Soft sensor based on Gaussian process regression and its application in erythromycin fermentation process
CHEMICAL INDUSTRY & CHEMICAL ENGINEERING QUARTERLY(2016)
摘要
Erythromycin fermentation is a typical microbial fermentation process. Soft sensors can be used to estimate the biomass of Erythromycin fermentation process due to their relative low cost, simple development, and ability to predict difficult-to-measure variables. However, traditional soft sensors, e.g. artificial neural network (ANN) soft sensors, support vector machine (SVM) soft sensors, etc., cannot represent the uncertainty (measurement precision) of outputs, which results in difficulties in practice. Gaussian process regression (GPR) provides a novel framework to solve regression problems. The output uncertainty of a GPR model follows Gaussian distribution, expressed in terms of mean and variance. The mean represents the predicted output. The variance can be viewed as the measure of confidence in the predicted output that distinguishes the GPR from NN and SVM soft sensor models. We propose a systematic approach based on GPR and principal component analysis (PCA) to establish a soft sensor to estimate biomass of erythromycin fermentation process. Simulations on industrial data from an erythromycin fermentation process show the proposed GPR soft sensor has high performance of modeling the uncertainty of estimates.
更多查看译文
关键词
fermentation process,soft sensor,uncertainly,Gaussian process regression,principle component analysis
AI 理解论文
溯源树
样例
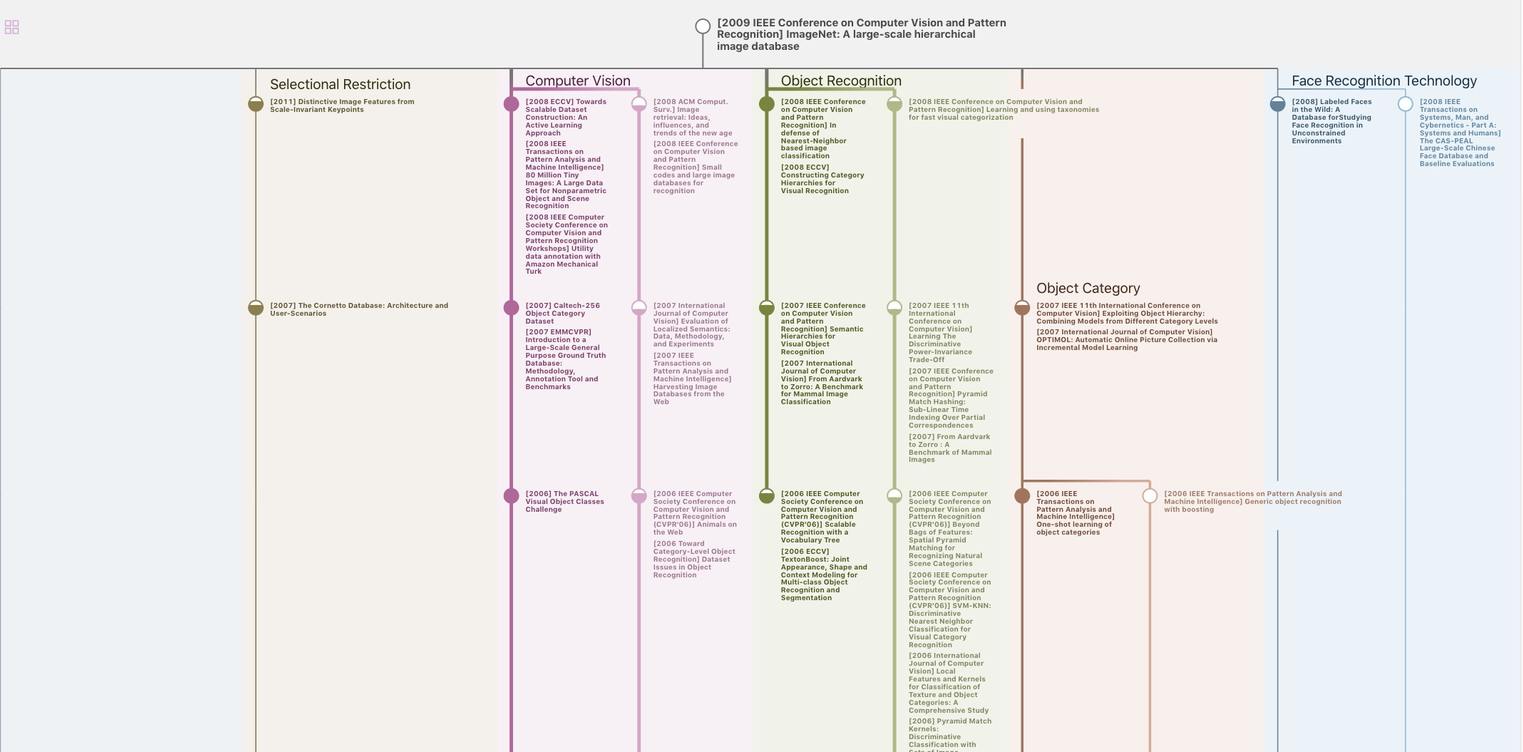
生成溯源树,研究论文发展脉络
Chat Paper
正在生成论文摘要