Hierarchical and variational Bayesian approaches to least-squares migration
Seg Technical Program Expanded Abstracts(2014)
摘要
PreviousNext No AccessSEG Technical Program Expanded Abstracts 2014Hierarchical and variational Bayesian approaches to least-squares migrationAuthors: S. Ahmad Zamanian*William L. RodiMichael C. FehlerJonathan A. KaneS. Ahmad Zamanian*MITSearch for more papers by this author, William L. RodiMITSearch for more papers by this author, Michael C. FehlerMITSearch for more papers by this author, and Jonathan A. KaneShell International E & P, Inc.Search for more papers by this authorhttps://doi.org/10.1190/segam2014-0954.1 SectionsSupplemental MaterialAboutPDF/ePub ToolsAdd to favoritesDownload CitationsTrack CitationsPermissions ShareFacebookTwitterLinked InRedditEmail Abstract Within the context of least-squares migration (LSM), we describe a methodology for using surface seismic data to infer the spatial smoothness structure of the subsurface in addition to the subsurface reflectivity model (or seismic image). In LSM (and other geophysical inverse problems), smoothness assumptions about the subsurface are commonly invoked to regularize the inversion procedure. The regularization serves to mitigate the effects of poor resolution and noise in the data and improve the quality of the inferred subsurface parameters. Viewing the problem from the perspective of Bayesian inference, a priori assumptions about the probabilistic structure of the model parameters impose this regularization. We generalize this by considering a hierarchical Bayesian formulation of the LSM problem in which parameters controlling the regularization, in addition to the reflectivity model itself, are treated as problem unknowns. Hence, rather than fixing the regularization parameters prior to inverting for the image, we allow the data to dictate where and how strongly to smooth the image. In order to construct our prior model of the subsurface and regularization parameters, we define an undirected graphical model (or Markov random field) on the subsurface model in which vertices of the graph represent reflectivity values, and edge strengths, defined on the edges of the graph, capture the degree of correlation (or lack thereof) between the vertices. Inferring the edge strengths from the data should produce an estimate of the image which preserves sharpness at the true discontinuities in the image while still allowing for smoothing of noise where appropriate. We solve the inference problem using a powerful approximation methodology known as variational Bayes, which produces marginal posterior distributions for the image and regularization parameters. Our inference framework is verified on the Marmousi model, where the hierarchical Bayesian imaging results significantly outperform standard LSM imaging. Keywords: algorithm, estimation, least squares, migration, statisticalPermalink: https://doi.org/10.1190/segam2014-0954.1FiguresReferencesRelatedDetails SEG Technical Program Expanded Abstracts 2014ISSN (print):1052-3812 ISSN (online):1949-4645Copyright: 2014 Pages: 5183 publication data© 2014 Published in electronic format with permission by the Society of Exploration GeophysicistsPublisher:Society of Exploration Geophysicists HistoryPublished: 05 Aug 2014 CITATION INFORMATION S. Ahmad Zamanian*, William L. Rodi, Michael C. Fehler, and Jonathan A. Kane, (2014), "Hierarchical and variational Bayesian approaches to least-squares migration," SEG Technical Program Expanded Abstracts : 3731-3736. https://doi.org/10.1190/segam2014-0954.1 Plain-Language Summary Keywordsalgorithmestimationleast squaresmigrationstatisticalPDF DownloadLoading ...
更多查看译文
关键词
variational bayesian approaches,migration,least-squares
AI 理解论文
溯源树
样例
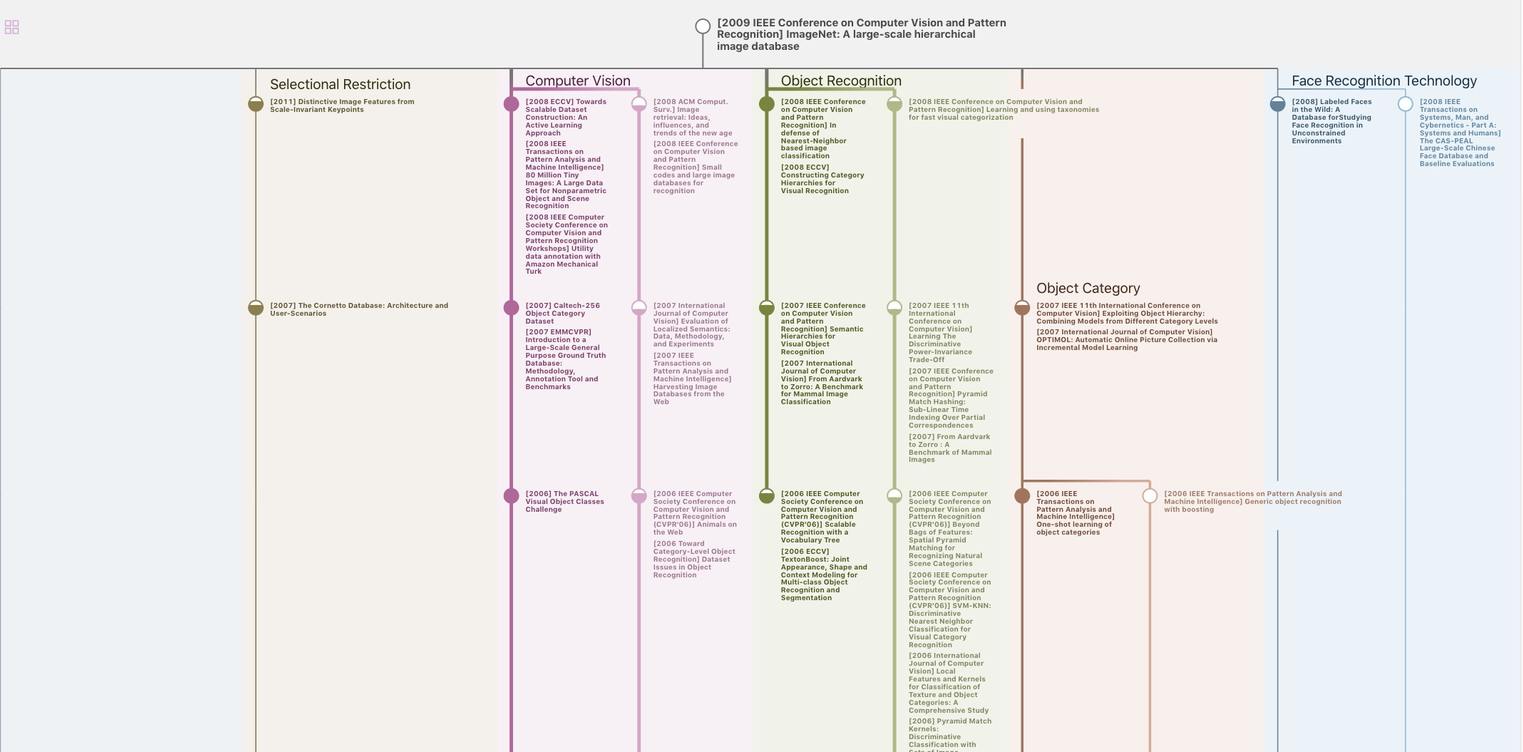
生成溯源树,研究论文发展脉络
Chat Paper
正在生成论文摘要