Ensembles of evolutionarily-constructed support vector machine cascades
KNOWLEDGE-BASED SYSTEMS(2024)
Abstract
Support vector machine (SVM) is a well -established and widely -used classifier, but it is also known for high time and memory complexity of the training process. Coupled with its sensitivity to the model hyperparameters, it is often quite challenging to apply SVMs in practice to classify real -life data. At the same time, classifier ensembles composed of many light base models are known to achieve high classification performance, but SVMs are not exploited extensively here. In this paper, we propose a new approach that consists in creating an ensemble of SVM cascades, each of which is constructed using an evolutionary algorithm for training set selection and hyperparameter optimization. The obtained ensembles allow for high classification performance and they scale well for large datasets. The results of our experimental validation performed for over 40 datasets indicate that the proposed technique is competitive compared with the state-of-the-art methods for constructing ensembles. To deeper understand the proposed technique, we report and discuss the outcome of an extensive ablation study coupled with visualizations obtained for 2D datasets that enrich our qualitative analysis.
MoreTranslated text
Key words
Support vector machine,Evolutionary algorithm,Ensemble learning,Training set selection,Hyperparameters optimization
AI Read Science
Must-Reading Tree
Example
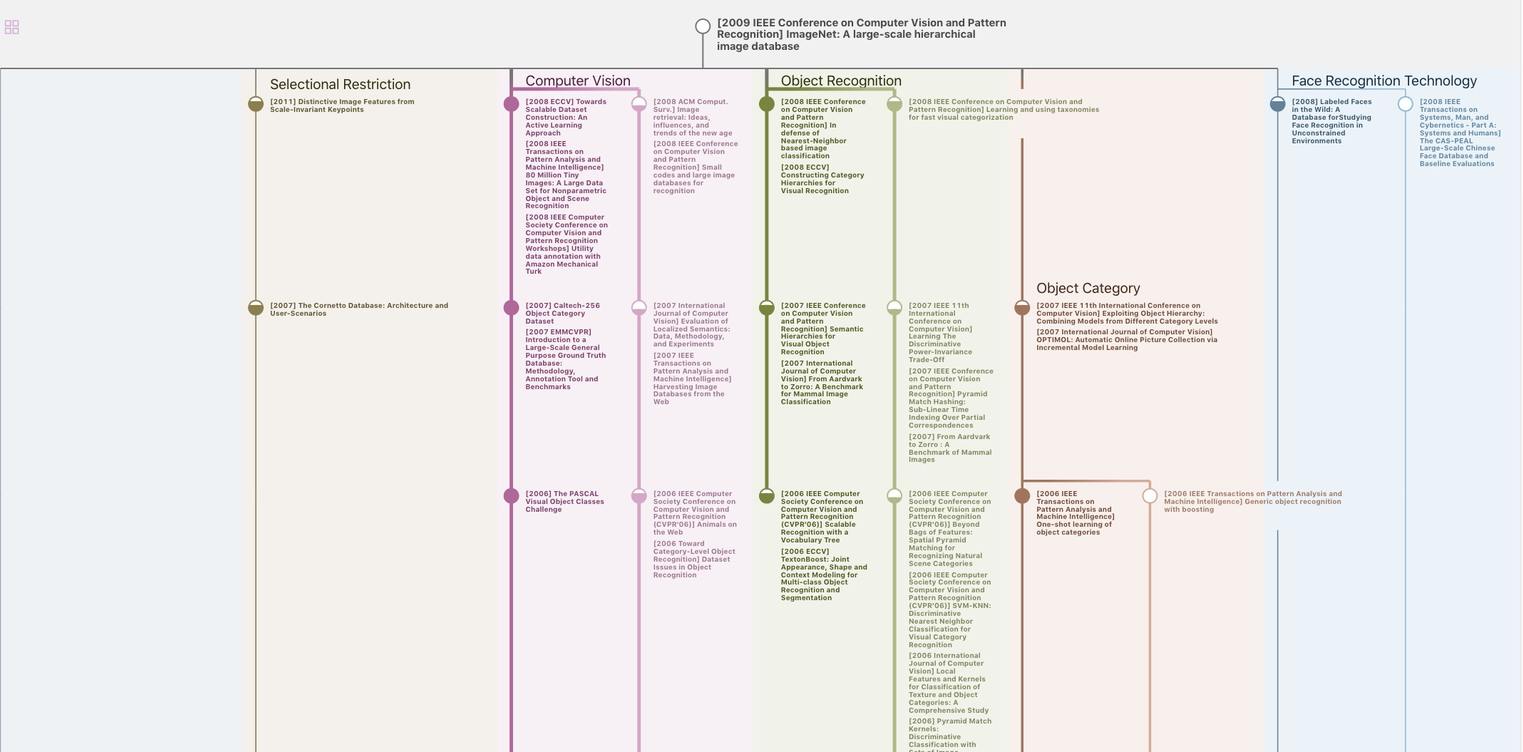
Generate MRT to find the research sequence of this paper
Chat Paper
Summary is being generated by the instructions you defined