Stochastic inversion of 2D magnetotelluric data using pixel-based parameterization
Seg Technical Program Expanded Abstracts(2014)
Abstract
We develop a Bayesian model to invert 2D magnetotelluric (MT) data using a pixel-based parameterization, and apply it to an active geothermal field. Although this parameterization is more flexible in representing subsurface resistivity structure than sharp boundary models, it may cause problems for Markov chain Monte Carlo (MCMC) based Bayesian methods because it significantly increases the total number of unknowns, most of which are spatially correlated. The key to success for stochastic approaches is to employ a suitable prior model to represent the spatial dependence and to develop efficient sampling strategies for exploring the joint posterior distribution. In this study, we use a Gaussian Markov random field with an unknown controlling parameter to describe the spatial dependence of the resistivity and use gradient-based algorithms to draw MCMC samples. To shorten the burn-in time, we run multiple chains from the local optimal solution found by Occam inversion methods. The case study results show the developed stochastic method and the sampling strategies are effective for 2D MT inversion. Compared to deterministic inversion methods, such as 2D Occam inversion or 3D Gauss-Newton methods, the stochastic method provides significantly more uncertainty information on the features of interest.
MoreTranslated text
Key words
Magnetotelluric Imaging,Inverse Modeling,Geophysical Inversion,Spectral Induced Polarization,Transport Modeling
AI Read Science
Must-Reading Tree
Example
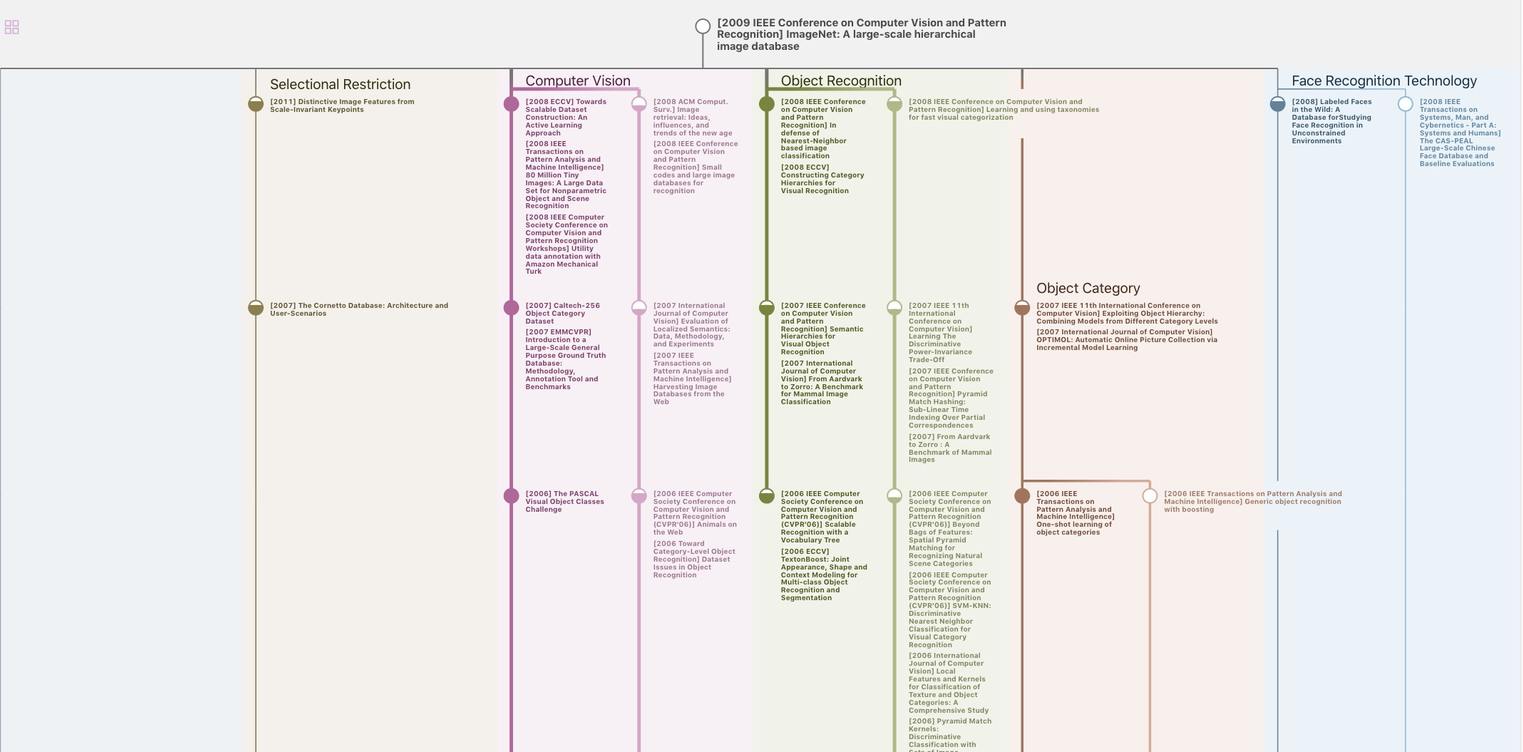
Generate MRT to find the research sequence of this paper
Chat Paper
Summary is being generated by the instructions you defined