Kernel regression estimation for incomplete data with applications
Statistical Papers(2015)
摘要
Methods are proposed to construct kernel estimators of a regression function in the presence of incomplete data. Furthermore, exponential upper bounds are derived on the performance of the L_p norms of the proposed estimators, which can then be used to establish various strong convergence results. The presence of incomplete data points are handled by a Horvitz–Thompson-type inverse weighting approach, where the unknown selection probabilities are estimated by both kernel regression and least-squares methods. As an immediate application of these results, the problem of nonparametric classification with partially observed data will be studied.
更多查看译文
关键词
Regular kernels, Incomplete data, Convergence, Regression, Classification, 62G08
AI 理解论文
溯源树
样例
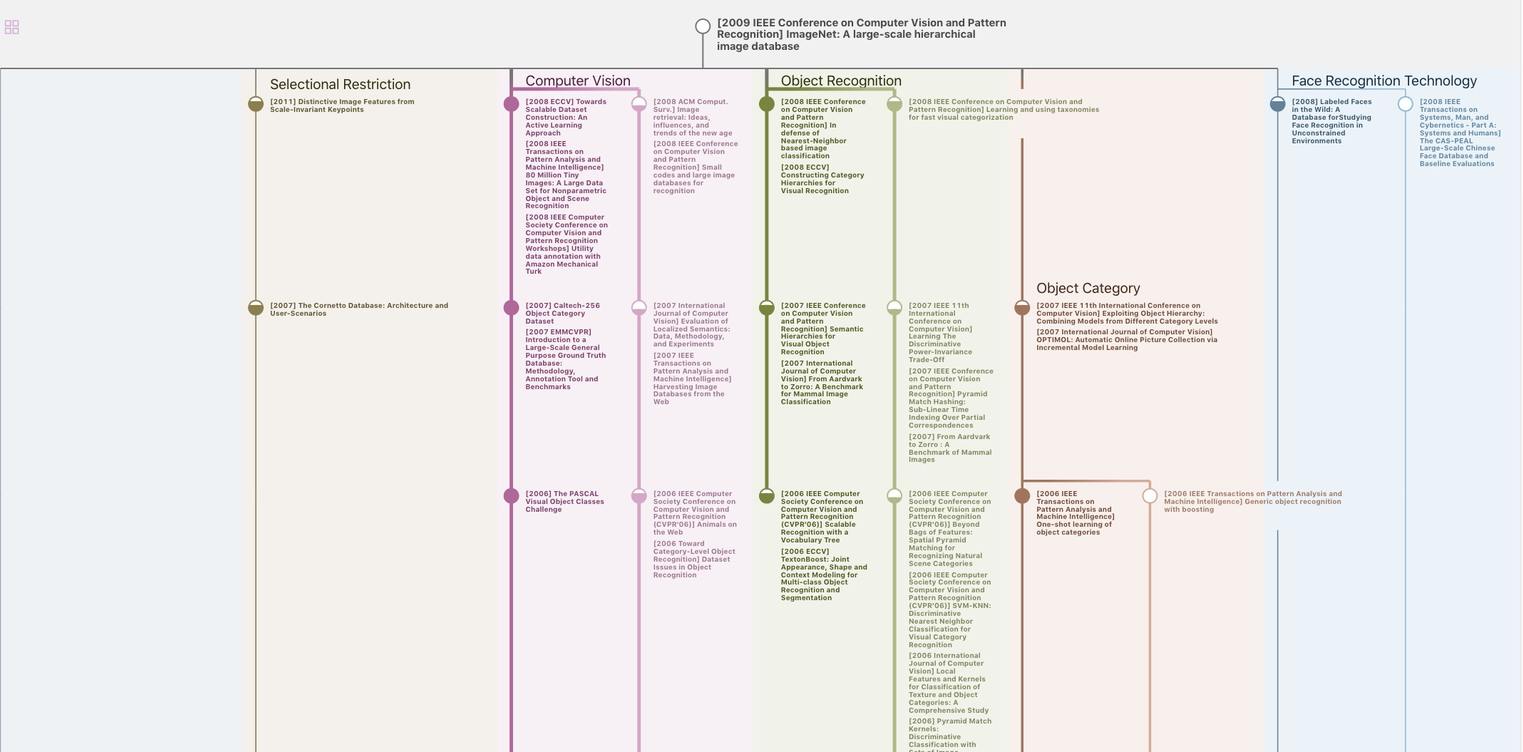
生成溯源树,研究论文发展脉络
Chat Paper
正在生成论文摘要