Ultra-deep proteomics by Thin-diaPASEF with a 60-cm long column system
crossref(2024)
Abstract
Recent advances have allowed for the detection of 10,000 proteins from cultured human cell samples, such as HeLa and HEK293T cells in a single-shot proteome analysis. However, deeper analysis remains challenging. Therefore, in this study, we aimed to perform a deep proteomic single-shot analysis using timsTOF HT. To achieve deep proteomics, we developed Thin-diaPASEF, a parallel accumulation-serial fragmentation (PASEF) technology featuring a thinly divided m/z axis only in regions of high ion density. Furthermore, using a 60-cm long C18 column with a particle size of 1.7 µm, an average of 11,698, 11,615 and 11,019 unique proteins were successfully detected from 500 ng of HEK293T, HeLa and K562 cell digests, respectively, with a 100 min active gradient. The same system was used to analyze Lycopersicon esculentum lectin (LEL) enriched plasma and serum. The LEL method identified an average of 8,613 and 4,078 unique proteins, in plasma and serum, respectively. Our ultra-deep proteomic analysis system will be helpful for the in-depth comparison of proteins in medical and biological research because it enables the analysis of highly proteome coverage in a single-shot.
### Competing Interest Statement
The authors have declared no competing interest.
Mass spectrometry proteomic data analysis was deposited in the ProteomeXchange Consortium via the jPOST partner repository ([27][1]) with the dataset identifiers PXDxxxxx for ProteomeXchange and JPSTxxxxx for jPOST.
* ACN
: acetonitrile
DIA
: data-independent acquisition
DMNG
: decyl maltose neopentyl glycol
diaPASEF
: DIA method using parallel accumulation-serial fragmentation technology
FDA
: Food and Drug Administration
LC
: Liquid chromatography
LEL
: Lycopersicon esculentum lectin
PASEF
: parallel accumulation-serial fragmentation
SPD
: samples per day
TFA
: trifluoroacetic acid
TBS
: Tris buffered saline
[1]: #ref-27
MoreTranslated text
AI Read Science
Must-Reading Tree
Example
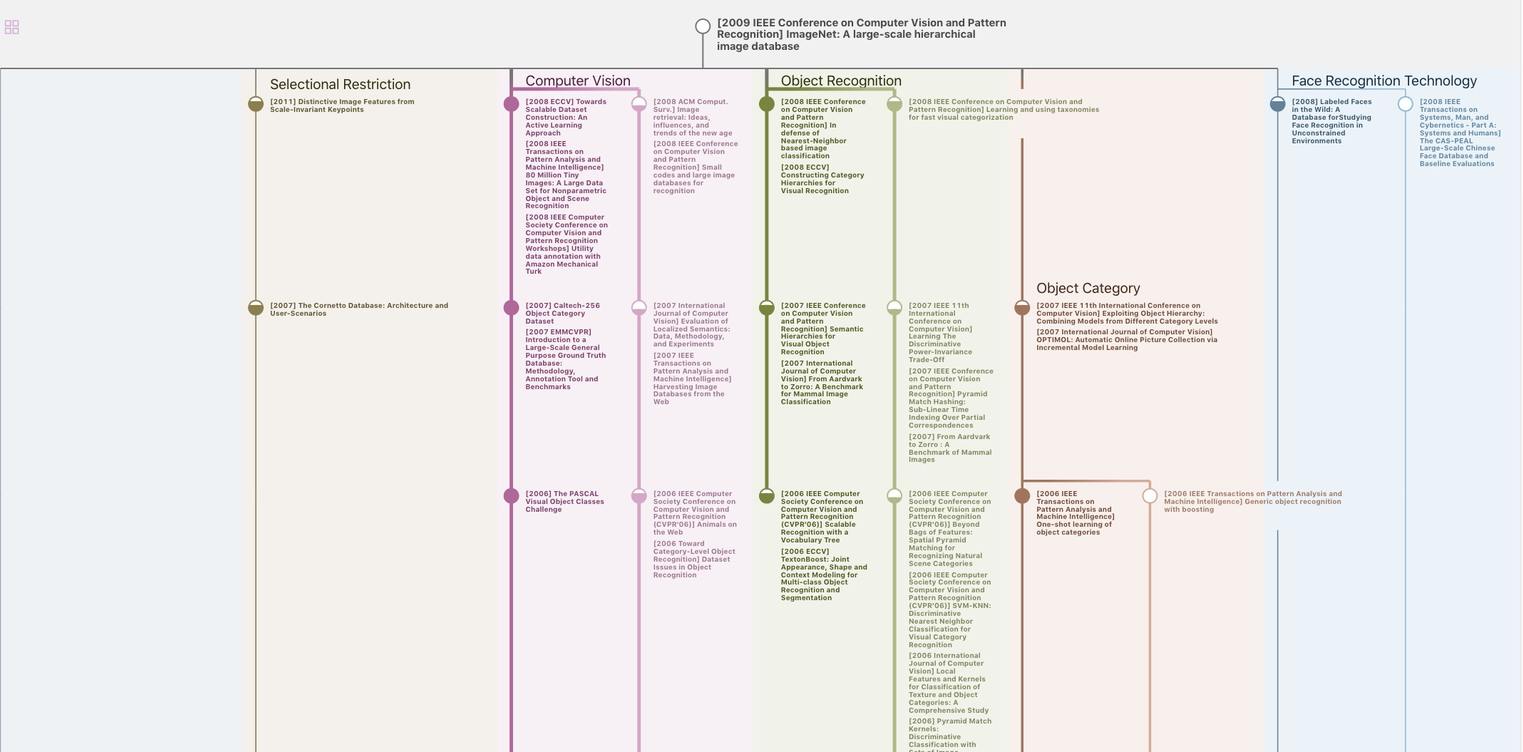
Generate MRT to find the research sequence of this paper
Chat Paper
Summary is being generated by the instructions you defined