Granular self-organizing map (grSOM) neural network for industrial quality control
mag(2005)
摘要
Industrial product quality is frequently assessed using up to second-order statistics of populations of measurements. Lately, a fuzzy interval number (FIN) was used for representing a whole population of samples. It turns out that a FIN can asymptotically capture statistics of all orders. The space F of FINs, including both conventional (fuzzy) numbers and conventional intervals, is studied here. A FIN is interpreted as a (linguistic) information granule that can capture industrial ambiguity. Based on generalized interval analysis it is shown rigorously that F is a metric mathematical lattice; moreover it is shown that F a cone in a linear space. An enhanced extension of Kohonen's Self-Organizing Map (KSOM), namely granular SOM or grSOM for short, is presented in FN for inducing a distribution of FINs from populations of measurements. The grSOM produces descriptive decision-making knowledge (i.e. rules) from the training data by expert attaching labels to induced n-tuples of FINs. Generalization is feasible beyond rule support. A positive valuation function, computable genetically, can introduce tunable nonlinearities. Preliminary results are demonstrated regarding industrial fertilizer quality assessment. Fuzzy-mathematical-morphology-based image processing techniques, which combine binary thresholding and object recognition, are used to automatically measure the geometry of fertilizer granules. Additional measurements are also considered. The far-reaching practical potential of the proposed techniques is discussed.
更多查看译文
关键词
quality control,image processing,linear space,industrial production,object recognition,genetics,fuzzy number,neural networks,interval analysis,neural network,vector spaces
AI 理解论文
溯源树
样例
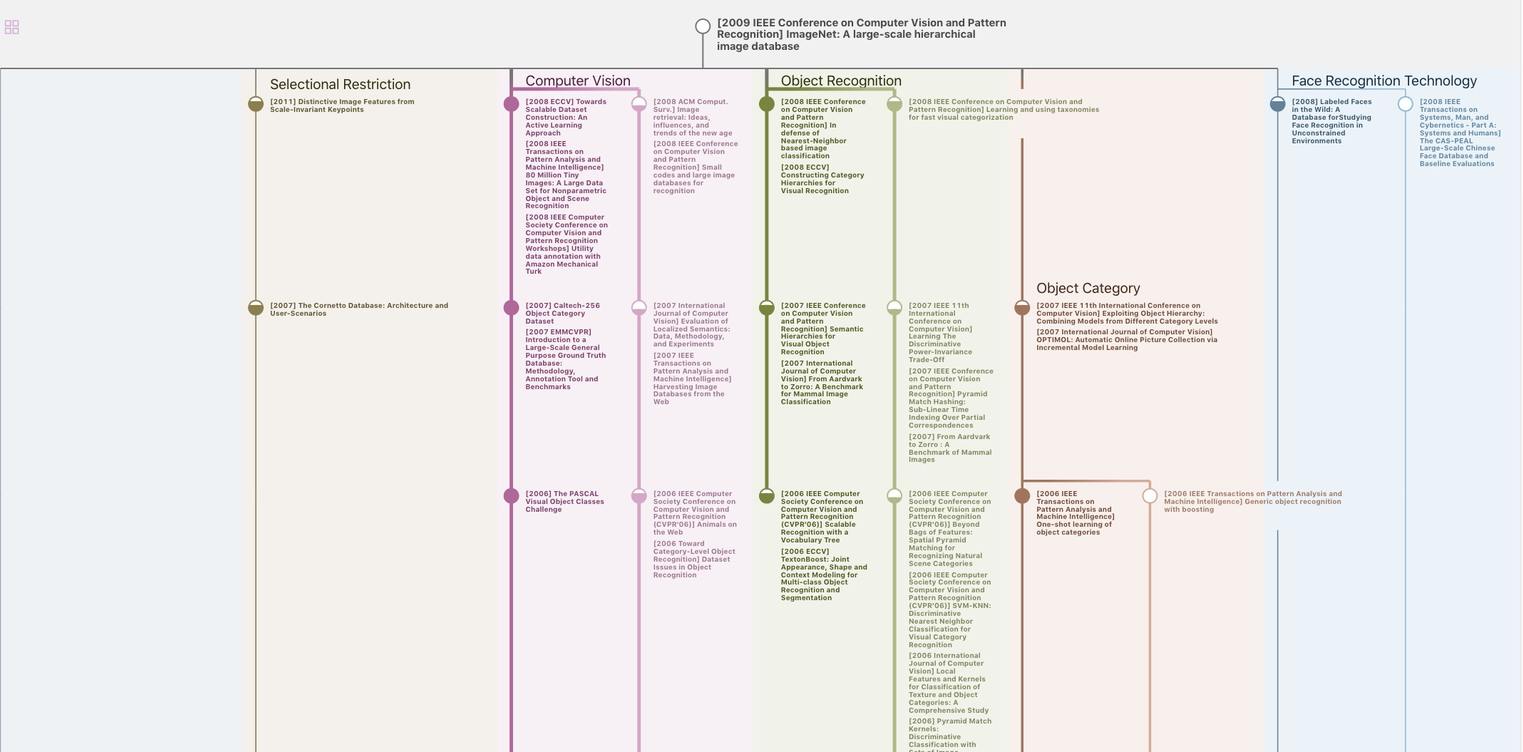
生成溯源树,研究论文发展脉络
Chat Paper
正在生成论文摘要