On the equivalence between regularized NMF and similarity-augmented graph partitioning
ESANN(2015)
摘要
Many papers pointed out the interest of (co-)clustering both data and features in a dataset to obtain better performances than methods focused on data only. In addition, recent work have shown that data and features lie in low dimensional manifolds embedded into the original space and this information has been introduced as regularization terms in clustering objectives. Very popular and recent examples are regularized NMF algorithms. However, these techniques have difficulties to avoid local optima and require high computation times, making them inadequate for large scale data. In this paper, we show that NMF with manifolds regularization on a binary matrix is mathematically equivalent to an edge-cut partitioning in a graph augmented with manifolds information in the case of hard co-clustering. Based on these results, we explore experimentally the efficiency of regularized graph partitioning methods for hard co- clustering on more relaxed datasets and show that regularized multi-level graph partitioning is much faster and often find better clustering results than regularized NMF, and other well-known algorithms.
更多查看译文
关键词
regularized nmf,graph,similarity-augmented
AI 理解论文
溯源树
样例
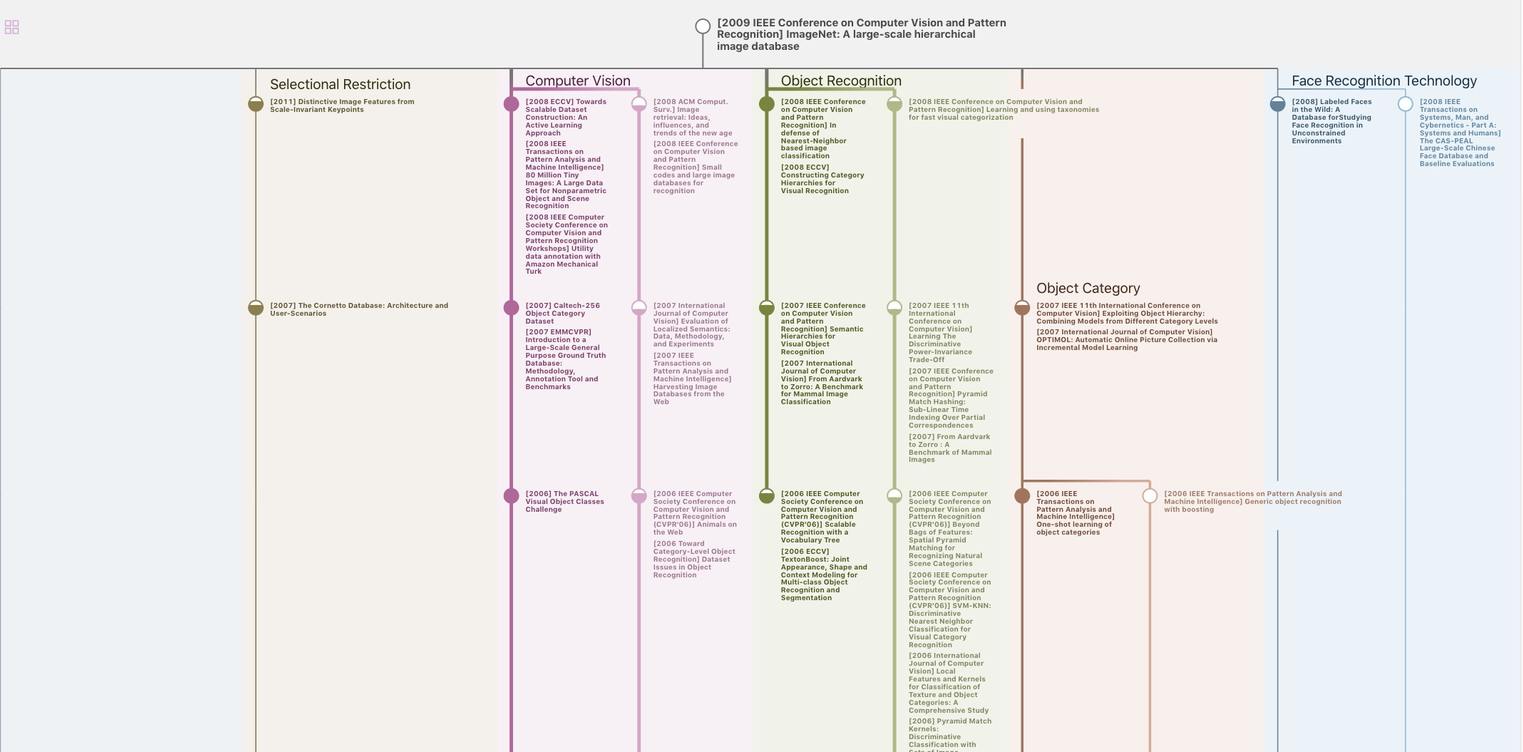
生成溯源树,研究论文发展脉络
Chat Paper
正在生成论文摘要