Bayesian Constrained Local Models Revisited
Pattern Analysis and Machine Intelligence, IEEE Transactions(2016)
摘要
This paper presents a novel Bayesian formulation for aligning faces in unseen images. Our approach revisits the Constrained Local Models (CLM) formulation where an ensemble of local feature detectors are constrained to lie within the subspace spanned by a Point Distribution Model (PDM). Fitting such a model to an image typically involves two main steps: a local search using a detector, obtaining response maps for each landmark (likelihood term) and a global optimization that finds the PDM parameters that jointly maximize all the detections at once. The so-called global optimization can be posed as a Bayesian inference problem, where the posterior distribution of the shape (and pose) parameters can be inferred in a maximum a posteriori (MAP) sense. This work introduces an extended Bayesian global optimization strategy that includes two novel additions: (1) to perform second order updates of the PDM parameters (accounting for their covariance) and (2) to model the underlying dynamics of the shape variations, encoded in the prior term, by using recursive Bayesian estimation. Extensive evaluations were performed against state-of-the-art methods on several standard datasets (IMM, BioID, XM2VTS, LFW and FGNET Talking Face). Results show that the proposed approach significantly increases the fitting performance.
更多查看译文
关键词
Active Shape Models (ASM),Constrained Local Models (CLM),Non-rigid face alignment,face registration
AI 理解论文
溯源树
样例
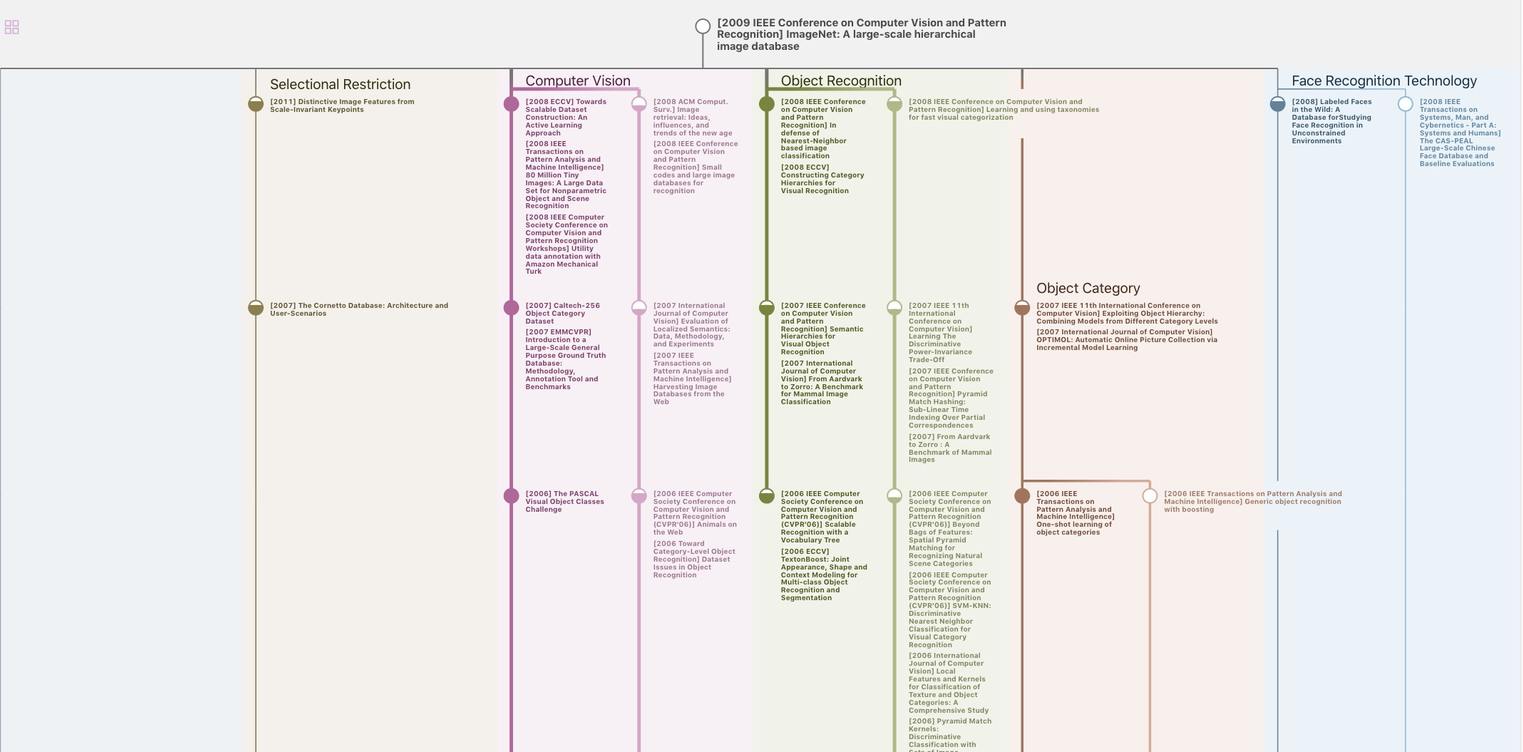
生成溯源树,研究论文发展脉络
Chat Paper
正在生成论文摘要