Inverse spatial principal component analysis for geophysical survey data interpolation
Journal of Applied Geophysics(2015)
Abstract
The starting point for data processing, visualization, and overlay with other data sources in geological applications often involves building a regular grid by interpolation of geophysical measurements. Typically, the sampling interval along survey lines is much higher than the spacing between survey lines because the geophysical recording system is able to operate with a high sampling rate, while the costs and slower speeds associated with operational platforms limit line spacing. However, currently available interpolating methods often smooth data observed with higher sampling rate along a survey line to accommodate the lower spacing across lines, and much of the higher resolution information is not captured in the interpolation process. In this approach, a method termed as the inverse spatial principal component analysis (isPCA) is developed to address this problem. In the isPCA method, a whole profile observation as well as its line position is handled as an entity and a survey collection of line entities is analyzed for interpolation. To test its performance, the developed isPCA method is used to process a simulated airborne magnetic survey from an existing magnetic grid offshore the Atlantic coast of Canada. The interpolation results using the isPCA method and other methods are compared with the original survey grid. It is demonstrated that the isPCA method outperforms the Inverse Distance Weighting (IDW), Kriging (Geostatistical), and MINimum Curvature (MINC) interpolation methods in retaining detailed anomaly structures and restoring original values. In a second test, a high resolution magnetic survey offshore Cape Breton, Nova Scotia, Canada, was processed and the results are compared with other geological information. This example demonstrates the effective performance of the isPCA method in basin structure identification.
MoreTranslated text
Key words
Inverse spatial principal component analysis,Interpolation,Filtering methods,Singular spectral analysis,Airborne geophysics
AI Read Science
Must-Reading Tree
Example
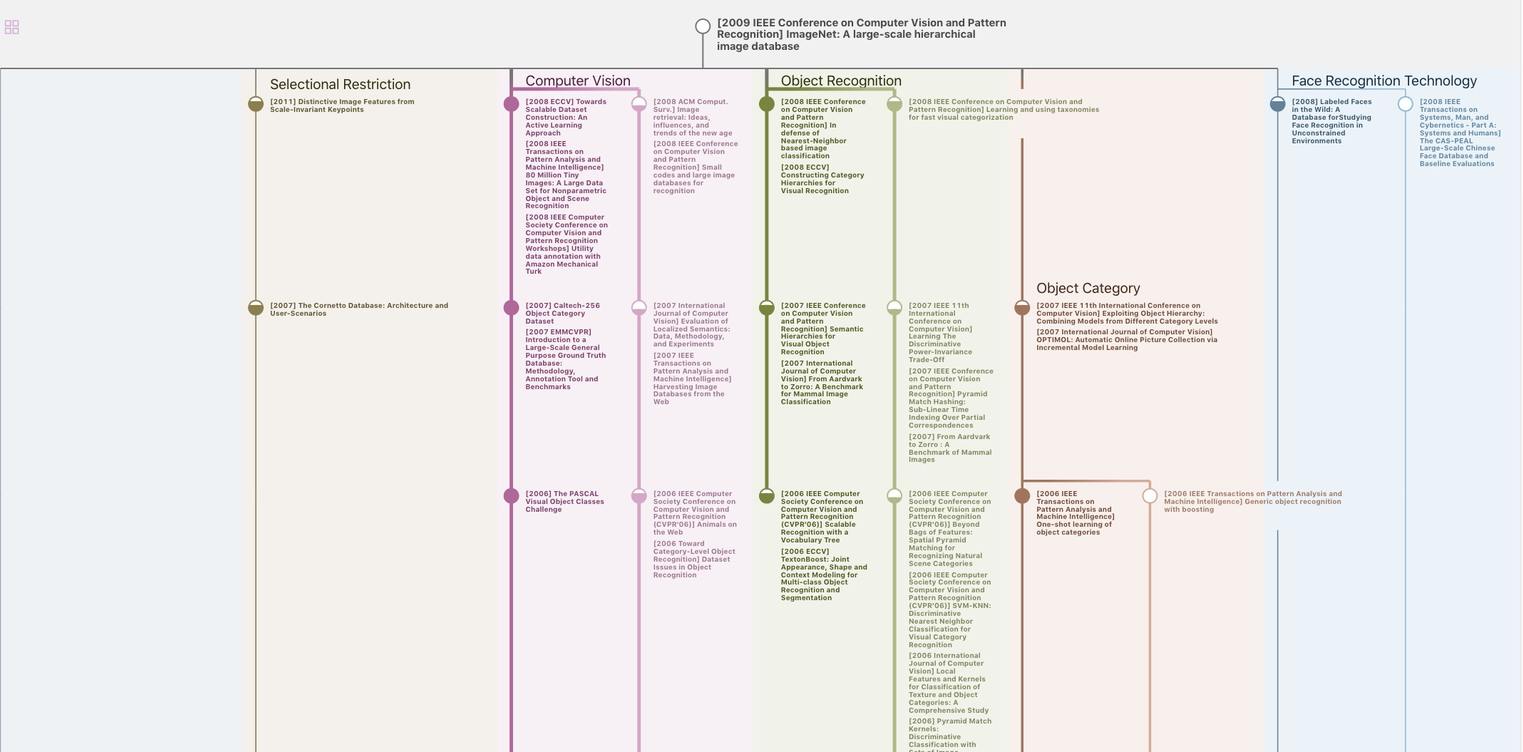
Generate MRT to find the research sequence of this paper
Chat Paper
Summary is being generated by the instructions you defined