Intrusion detection and Big Heterogeneous Data: a Survey
J. Big Data(2015)
摘要
Intrusion Detection has been heavily studied in both industry and academia, but cybersecurity analysts still desire much more alert accuracy and overall threat analysis in order to secure their systems within cyberspace. Improvements to Intrusion Detection could be achieved by embracing a more comprehensive approach in monitoring security events from many different heterogeneous sources. Correlating security events from heterogeneous sources can grant a more holistic view and greater situational awareness of cyber threats. One problem with this approach is that currently, even a single event source (e.g., network traffic) can experience Big Data challenges when considered alone. Attempts to use more heterogeneous data sources pose an even greater Big Data challenge. Big Data technologies for Intrusion Detection can help solve these Big Heterogeneous Data challenges. In this paper, we review the scope of works considering the problem of heterogeneous data and in particular Big Heterogeneous Data. We discuss the specific issues of Data Fusion, Heterogeneous Intrusion Detection Architectures, and Security Information and Event Management (SIEM) systems, as well as presenting areas where more research opportunities exist. Overall, both cyber threat analysis and cyber intelligence could be enhanced by correlating security events across many diverse heterogeneous sources.
更多查看译文
关键词
Intrusion detection, Big data, Security, IDS, SIEM, Data fusion, Heterogeneous, Hadoop, Cloud, Feature selection, Situational awareness, Big Heterogeneous Data
AI 理解论文
溯源树
样例
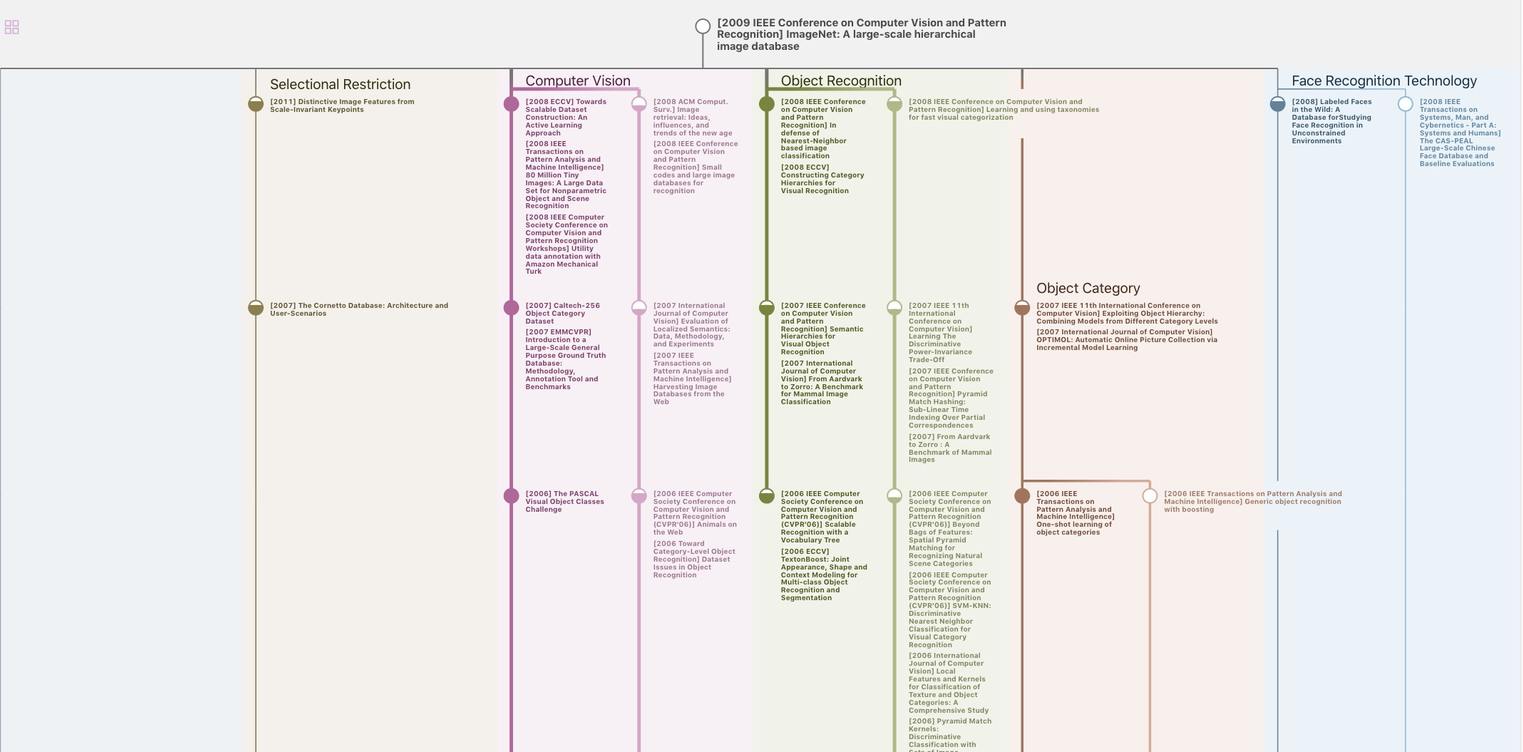
生成溯源树,研究论文发展脉络
Chat Paper
正在生成论文摘要