Hybrid Imitative Planning with Geometric and Predictive Costs in Off-road Environments
IEEE International Conference on Robotics and Automation(2022)
摘要
Geometric methods for solving open-world off-road navigation tasks, by learning occupancy and metric maps, provide good generalization but can be brittle in outdoor environments that violate their assumptions (e.g., tall grass). Learning-based methods can directly learn collision-free behavior from raw observations, but are difficult to integrate with standard geometry-based pipelines. This creates an unfortunate conflict – either use learning and lose out on well-understood geometric navigational components, or do not use it, in favor of extensively hand-tuned geometry-based cost maps. In this work, we reject this dichotomy by designing the learning and non-learning-based components in a way such that they can be effectively combined in a self-supervised manner. Both components contribute to a planning criterion: the learned component contributes predicted traversability as rewards, while the geometric component contributes obstacle cost information. We instantiate and comparatively evaluate our system in both in-distribution and out-of-distribution environments, showing that this approach inherits complementary gains from the learned and geometric components and significantly outperforms either of them.
更多查看译文
关键词
outdoor environments,tall grass,learning-based methods,collision-free behavior,raw observations,standard geometry-based pipelines,planning criterion,obstacle cost information,out-of-distribution environments,geometric components,hybrid imitative planning,off-road environments,off-road navigation tasks,metric maps,predictive costs,component contribute learning,hand-tuned geometry-based cost maps,geometric navigational components
AI 理解论文
溯源树
样例
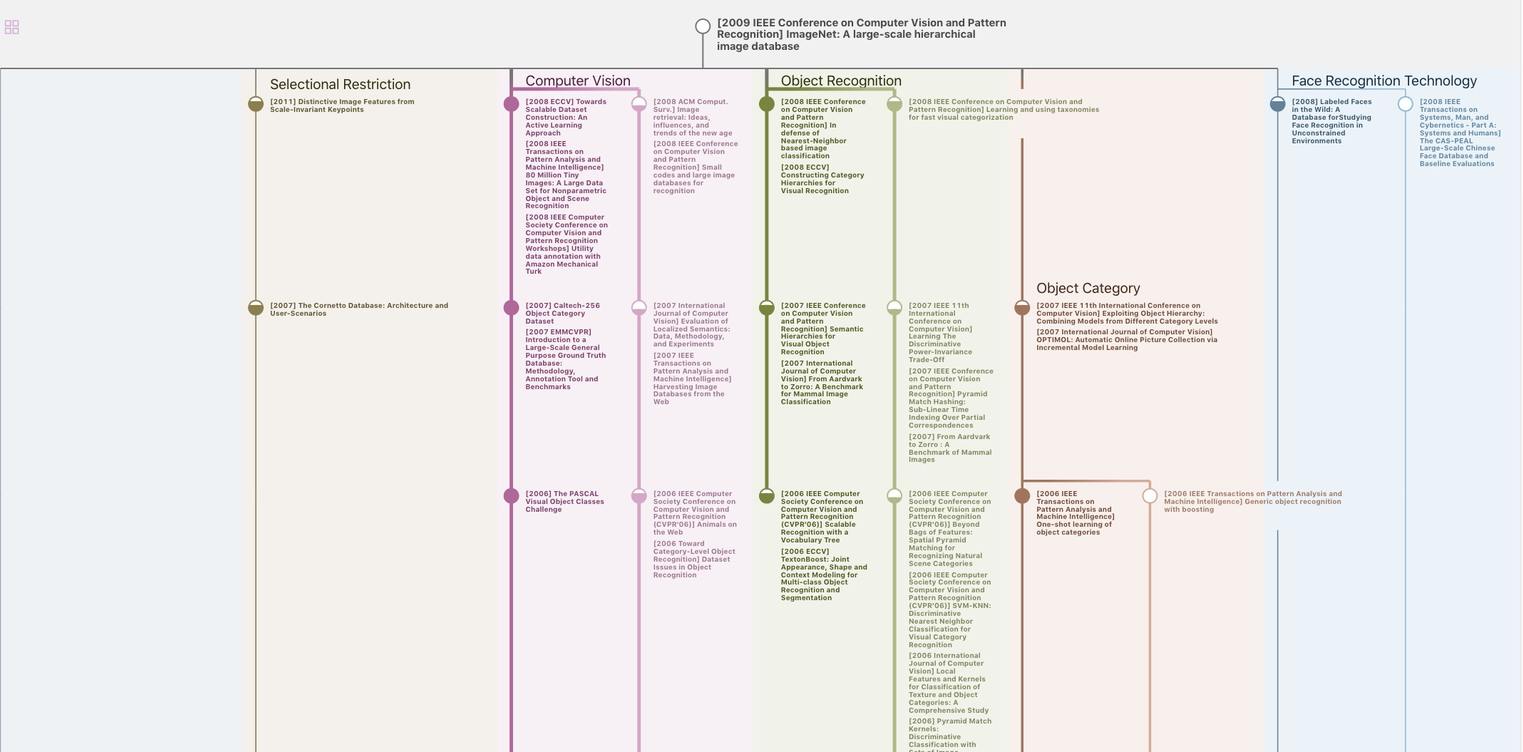
生成溯源树,研究论文发展脉络
Chat Paper
正在生成论文摘要