Lessons Learned from a Cross-Model Validation between a Discrete Event Simulation Model and a Cohort State-Transition Model for Personalized Breast Cancer Treatment.
MEDICAL DECISION MAKING(2016)
摘要
Objectives. Breast cancer is the most common malignancy among women in developed countries. We developed a model (the Oncotyrol breast cancer outcomes model) to evaluate the cost-effectiveness of a 21-gene assay when used in combination with Adjuvant! Online to support personalized decisions about the use of adjuvant chemotherapy. The goal of this study was to perform a cross-model validation. Methods. The Oncotyrol model evaluates the 21-gene assay by simulating a hypothetical cohort of 50-year-old women over a lifetime horizon using discrete event simulation. Primary model outcomes were life-years, quality-adjusted life-years (QALYs), costs, and incremental cost-effectiveness ratios (ICERs). We followed the International Society for Pharmacoeconomics and Outcomes Research-Society for Medical Decision Making (ISPOR-SMDM) best practice recommendations for validation and compared modeling results of the Oncotyrol model with the state-transition model developed by the Toronto Health Economics and Technology Assessment (THETA) Collaborative. Both models were populated with Canadian THETA model parameters, and outputs were compared. Results. The differences between the models varied among the different validation end points. The smallest relative differences were in costs, and the greatest were in QALYs. All relative differences were less than 1.2%. The cost-effectiveness plane showed that small differences in the model structure can lead to different sets of nondominated test-treatment strategies with different efficiency frontiers. We faced several challenges: distinguishing between differences in outcomes due to different modeling techniques and initial coding errors, defining meaningful differences, and selecting measures and statistics for comparison (means, distributions, multivariate outcomes). Conclusions. Cross-model validation was crucial to identify and correct coding errors and to explain differences in model outcomes. In our comparison, small differences in either QALYs or costs led to changes in ICERs because of changes in the set of dominated and nondominated strategies.
更多查看译文
关键词
cross-model validation,decision analysis,discrete event simulation,Markov models,state-transition model,cost-effectiveness analysis
AI 理解论文
溯源树
样例
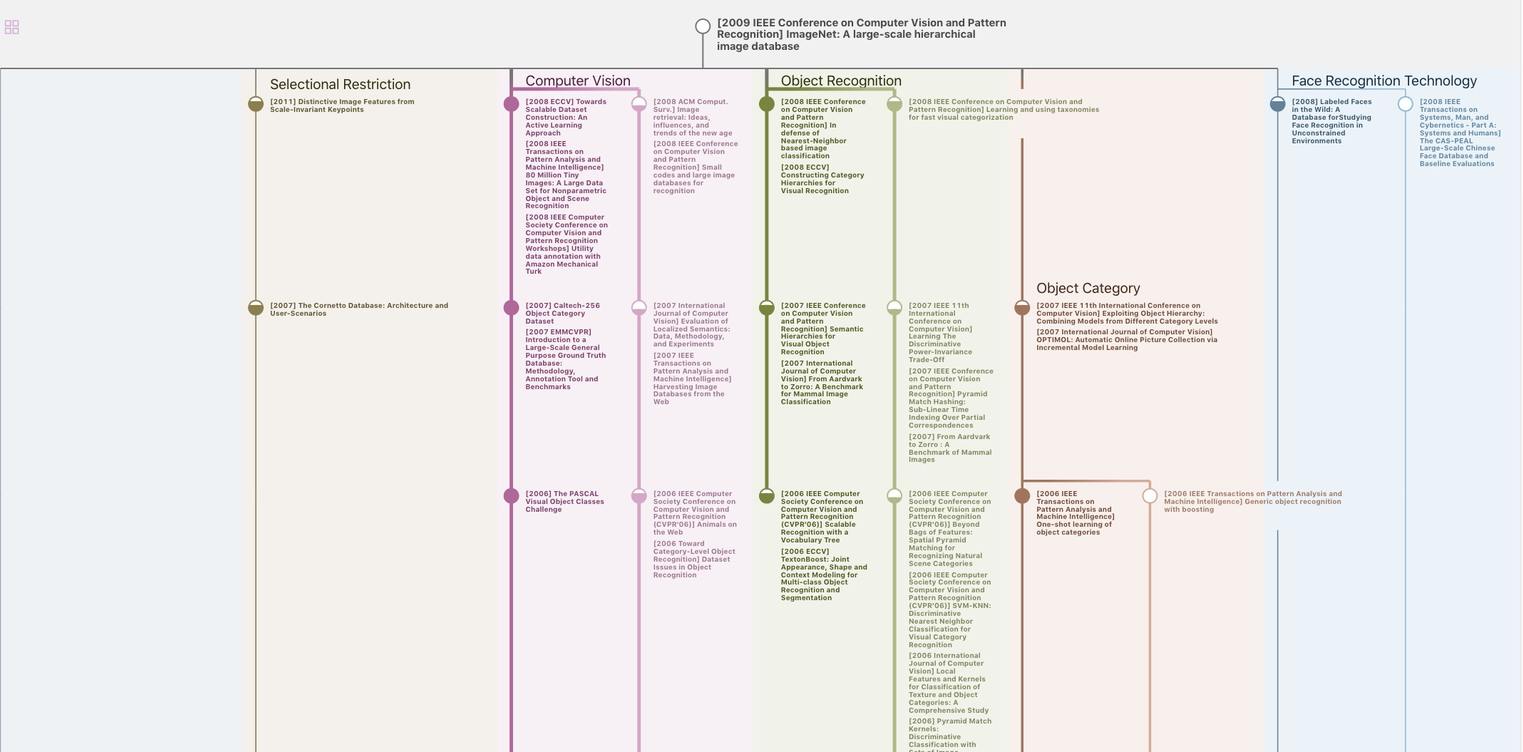
生成溯源树,研究论文发展脉络
Chat Paper
正在生成论文摘要