Analysis of entropies based on empirical mode decomposition in amnesic mild cognitive impairment of diabetes mellitus
JOURNAL OF INNOVATIVE OPTICAL HEALTH SCIENCES(2015)
摘要
EEG characteristics that correlate with the cognitive functions are important in detecting mild cognitive impairment (MCI) in T2DM. To investigate the complexity between aMCI group and age-matched non-aMCI control group in T2DM, six entropies combining empirical mode decomposition (EMD), including Approximate entropy (ApEn), Sample entropy (SaEn), Fuzzy entropy (FEn), Permutation entropy (PEn), Power spectrum entropy (PsEn) and Wavelet entropy (WEn) were used in the study. A feature extraction technique based on maximization of the area under the curve (AUC) and a support vector machine (SVM) were subsequently used to for features selection and classification. Finally, Pearson's linear correlation was employed to study associations between these entropies and cognitive functions. Compared to other entropies, FEn had a higher classification accuracy, sensitivity and specificity of 68%, 67.1% and 71.9%, respectively. Top 43 salient features achieved classification accuracy, sensitivity and specificity of 73.8%, 72.3% and 77.9%, respectively. P4, T4 and C4 were the highest ranking salient electrodes. Correlation analysis showed that FEn based on EMD was positively correlated to memory at electrodes F7, F8 and P4, and PsEn based on EMD was positively correlated to Montreal cognitive assessment (MoCA) and memory at electrode T4. In sum, FEn based on EMD in right-temporal and occipital regions may be more suitable for early diagnosis of the MCI with T2DM.
更多查看译文
关键词
Entropy,empirical mode decomposition,amnestic mild cognitive impairment,type 2 diabetes mellitus
AI 理解论文
溯源树
样例
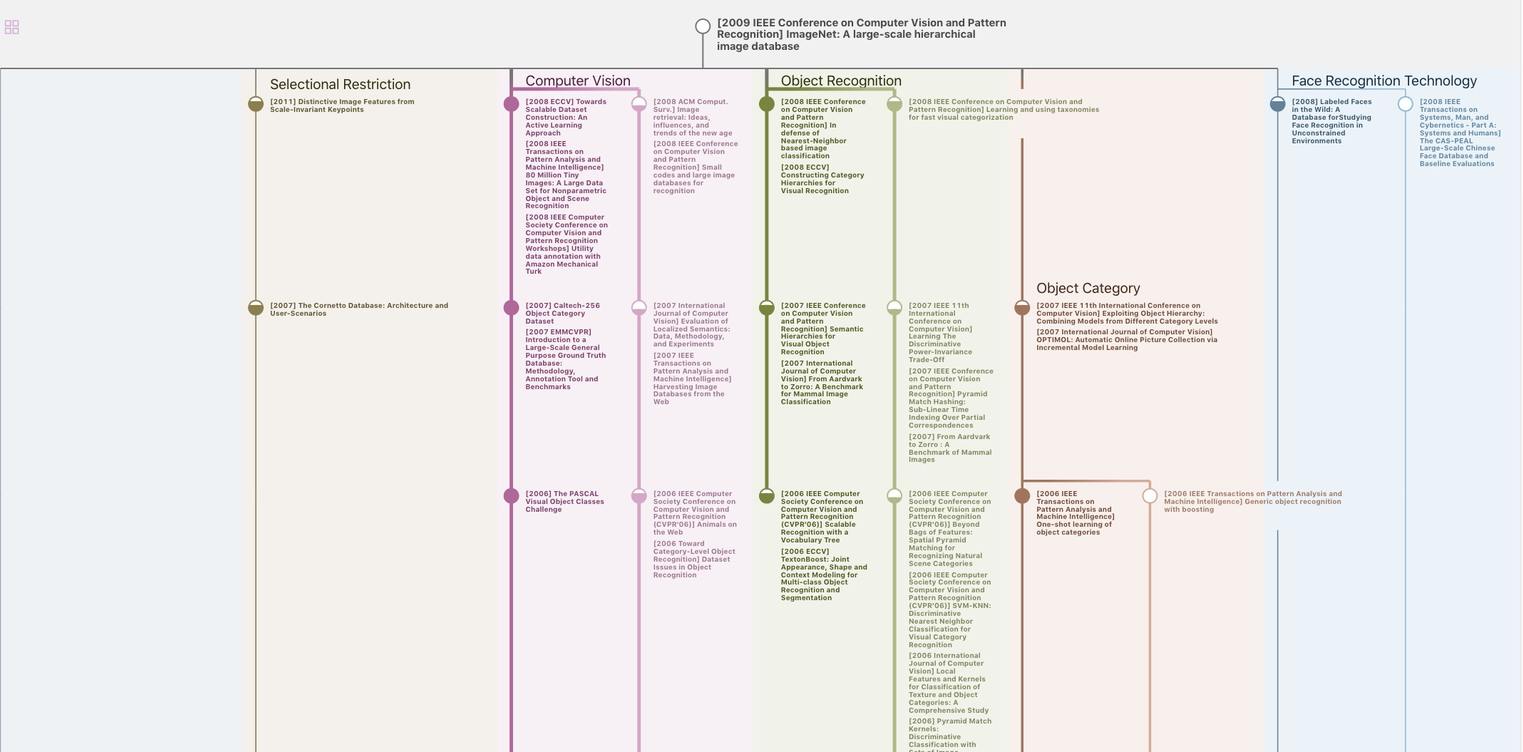
生成溯源树,研究论文发展脉络
Chat Paper
正在生成论文摘要