Feature Extraction Using Power-Law Adjusted Linear Prediction With Application to Speaker Recognition Under Severe Vocal Effort Mismatch
Audio, Speech, and Language Processing, IEEE/ACM Transactions(2016)
摘要
Linear prediction is one of the most established techniques in signal estimation, and it is widely utilized in speech signal processing. It has been long understood that the nerve firing rate of human auditory system can be approximated by power law non-linearity, and this has been the motivation behind using perceptual linear prediction in extracting acoustic features in a variety of speech processing applications. In this paper, we revisit the application of power law non-linearity in speech spectrum estimation by compressing/expanding power spectrum in autocorrelation-based linear prediction. The development of so-called LP- α is motivated by a desire to obtain spectral features that present less mismatch than conventionally used spectrum estimation methods when speech of normal loudness is compared to speech under vocal effort. The effectiveness of the proposed approach is demonstrated in a speaker recognition task conducted under severe vocal effort mismatch comparing shouted versus normal speech mode.
更多查看译文
关键词
acoustic signal processing,feature extraction,speaker recognition,speech processing,acoustic features,autocorrelation-based linear prediction,compressing-expanding power spectrum,feature extraction,human auditory system,nerve firing rate,perceptual linear prediction,power law nonlinearity,power-law adjusted linear prediction,severe vocal effort mismatch,signal estimation,speaker recognition,speech processing,speech signal processing,speech spectrum estimation,Speaker recognition,linear prediction,mismatch,power- law,shouting,vocal effort
AI 理解论文
溯源树
样例
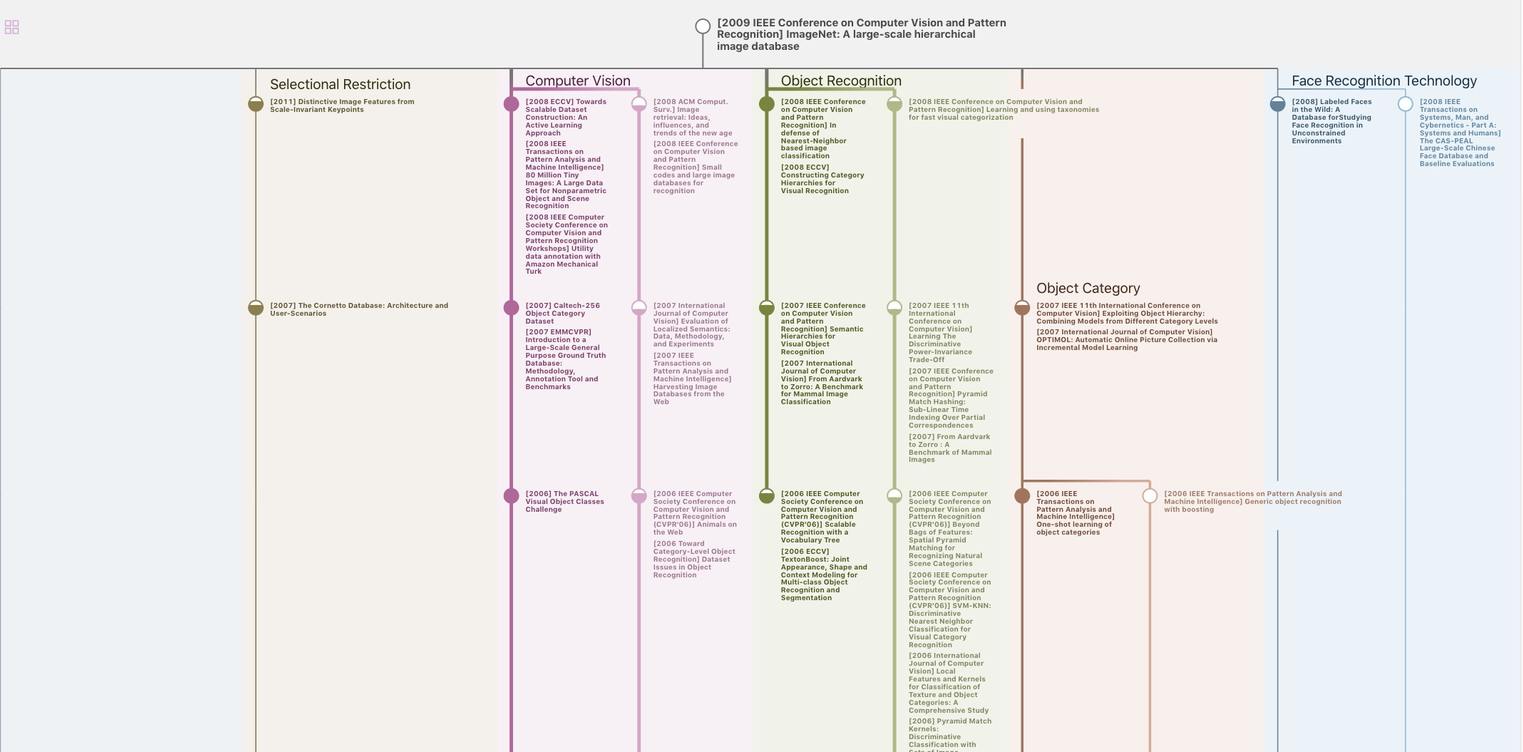
生成溯源树,研究论文发展脉络
Chat Paper
正在生成论文摘要