Predicting drug side effects by multi-label learning and ensemble learning
BMC BIOINFORMATICS(2015)
Abstract
Background Predicting drug side effects is an important topic in the drug discovery. Although several machine learning methods have been proposed to predict side effects, there is still space for improvements. Firstly, the side effect prediction is a multi-label learning task, and we can adopt the multi-label learning techniques for it. Secondly, drug-related features are associated with side effects, and feature dimensions have specific biological meanings. Recognizing critical dimensions and reducing irrelevant dimensions may help to reveal the causes of side effects. Methods In this paper, we propose a novel method ‘feature selection-based multi-label k-nearest neighbor method’ (FS-MLKNN), which can simultaneously determine critical feature dimensions and construct high-accuracy multi-label prediction models. Results Computational experiments demonstrate that FS-MLKNN leads to good performances as well as explainable results. To achieve better performances, we further develop the ensemble learning model by integrating individual feature-based FS-MLKNN models. When compared with other state-of-the-art methods, the ensemble method produces better performances on benchmark datasets. Conclusions In conclusion, FS-MLKNN and the ensemble method are promising tools for the side effect prediction. The source code and datasets are available in the Additional file 1 .
MoreTranslated text
Key words
Side effects,Multi-label learning,Ensemble learning
AI Read Science
Must-Reading Tree
Example
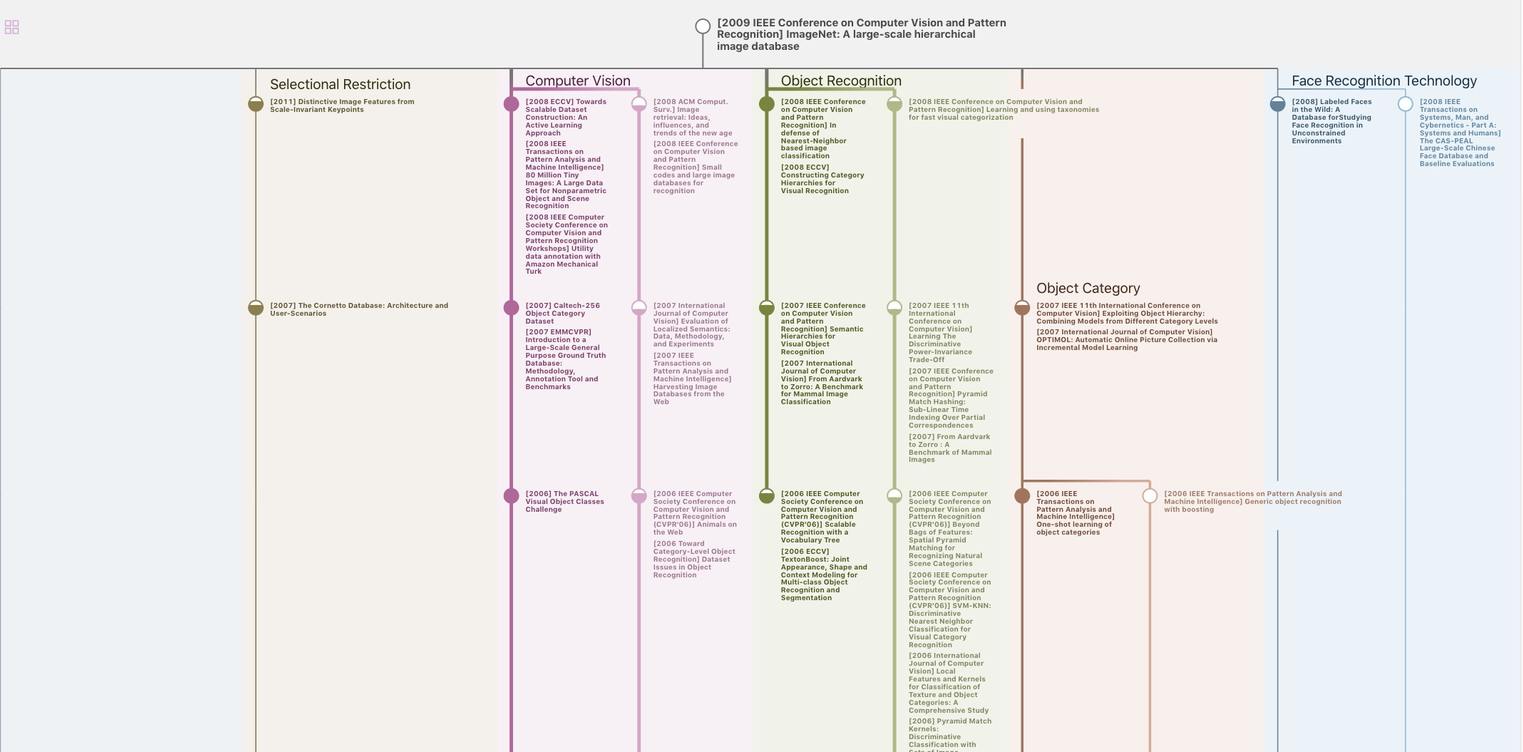
Generate MRT to find the research sequence of this paper
Chat Paper
Summary is being generated by the instructions you defined