Network inference through synergistic subnetwork evolution
EURASIP J. Bioinformatics and Systems Biology(2015)
摘要
Study of signaling networks is important for a better understanding of cell behaviors e.g., growth, differentiation, metabolism, proptosis, and gaining deeper insights into the molecular mechanisms of complex diseases. While there have been many successes in developing computational approaches for identifying potential genes and proteins involved in cell signaling, new methods are needed for identifying network structures that depict underlying signal cascading mechanisms. In this paper, we propose a new computational approach for inferring signaling network structures from overlapping gene sets related to the networks. In the proposed approach, a signaling network is represented as a directed graph and is viewed as a union of many active paths representing linear and overlapping chains of signal cascading activities in the network. Gene sets represent the sets of genes participating in active paths without prior knowledge of the order in which genes occur within each path. From a compendium of unordered gene sets, the proposed algorithm reconstructs the underlying network structure through evolution of synergistic active paths. In our context, the extent of edge overlapping among active paths is used to define the synergy present in a network. We evaluated the performance of the proposed algorithm in terms of its convergence and recovering true active paths by utilizing four gene set compendiums derived from the KEGG database. Evaluation of results demonstrate the ability of the algorithm in reconstructing the underlying networks with high accuracy and precision.
更多查看译文
关键词
Genetic Algorithm, Signaling Network, Active Path, Fitness Score, Candidate Network
AI 理解论文
溯源树
样例
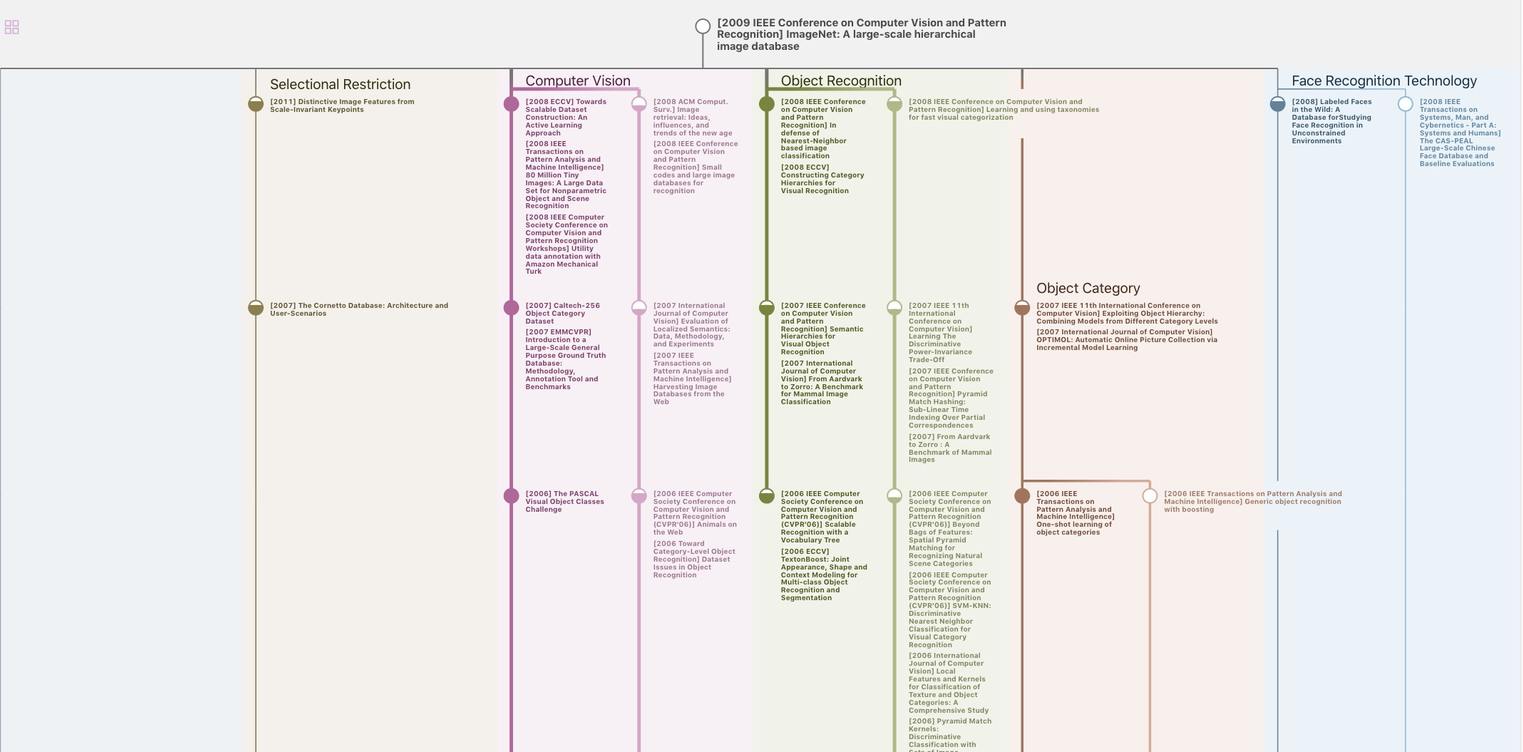
生成溯源树,研究论文发展脉络
Chat Paper
正在生成论文摘要