Hit and Run ARMS: Adaptive Rejection Metropolis Sampling with Hit and Run Random Direction
JOURNAL OF STATISTICAL COMPUTATION AND SIMULATION(2016)
摘要
An algorithm for sampling from non-log-concave multivariate distributions is proposed, which improves the adaptive rejection Metropolis sampling (ARMS) algorithm by incorporating the hit and run sampling. It is not rare that the ARMS is trapped away from some subspace with significant probability in the support of the multivariate distribution. While the ARMS updates samples only in the directions that are parallel to dimensions, our proposed method, the hit and run ARMS (HARARMS), updates samples in arbitrary directions determined by the hit and run algorithm, which makes it almost not possible to be trapped in any isolated subspaces. The HARARMS performs the same as ARMS in a single dimension while more reliable in multidimensional spaces. Its performance is illustrated by a Bayesian free-knot spline regression example. We showed that it overcomes the well-known lethargy' property and decisively find the global optimal number and locations of the knots of the spline function.
更多查看译文
关键词
adaptive rejection metropolis sampling,hit and run algorithm,regression splines,free-knot splines,empirical Bayesian method
AI 理解论文
溯源树
样例
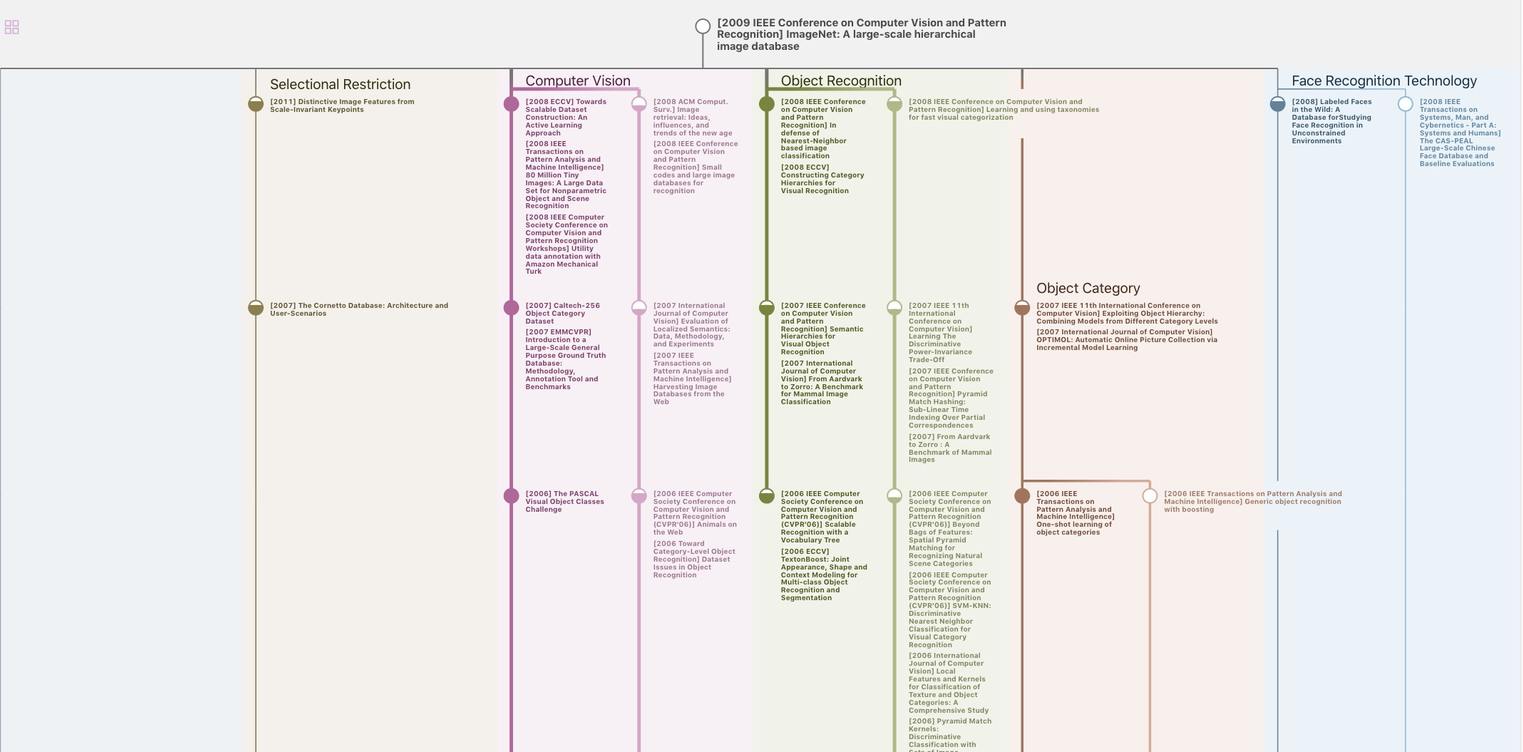
生成溯源树,研究论文发展脉络
Chat Paper
正在生成论文摘要