An Initial Study on Climate Change Fingerprinting Using The Reflected Solar Spectra
JOURNAL OF CLIMATE(2016)
摘要
Attribution of averaged spectral variation over large spatial and temporal scales to different climate variables is central to climate change fingerprinting. Using 10 years of satellite data for simulation, the authors generate a group of observation-based spectral fingerprints and a time series of monthly mean reflectance spectra over the ocean in five large latitude regions and globally. Next, these fingerprints and the interannual variation spectra are used to retrieve the interannual changes in the relevant climate variables to test the concept of using the spectral fingerprinting approach for climate change attribution. Comparing the fingerprinting retrieval of climate variable change to the actual underlying variable change, the RMS differences between the two are less than twice as large as the monthly variability for all variables in all regions. Instances where larger errors are observed correspond to those variables with large nonlinear radiative response, such as the cloud optical depth and the ice particle size. Using the linear fingerprinting approach and accounting for the nonlinear radiative error in fingerprints results in significantly higher retrieval accuracy; the RMS errors are reduced to less than the monthly variability for nearly all variables, indicating the profound impact of the nonlinear error on fingerprinting retrieval. Another important finding is that if the cloud fraction is known a priori, the retrieval accuracy in cloud optical depth would be improved substantially. Moreover, a better retrieval for the water vapor amount and aerosol optical depth can be achieved from the clear-sky data only. The test results demonstrate that climate change fingerprinting based on reflected solar benchmark spectra is possible.
更多查看译文
关键词
Variability,Cloud radiative effects,Interannual variability,Variational analysis,Radiative transfer,Mathematical and statistical techniques,Physical Meteorology and Climatology
AI 理解论文
溯源树
样例
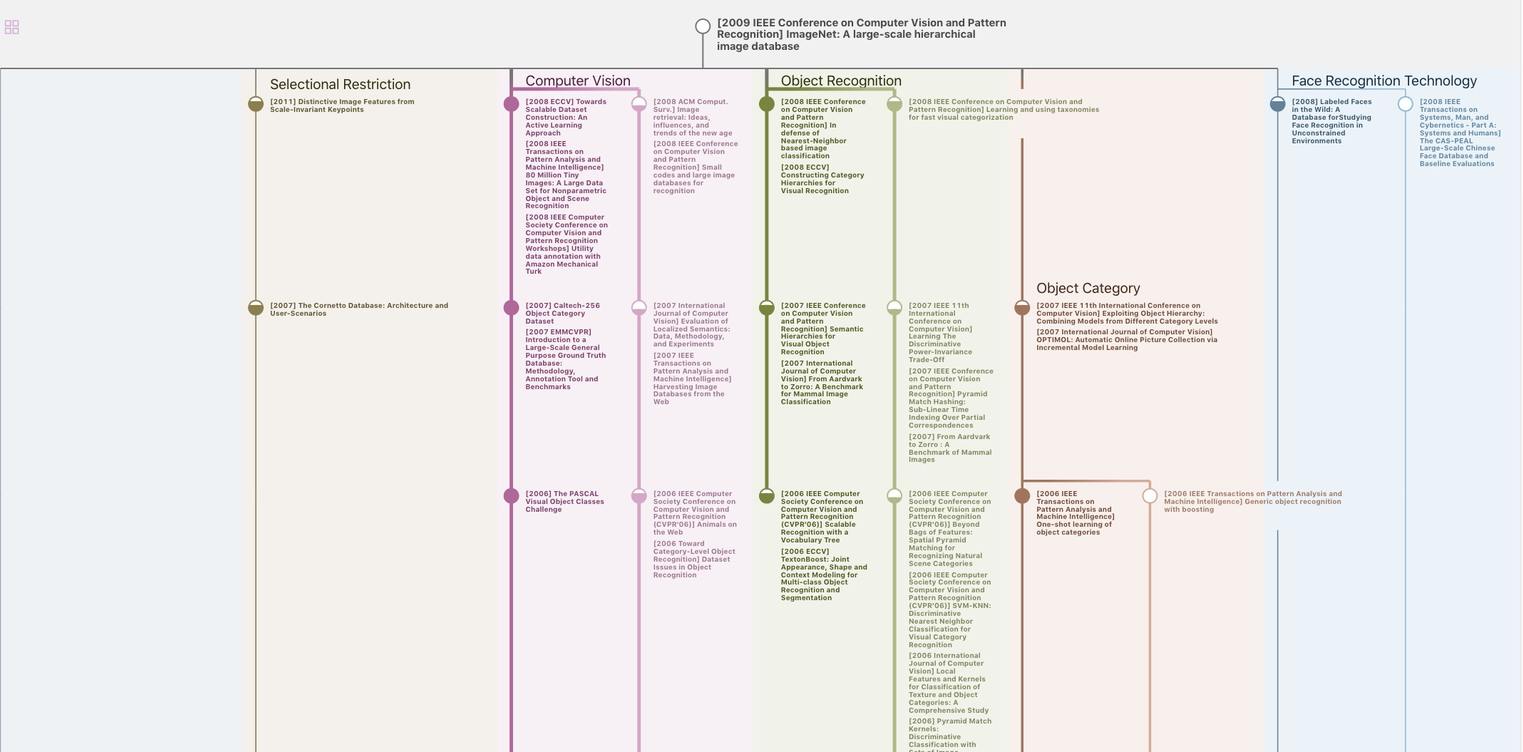
生成溯源树,研究论文发展脉络
Chat Paper
正在生成论文摘要