Universalization of narrow methods: Case study on autoencoders
2014 IEEE 3rd International Conference on Cloud Computing and Intelligence Systems(2014)
摘要
The problem of bridging the gap between efficient but narrow methods of machine learning, and universal but inefficient methods was considered. Our main claim, which is methodologically important to the field of Artificial General Intelligence (AGI), is that neither narrow nor basic universal methods are sufficient for AGI. This claim was illustrated on example of pattern recognition task using stacked autoencoders and their two extensions with more exhaustive search and richer solution space. These three types of classifiers were evaluated on the base of a criterion that account for both error rate and training time. Depending on the urgency of the task to be solved, less or more universal methods appeared to be better. Thus, AGI might start with narrow methods, but should be able to perform their “universalization” (i.e. extension of the model space possibly up to Turing-complete space if it is appropriate in a certain situation).
更多查看译文
关键词
Narrow methods,Artificial General Intelligence (AGI),Stacked autoencoders
AI 理解论文
溯源树
样例
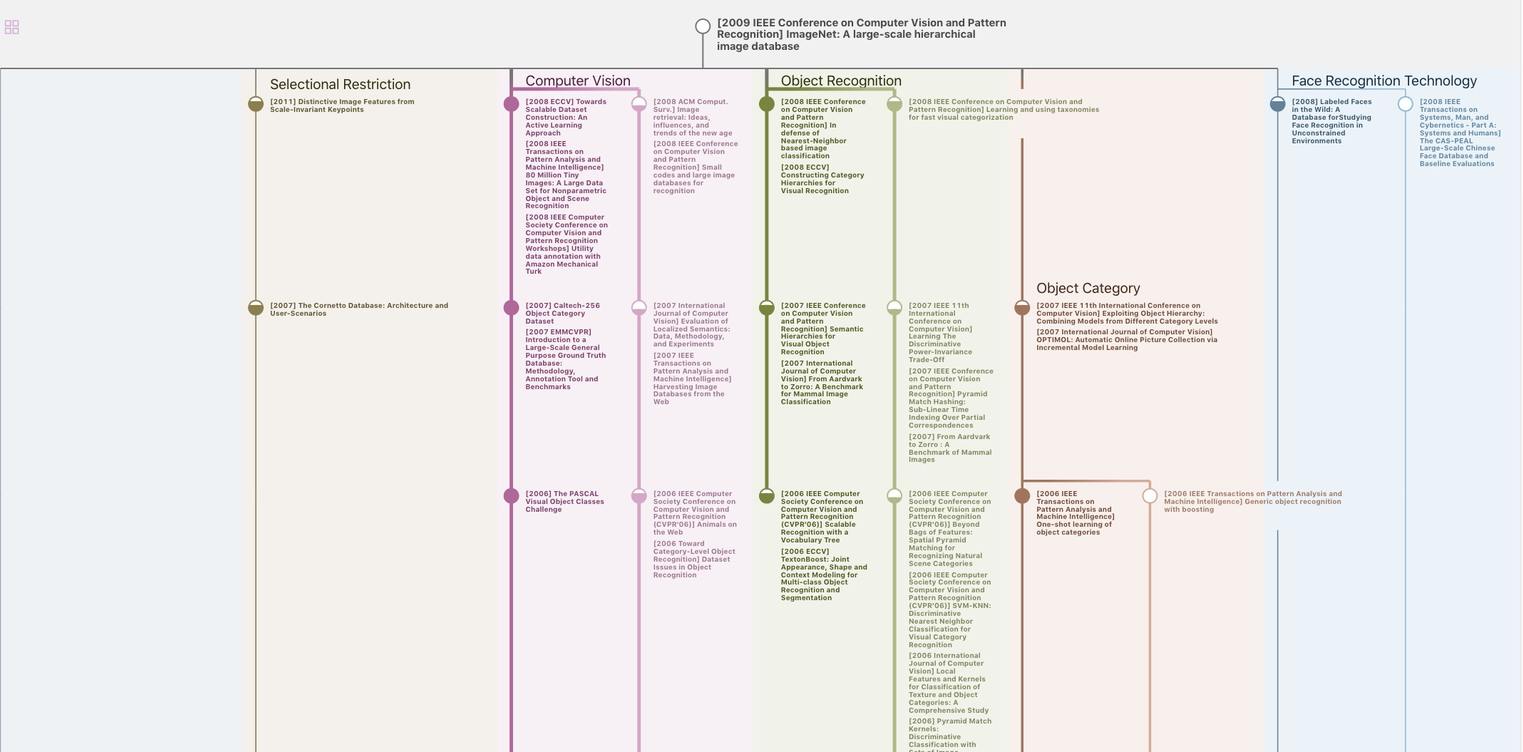
生成溯源树,研究论文发展脉络
Chat Paper
正在生成论文摘要