Opponent Modeling By Expectation-Maximization And Sequence Prediction In Simplified Poker
IEEE TRANSACTIONS ON COMPUTATIONAL INTELLIGENCE AND AI IN GAMES(2017)
摘要
We consider the problem of learning an effective strategy online in a hidden information game against an opponent with a changing strategy. We want to model and exploit the opponent and make three proposals to do this; first, to infer its hidden information using an expectation-maximization (EM) algorithm; second, to predict its actions using a sequence prediction method; and third, to simulate games between our agent and our opponent model in-between games against the opponent. Our approach does not require knowledge outside the rules of the game, and does not assume that the opponent's strategy is stationary. Experiments in simplified poker games show that it increases the average payoff per game of a state-of-the-art no-regret learning algorithm.
更多查看译文
关键词
Counterfactual regret minimization,expectation-maximization (EM) algorithms,learning in games,opponent modeling,sequence prediction,simplified poker
AI 理解论文
溯源树
样例
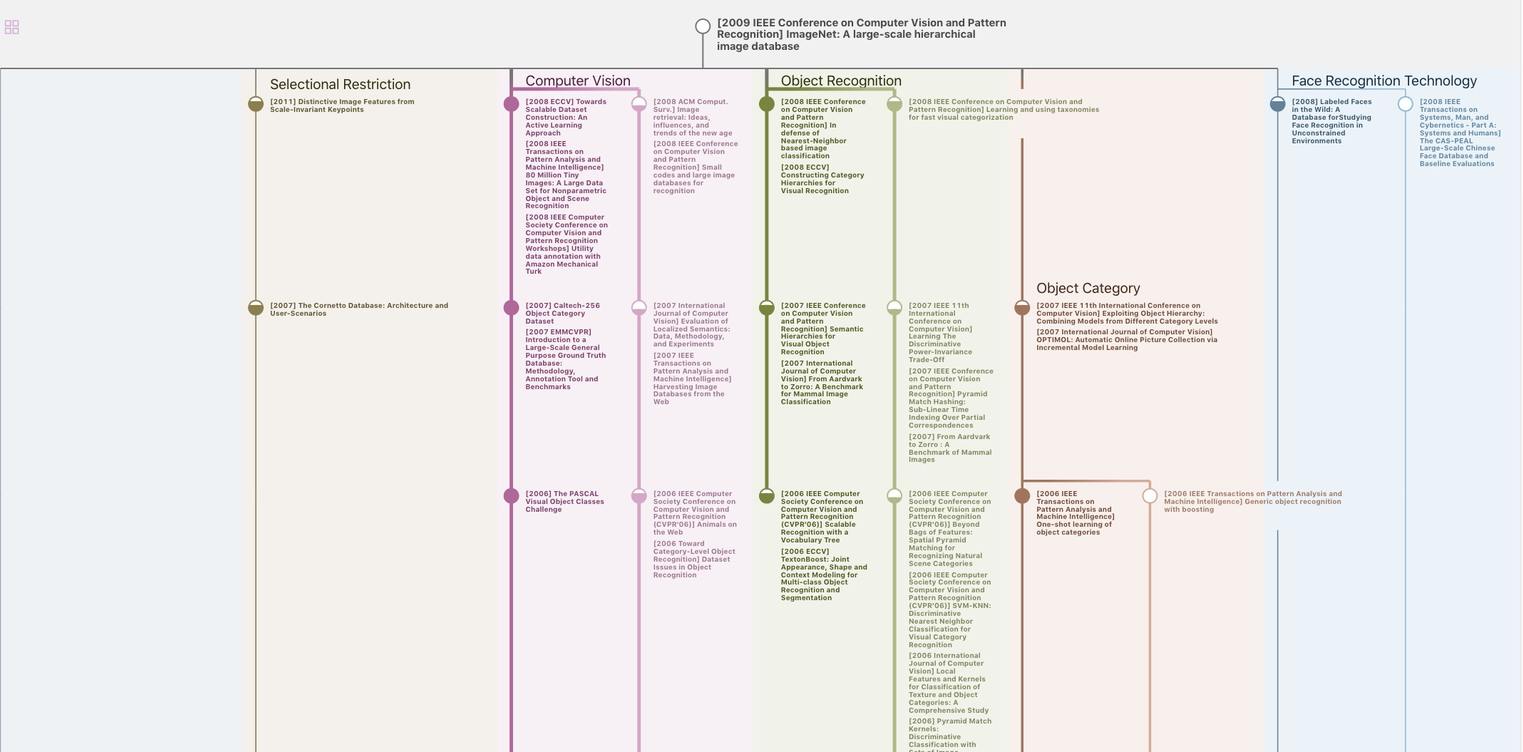
生成溯源树,研究论文发展脉络
Chat Paper
正在生成论文摘要