Longitudinal conditional models with intermittent missingness: SAS code and applications
JOURNAL OF STATISTICAL COMPUTATION AND SIMULATION(2014)
Abstract
This work provides a set of macros performed with SAS (Statistical Analysis System) for Windows, which can be used to fit conditional models under intermittent missingness in longitudinal data. A formalized transition model, including random effects for individuals and measurement error, is presented. Model fitting is based on the missing completely at random or missing at random assumptions, and the separability condition. The problem translates to maximization of the marginal observed data density only, which for Gaussian data is again Gaussian, meaning that the likelihood can be expressed in terms of the mean and covariance matrix of the observed data vector. A simulation study is presented and misspecification issues are considered. A practical application is also given, where conditional models are fitted to the data from a clinical trial that assessed the effect of a Cuban medicine on a disease of the respiratory system.
MoreTranslated text
Key words
transition model,auto-regressive sequences,likelihood,missing data mechanism,macro
AI Read Science
Must-Reading Tree
Example
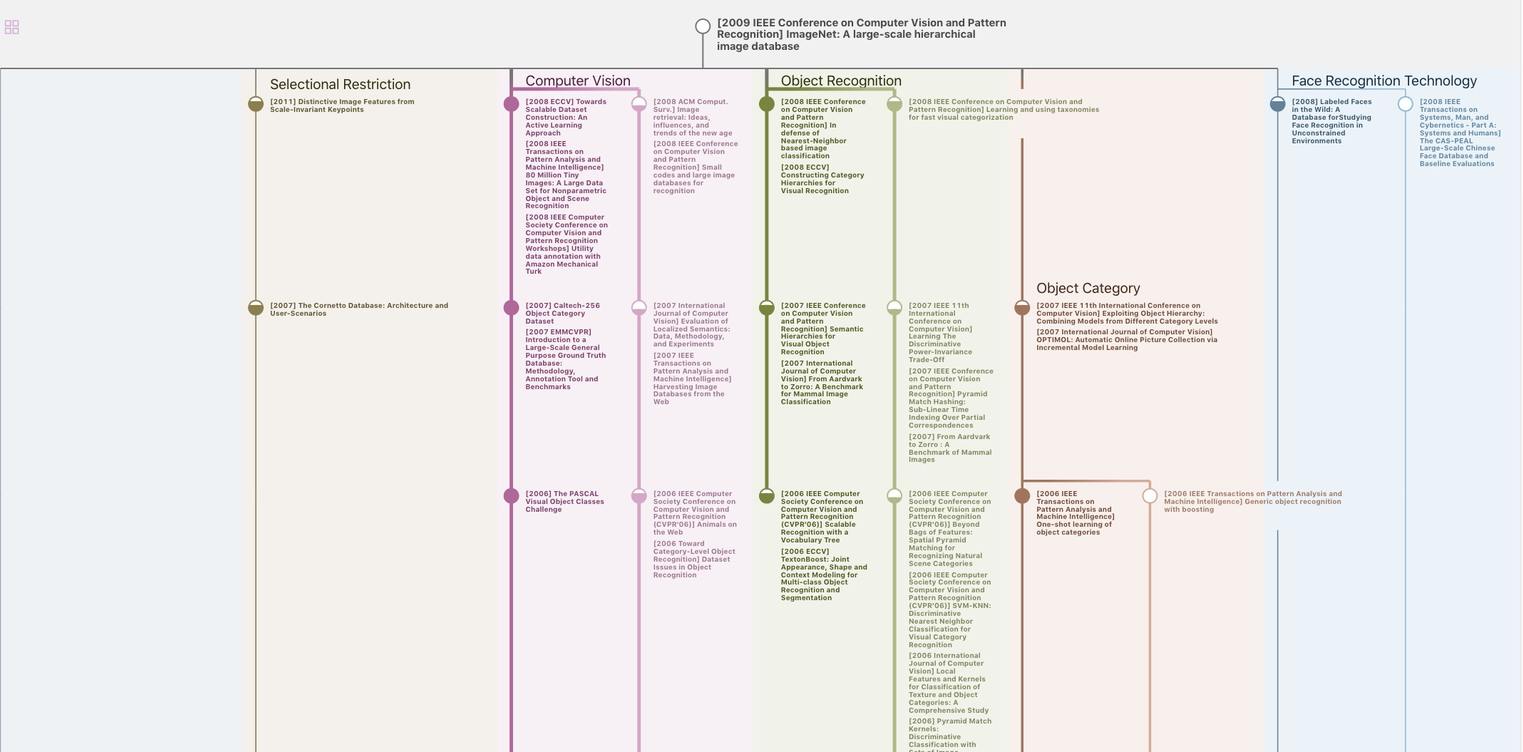
Generate MRT to find the research sequence of this paper
Chat Paper
Summary is being generated by the instructions you defined