining images of the chemokine CCL21, which is responsible for dendritic cell guidance towards the lymphoid vessel. The images contain outliers created by migrating fibroblasts and our novel method could substantially reduce the bias in the estimated parameters. The developed method is widely applicable to parameter estimation problems from images and does, in contrast to filtering methods, not require the fine tuning of parameters.
更多查看译文
关键词
image analysis,parameter estimation
AI 理解论文
溯源树
样例
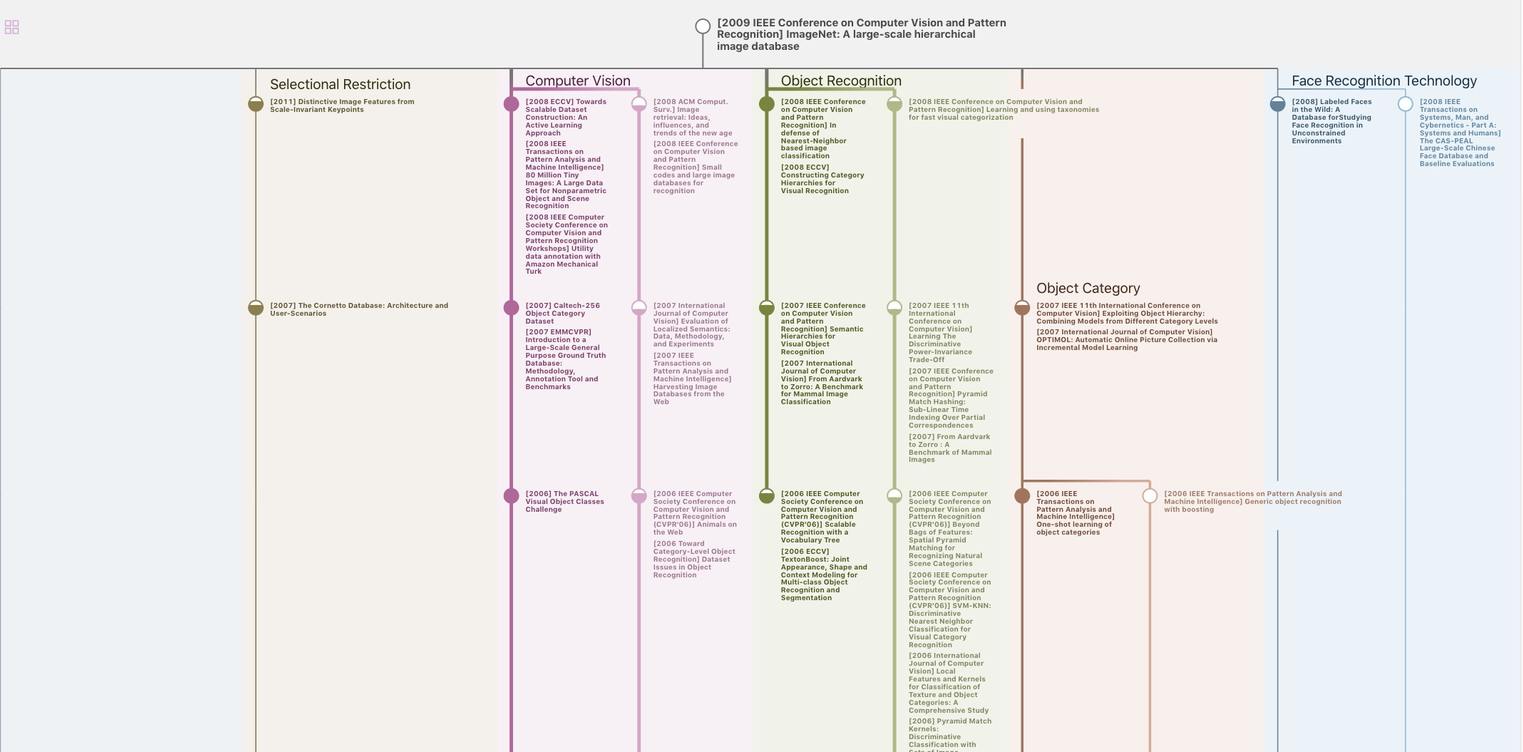
生成溯源树,研究论文发展脉络
Chat Paper
正在生成论文摘要