Automatic de-identification of electronic medical records using token-level and character-level conditional random fields
Journal of Biomedical Informatics(2015)
摘要
De-identification, identifying and removing all protected health information (PHI) present in clinical data including electronic medical records (EMRs), is a critical step in making clinical data publicly available. The 2014 i2b2 (Center of Informatics for Integrating Biology and Bedside) clinical natural language processing (NLP) challenge sets up a track for de-identification (track 1). In this study, we propose a hybrid system based on both machine learning and rule approaches for the de-identification track. In our system, PHI instances are first identified by two (token-level and character-level) conditional random fields (CRFs) and a rule-based classifier, and then are merged by some rules. Experiments conducted on the i2b2 corpus show that our system submitted for the challenge achieves the highest micro F-scores of 94.64%, 91.24% and 91.63% under the 'token', 'strict' and 'relaxed' criteria respectively, which is among top-ranked systems of the 2014 i2b2 challenge. After integrating some refined localization dictionaries, our system is further improved with F-scores of 94.83%, 91.57% and 91.95% under the 'token', 'strict' and 'relaxed' criteria respectively. © 2015 Elsevier Inc..
更多查看译文
关键词
De-identification,Electronic medical records,Hybrid method,Natural language processing,Protected health information,i2b2
AI 理解论文
溯源树
样例
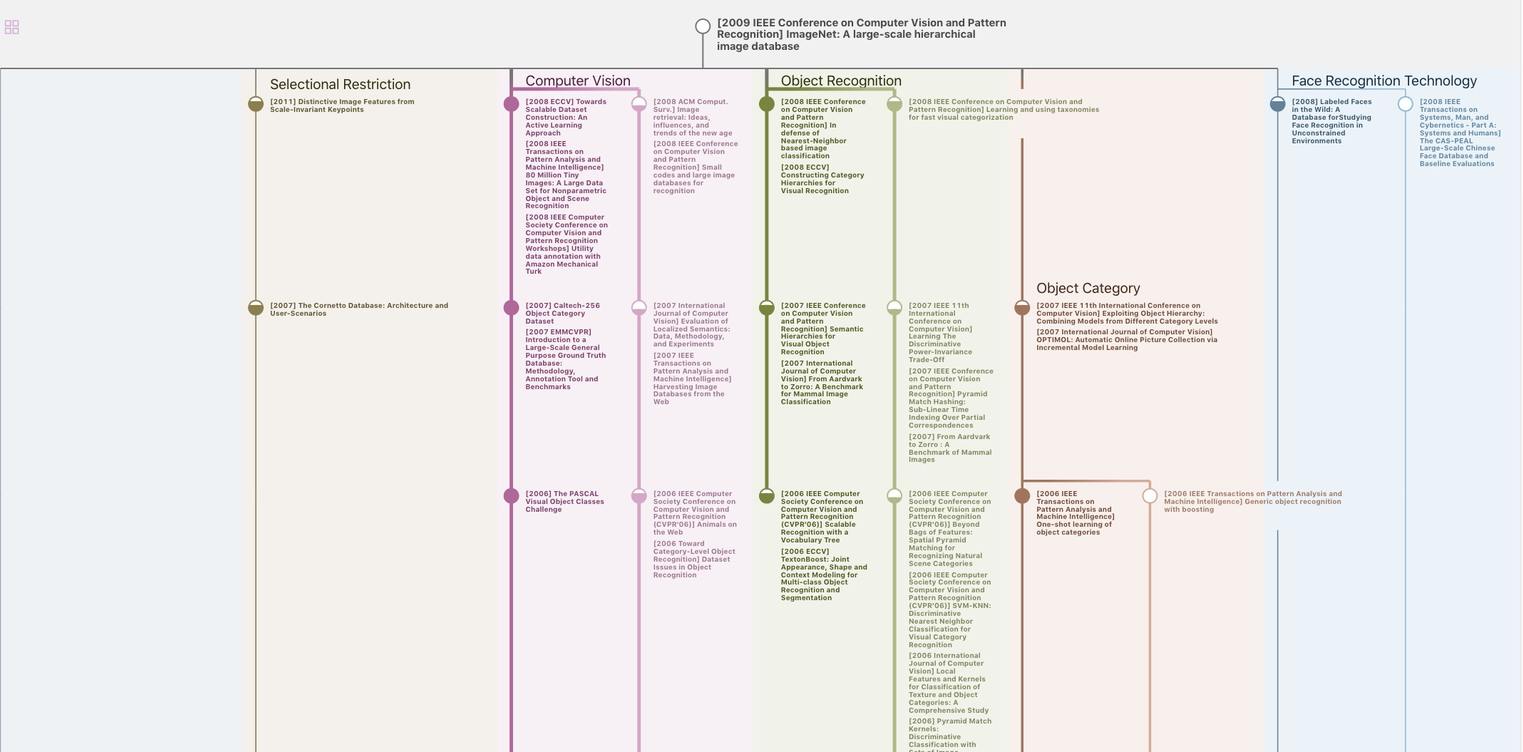
生成溯源树,研究论文发展脉络
Chat Paper
正在生成论文摘要