Quantifying Genomic Privacy Via Inference Attack With High-Order Snv Correlations
2015 IEEE SECURITY AND PRIVACY WORKSHOPS (SPW)(2015)
摘要
As genomic data becomes widely used, the problem of genomic data privacy becomes a hot interdisciplinary research topic among geneticists, bioinformaticians and security and privacy experts. Practical attacks have been identified on genomic data, and thus break the privacy expectations of individuals who contribute their genomic data to medical research, or simply share their data online. Frustrating as it is, the problem could become even worse. Existing genomic privacy breaches rely on low-order SNV (Single Nucleotide Variant) correlations. Our work shows that far more powerful attacks can be designed if high-order correlations are utilized. We corroborate this concern by making use of different SNV correlations based on various genomic data models and applying them to an inference attack on individuals' genotype data with hidden SNVs. We also show that low-order models behave very differently from real genomic data and therefore should not be relied upon for privacy-preserving solutions.
更多查看译文
关键词
SNV correlation,genomic privacy,high order,inference attack,
AI 理解论文
溯源树
样例
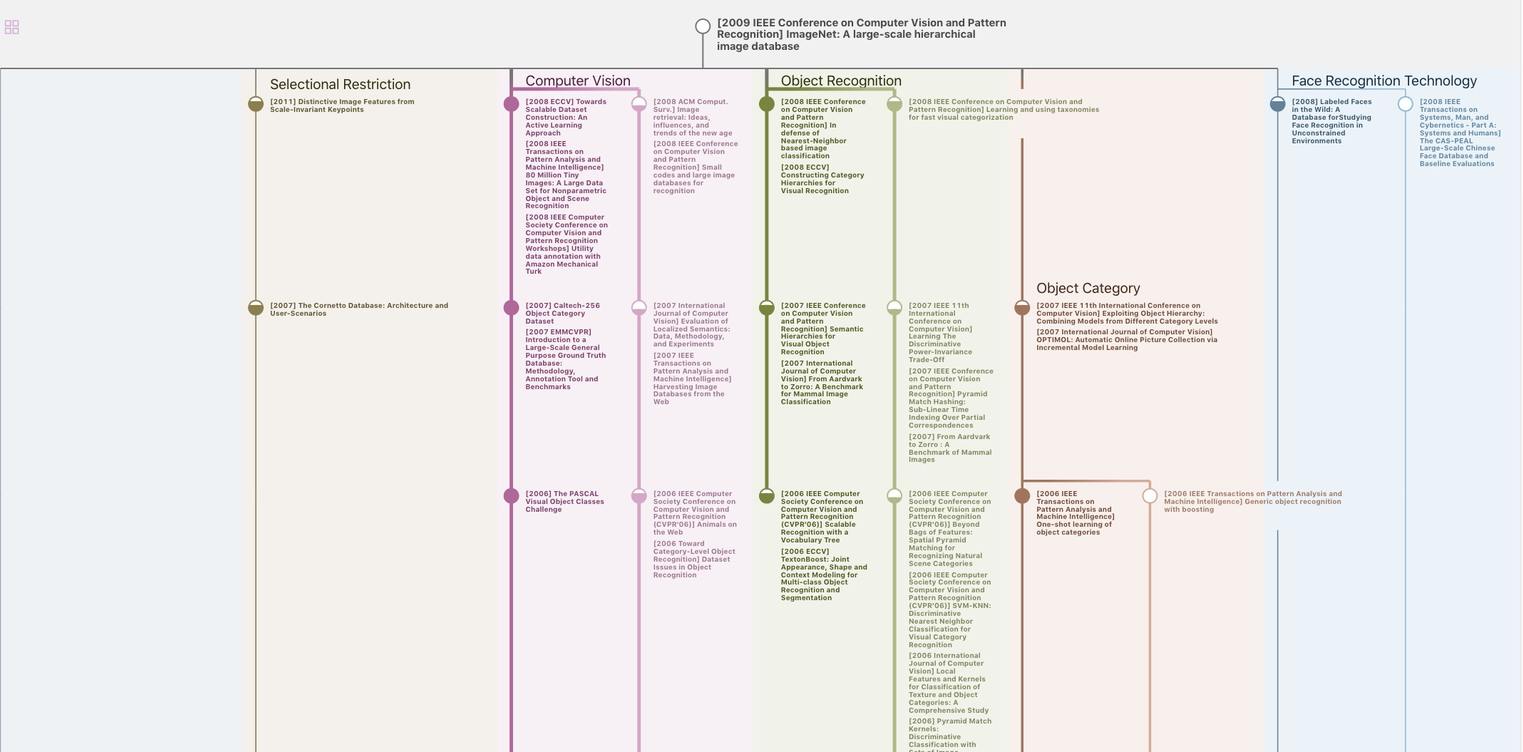
生成溯源树,研究论文发展脉络
Chat Paper
正在生成论文摘要