Tensor representation-based transferability analytics and selective transfer learning of prognostic knowledge for remaining useful life prediction across machines
RELIABILITY ENGINEERING & SYSTEM SAFETY(2024)
摘要
In recent years, deep transfer learning techniques have been successfully applied to solve RUL prediction across different working conditions. However, for RUL prediction across different machines in which the data distribution and fault evolution characteristics vary largely, the extraction and transition of prognostic knowledge become more challenging. Even if fault mode information can assist in the knowledge transfer, model bias will inevitably exist on the target machine with mixed or unknown faults. To address this issue from a transferability perspective, this paper proposes a novel selective transfer learning approach for RUL prediction across machines. First, the paper utilizes the tensor representation to construct the meta-degradation trend of each fault mode and evaluates the transferability of source domain data from fault mode and degradation characteristics through a new cross-machine transfer degree indicator (M-TDI). Second, a Long Short-Term Memory (LSTM)-based selective transfer strategy is proposed using the M-TDIs. The paper designs a training algorithm with an alternating optimization scheme to seek the optimal tensor decomposition and knowledge transfer effect. Theoretical analysis proves that the proposed approach significantly reduces the upper bound of prediction error. Furthermore, experimental results on three benchmark datasets prove the effectiveness of the proposed approach.
更多查看译文
关键词
Remaining useful life prediction,Transfer learning,Transferability analytics,LSTM,Tensor decomposition
AI 理解论文
溯源树
样例
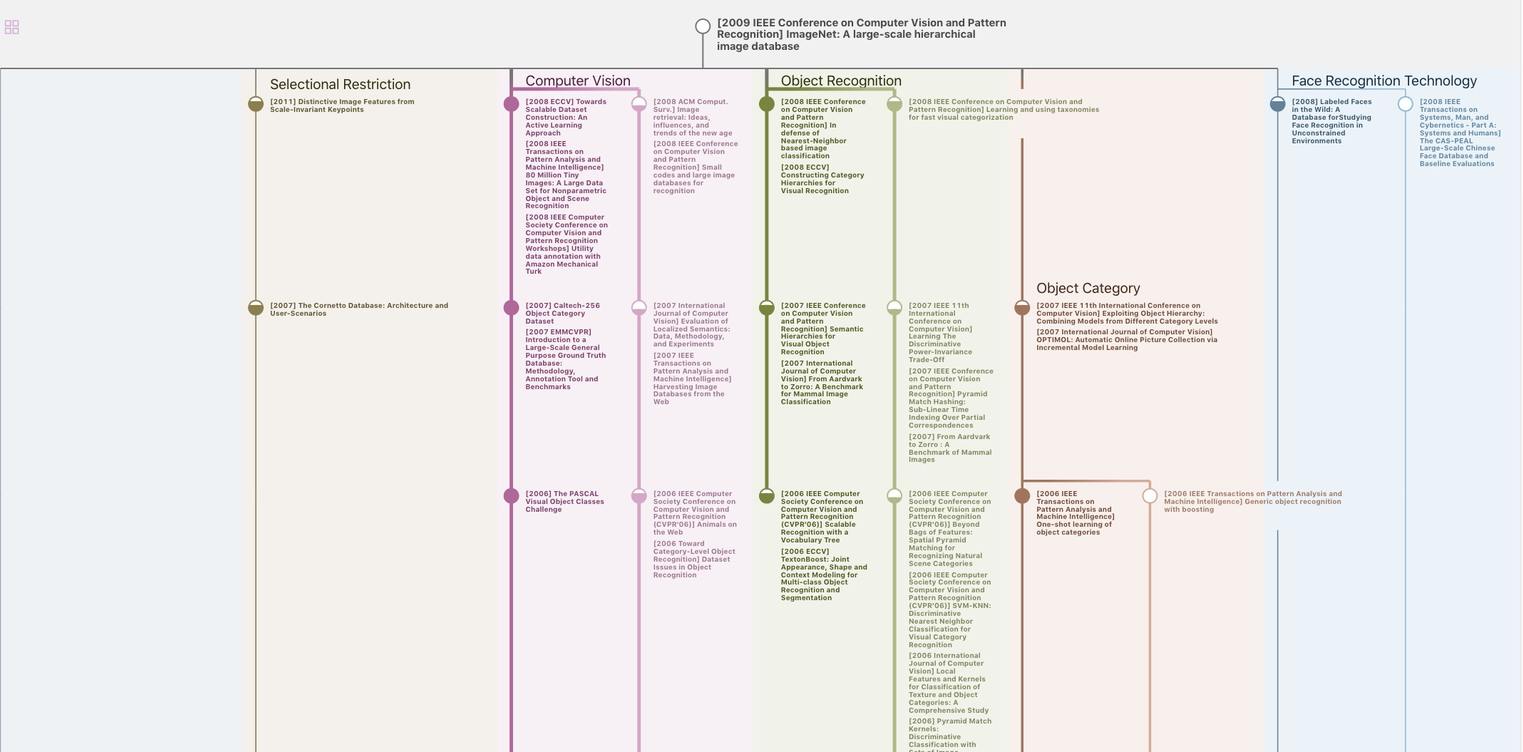
生成溯源树,研究论文发展脉络
Chat Paper
正在生成论文摘要