Estimating Forest Canopy Density Of Tropical Mixed Deciduous Vegetation Using Landsat Data: A Comparison Of Three Classification Approaches
INTERNATIONAL JOURNAL OF REMOTE SENSING(2012)
Abstract
Although a number of image classification approaches are available to estimate forest canopy density (FCD) using satellite data, assessment of their relative performances with tropical mixed deciduous vegetation is lacking. This study compared three image classification approaches - maximum likelihood classification (MLC), multiple linear regression (MLR) and FCD Mapper - in estimating the FCD of mixed deciduous forest in Myanmar. The application of MLC and MLR was based on spectral reflectance of vegetation, whereas FCD Mapper was operated on integrating the biophysical indices derived from the reflectance of the vegetation. The FCD was classified into four categories: closed canopy forest (CCF; FCD >= 70%), medium canopy forest (MCF; 40% >= FCD < 70%), open canopy forest (OCF; 10% >= FCD < 40%) and non-forest (NF; FCD < 10%). In the three classification approaches, producer's and user's accuracies were higher for more homogeneous vegetation such as NF and CCF than for heterogeneous vegetation density (VD) such as OCF and MCF. FCD Mapper produced the best overall accuracy and kappa coefficient. This study revealed that only spectral reflectance is not enough to get good results in estimating FCD in tropical mixed deciduous vegetation. This study indicates that FCD Mapper, an inexpensive approach because it requires only validation data and thus saves time, can be applied to monitor tropical mixed deciduous vegetation over time at lower cost than alternative methods.
MoreTranslated text
AI Read Science
Must-Reading Tree
Example
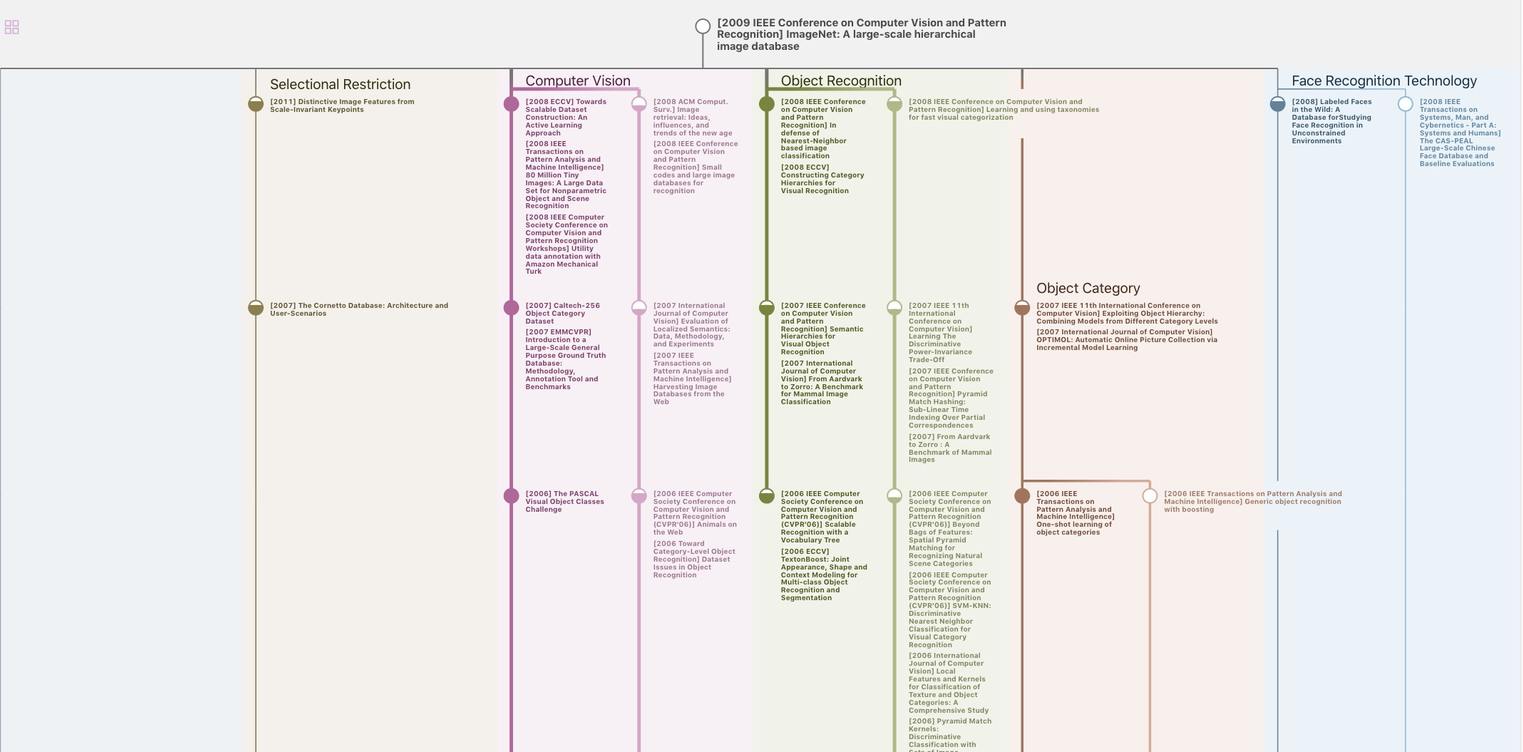
Generate MRT to find the research sequence of this paper
Chat Paper
Summary is being generated by the instructions you defined