Master regulators used as breast cancer prognostic markers
Clinical Cancer Research(2008)
摘要
A39 Background: In breast cancer, two recent Gene Expression Profile (GEP) studies identified independent ~70 gene panels that were highly accurate in predicting progression to metastatic cancer [1,2]. However, paradoxically, there was virtually no overlap between the two signatures and both signatures performed poorly when classifying samples from the other study. A key reason for such unstable and study-dependent signatures is that current methods identify “passengers” rather than “drivers” of the phenotypic differences. Rather than identifying genes correlated with the phenotypes of interest, one should look instead for their upstream regulators, causally associated with the observed expression patterns. Here, we show that computationally inferred transcriptional regulation maps can be effectively interrogated for the unbiased inference of Master Regulators that may induce or suppress specific gene signatures associated with poor prognosis.
Methods: We used two datasets of 295 and 286 samples each (hereafter denoted as NKI and Wang datasets) in which the authors had previously determined a 70-gene and a 76-gene prognostic signatures, respectively ( S NKI & S Wang ) [1,2]. We performed the following analysis independently on each of these datasets: (a) First, dataset was processed by the ARACNe algorithm [3] to infer a genome-wide transcriptional interaction network from GEP, (b) then, the network was interrogated using the Fisher Exact Test (FET) and Gene Sets Enrichment Analysis to identify a repertoire of candidate regulators, MR , whose targets were highly overlapping with the signature S , (c) finally, the MR genes were tested as classifiers of the good vs. poor outcome against the S signature, using 5-fold cross-validation based on a Support Vector Machine classifier.
Results: From an unbiased list of 852 Transcription Factors (TF) shared by the two datasets, the analysis produced a list of highly overlapping Master Regulator candidates, with a 30% overlap among the top 20 inferred master regulator TFs ( p = 2×10 -6 , FET), which is especially significant when compared to the 1 gene overlap between the two signatures, S NKI and S Wang ( p = 0.25, FET). To study the stability of MR genes as a function of training set, we repeated this procedure in the NKI dataset, using different GEP subsets to infer prognostic signatures. Results clearly show that while differential expression signatures were highly affected by the training sample choice, with only 8.6% average overlap between the top 70 genes, the inferred master regulator TFs were much more consistent, with a 47.8% overlap between the top 20 genes, despite the highly discordant signatures that were used to infer them. Classification result shows that even a single Master Regulator can predict progression to metastatic tumors with significant higher accuracy than the ≥70-gene signatures. Classification accuracy using the gene-signatures drops significantly (4% - 8%) when NKI signature is used to classify Wang samples or vice-versa, while Master Regulator predictions remained highly predictive.
Conclusions: The inferred master regulators were much better conserved across training sets, consistently outperformed differentially expressed signatures in cross-validation studies, and were better able to classify samples in independent studies.
References 1. van’t Veer LJ et al. Nature 2002
2. Wang Y et al. Lancet 2005
3. Margolin AA et al. Nat Protocols 2006
更多查看译文
AI 理解论文
溯源树
样例
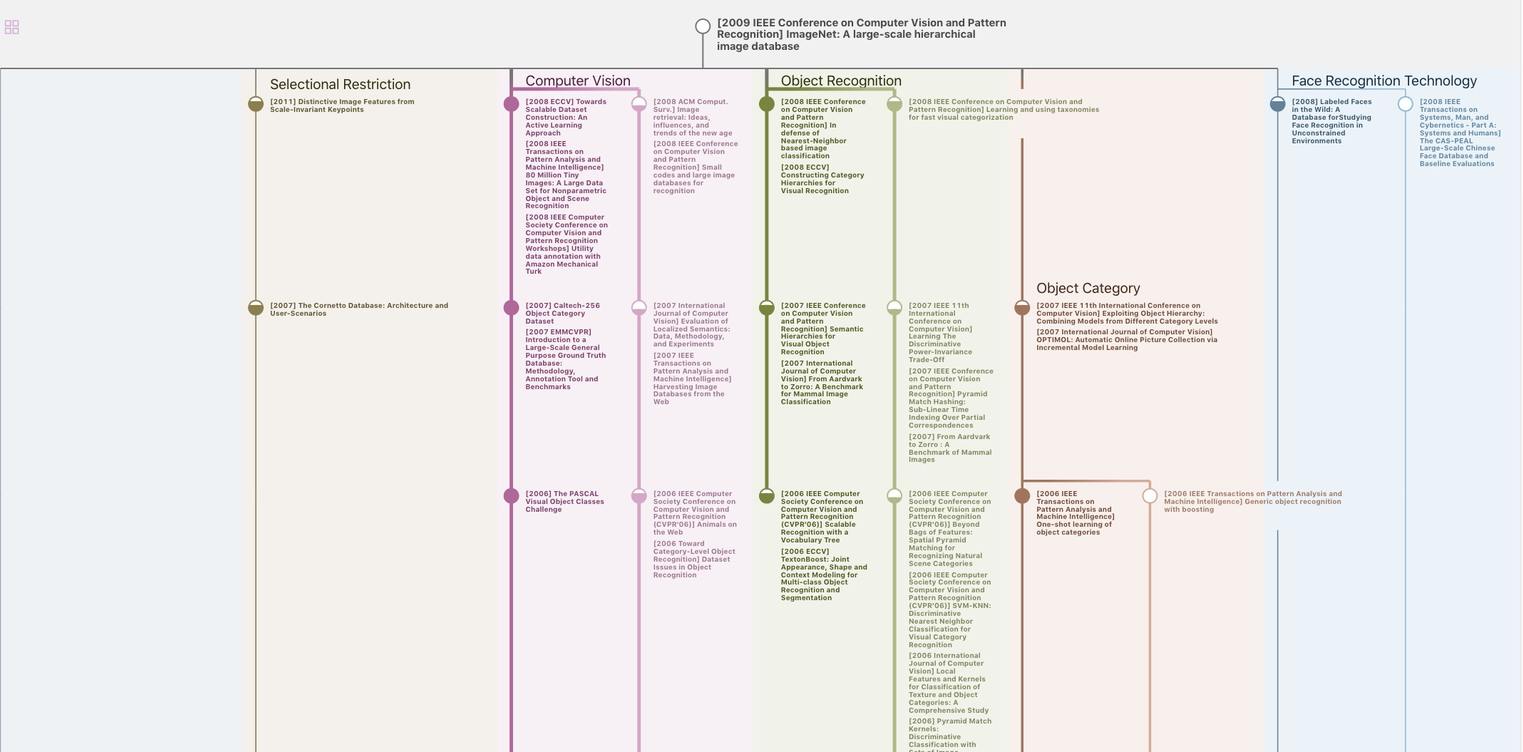
生成溯源树,研究论文发展脉络
Chat Paper
正在生成论文摘要