Partial Least Squares Regression Can Aid in Detecting Differential Abundance of Multiple Features in Sets of Metagenomic Samples.
Frontiers in genetics(2015)
摘要
It is now feasible to examine the composition and diversity of microbial communities (i.e., "microbiomes") that populate different human organs and orifices using DNA sequencing and related technologies. To explore the potential links between changes in microbial communities and various diseases in the human body, it is essential to test associations involving different species within and across microbiomes, environmental settings and disease states. Although a number of statistical techniques exist for carrying out relevant analyses, it is unclear which of these techniques exhibit the greatest statistical power to detect associations given the complexity of most microbiome datasets. We compared the statistical power of principal component regression, partial least squares regression, regularized regression, distance-based regression, Hill's diversity measures, and a modified test implemented in the popular and widely used microbiome analysis methodology "Metastats" across a wide range of simulated scenarios involving changes in feature abundance between two sets of metagenomic samples. For this purpose, simulation studies were used to change the abundance of microbial species in a real dataset from a published study examining human hands. Each technique was applied to the same data, and its ability to detect the simulated change in abundance was assessed. We hypothesized that a small subset of methods would outperform the rest in terms of the statistical power. Indeed, we found that the Metastats technique modified to accommodate multivariate analysis and partial least squares regression yielded high power under the models and data sets we studied. The statistical power of diversity measure-based tests, distance-based regression and regularized regression was significantly lower. Our results provide insight into powerful analysis strategies that utilize information on species counts from large microbiome data sets exhibiting skewed frequency distributions obtained on a small to moderate number of samples.
更多查看译文
AI 理解论文
溯源树
样例
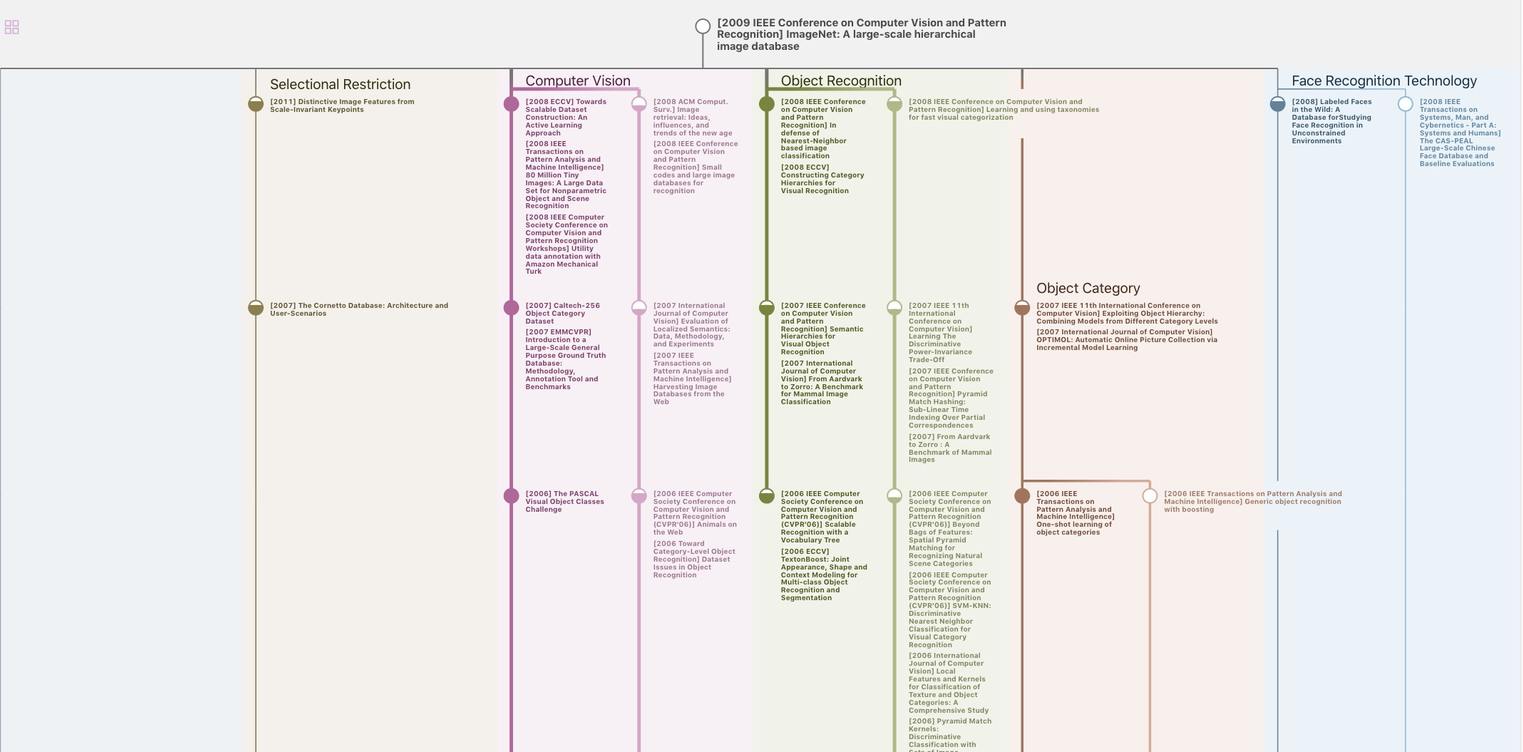
生成溯源树,研究论文发展脉络
Chat Paper
正在生成论文摘要