|[ldquo]|Validation|[rdquo]| of Outcome Measures in Dermatology
Journal of Investigative Dermatology(2013)
Abstract
Outcome measures are powerful tools in a clinician’s armamentarium. These instruments capture clinical information and may supplement clinical judgment in order to optimize management approach, medical treatment, and referrals to other appropriate health-care providers. They may shed light on psychosocial issues while providing insight into gaps in understanding not previously considered by the clinician or the patient. These tools highlight variability between diseases when using the same scoring system and may influence clinical guideline recommendations. Additionally, these instruments may influence policy directed toward allocation of limited resources, playing a significant role in future strategies aimed at cost-effectiveness. Scores, scales, profiles, and indexes are all examples of outcome measures. Outcome measures typically attempt to quantify either (i) clinical disease severity or (ii) patient-reported outcomes. Clinical disease severity–assessment tools gauge the global extent of disease, such as percentage of body surface area affected, physician global assessment, or the characteristics of isolated skin lesions. More disease-specific tools are the Psoriasis Area and Severity Index and the Scoring Atopic Dermatitis tool. Another group of tools focuses on patient-reported outcomes such as health-related quality of life (HRQoL), assessing the impact of a disease on patients’ lives or evaluating treatment preference/satisfaction. These instruments may be generic, allowing comparison across diseases (e.g., SF-36), dermatology specific (e.g., Dermatology Quality of Life index or Skindex), disease specific, or concept specific (e.g., stigmatization or anxiety). Figure 1 demonstrates the relationships among clinical disease severity measures, HRQoL tools, and therapeutic intervention data. Analytical treatment of an instrument has acquired the name “validation,” and it is the widely accepted method for evaluating the integrity of an instrument. The term “validation” is technically inappropriate because “validity” is only one of the axes or properties weighed. The evaluation of an instrument involves testing many properties, including validity, structure, reliability, and responsiveness. The outcome measure must first be developed (usually a questionnaire) and administered. Next, the tool undergoes property testing (statistical analysis) to determine the integrity of the tool based on the answers that are generated from initial test administration (Weller et al., 2012). Once this is accomplished with desirable results, the instrument can be used as a qualitative measure for use in a study and/or as a clinical decision–making tool. Because no gold standard exists against which to compare a tool, tools are judged on the basis of their behavior when tested for certain properties, such as structure, validity, reliability, and responsiveness. For example, for assessing HRQoL, the SF-36 and Skindex behave “well” when property-tested (Both et al., 2007). For instance, the Skindex includes constructs such as physical limitations and discomfort within the physical domain. Dimensions within the psychosocial domain include cognitive, social, and emotional disruptions, with the emotional dimension being further characterized by the constructs of depression, fear, embarrassment, and anger (Figure 2) (Chren et al., 1996). Items must be loaded onto a specific domain for instrument results to be appropriately interpreted. For example, the Skindex item “My skin hurts” is loaded onto the “discomfort” category, a component of the physical domain. Conversely, “I think about my skin condition” is loaded on the “cognitive” category, a psychosocial domain. Items may be loaded onto a construct in a variety of ways, ranging from objective to subjective. Content validity refers to the adequacy of the instrument to address all relevant items within a construct; this can be evaluated by the instrument respondents (e.g., patient). When assessed by experts, this is termed “face validity.” Convergent validity is achieved when a tool correlates well with tools that are supposed to measure the same underlying construct (e.g., a new tool assessing HRQoL in atopic eczema patients showed results similar to those obtained using an existing HRQoL tool). The statistical test used is the correlation coefficient. Construct validity is tested by hypothesizing that different groups of patients show differences in scores as expected. For example, patients with severe disease should exhibit higher levels of HRQoL impairment than those for patients with mild disease. If this is confirmed by the outcome-measure tool, it will suggest optimal construct validity (Prinsen et al., 2013). Internal consistency can be tested after one application of an instrument and examines the degree to which the set of items measures the same construct; this is measured by Cronbach’s α test. For example, an α > 0.7 suggests that the item is not highly correlated with the other items in the scale, suggesting it measures a different aspect of the disease. Conversely, an α > 0.9 suggests item redundancy, and the construct is being overemphasized and overrepresented within the instrument (Prinsen et al., 2013). Responsiveness refers to the instrument’s capability to change when the patient experiences a change in disease state. Responsiveness addresses whether a tool is sensitive enough to detect changes in patients. For example, the score should be lower after a successful therapeutic intervention than it was prior to treatment. The important question is whether the change in impairment reflects a statistically significant change while actually affecting the patient. To assess this, the minimal clinical important difference can be estimated (Revicki et al., 2008). Response distribution assesses whether the entire range of the item scores is being utilized. If more than 70% of patients score an item “0” (or any other score), then this item may not discriminate between patients and may be removed from the scale. Overall, the distribution of scores can also be measurement indicators. For example, the Psoriasis Area and Severity Index instrument curve is skewed right, underrepresenting patients who suffer from mild disease because the instrument is not as sensitive to detection of disease in this range (Spuls et al., 2010). Finally, other axes of instrument evaluation lie in identifying biases based on culture and language, as well as practical issues ranging from respondent burden (is the tool too long?) to administrative burdens, e.g., means of administration (verbal, over the phone, via computer) and data collection (Spuls et al., 2010). There are currently no guidelines for development or appropriate testing of intended health measurement within an outcome measure. The Consensus-based Standards for the Selection of Health Measurement Instruments study represents initial research in the development of a provider checklist to address this need in assessing different health-related, patient-reported outcomes using the Delphi procedure. This process includes sequential questionnaires, or “rounds,” with controlled feedback to gain consensus by a group of experts. It is favorable where there is a lack of empirical evidence, yet it is able to incorporate responses from leaders in many health-care fields of expertise (Mokkink et al., 2010). Thus, health-care providers may use this checklist to select appropriate measurement tools for patient feedback and optimal health-care outcomes. With more than 50 proposed outcome measures for patients with psoriasis, how can dermatologists determine which measurement tool is appropriate for their patients (Spuls et al., 2010)? The health-care provider must understand how to administer, as well as interpret the results of, an outcome measure for effective utilization while recognizing the limitations of each tool. Factors including study setting, disease manifestation, and patient type must also be considered when choosing an optimal outcome measure. Provider exposure and training are critical to a better understanding of outcome measures and their role in determining the extent of disease burden in order to assist dermatologists in providing optimal patient care. This article has been approved for 1 hour of Category 1 CME Credit. To take the online quiz, follow the link below: http://www.classmarker.com/online-test/start/?quiz=yxk51dc7bff36258 The authors state no conflict of interest. SUPPLEMENTARY MATERIAL Answers and a PowerPoint slide presentation appropriate for journal club or other teaching exercises are available at http://dx.doi.org/10.1038/jid.2013.332.
MoreTranslated text
Key words
cutaneous biology, skin disease, psoriasis, dermatitis, keratinocyte, melanocyte, skin cancer, dendritic cells, epidermis
AI Read Science
Must-Reading Tree
Example
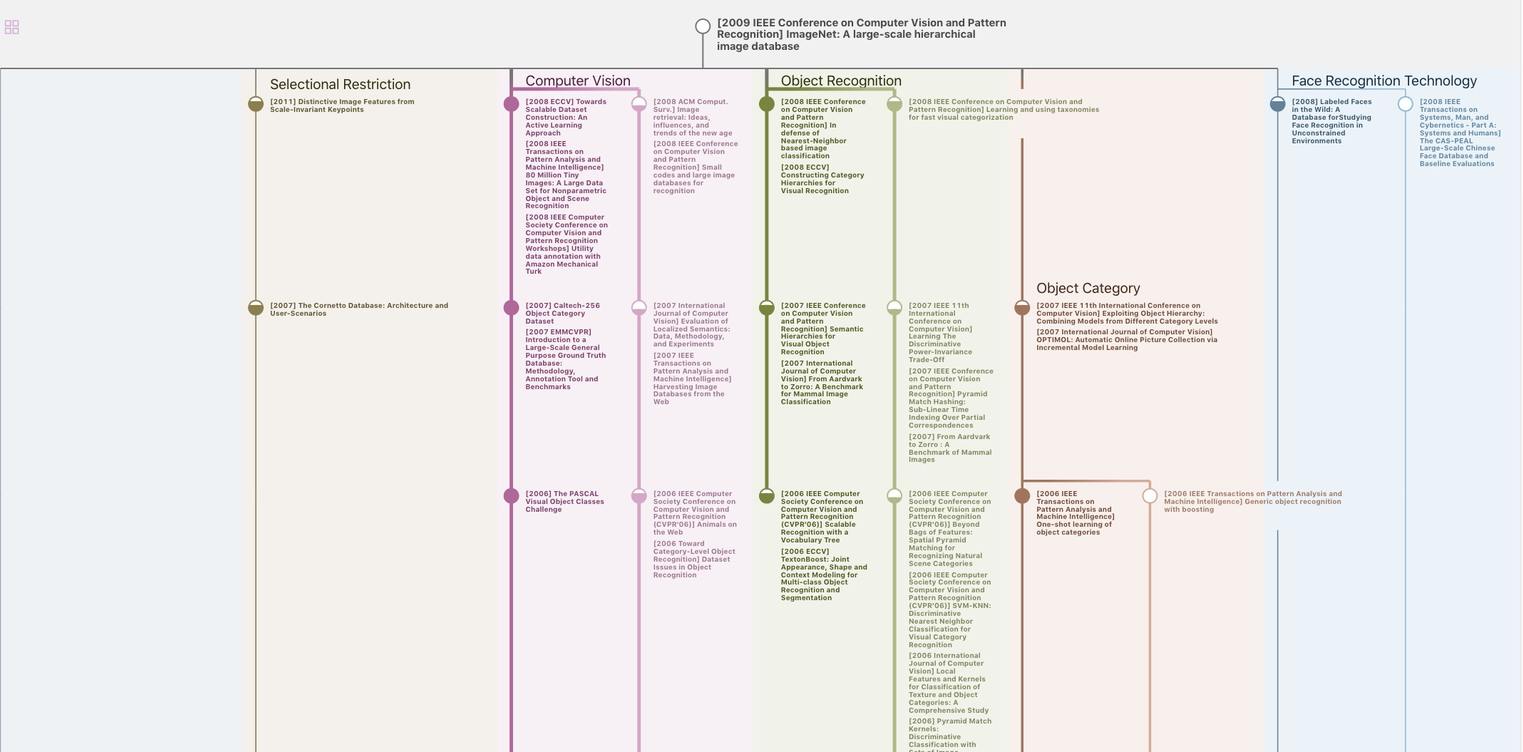
Generate MRT to find the research sequence of this paper
Chat Paper
Summary is being generated by the instructions you defined