Learning tensegrity locomotion using open-loop control signals and coevolutionary algorithms.
Artificial Life(2015)
摘要
Soft robots offer many advantages over traditional rigid robots. However, soft robots can be difficult to control with standard control methods. Fortunately, evolutionary algorithms can offer an elegant solution to this problem. Instead of creating controls to handle the intricate dynamics of these robots, we can simply evolve the controls using a simulation to provide an evaluation function. In this article, we show how such a control paradigm can be applied to an emerging field within soft robotics: robots based on tensegrity structures. We take the model of the Spherical Underactuated Planetary Exploration Robot ball (SUPERball), an icosahedron tensegrity robot under production at NASA Ames Research Center, develop a rolling locomotion algorithm, and study the learned behavior using an accurate model of the SUPERball simulated in the NASA Tensegrity Robotics Toolkit. We first present the historical-average fitness-shaping algorithm for coevolutionary algorithms to speed up learning while favoring robustness over optimality. Second, we use a distributed control approach by coevolving open-loop control signals for each controller. Being simple and distributed, open-loop controllers can be readily implemented on SUPERball hardware without the need for sensor information or precise coordination. We analyze signals of different complexities and frequencies. Among the learned policies, we take one of the best and use it to analyze different aspects of the rolling gait, such as lengths, tensions, and energy consumption. We also discuss the correlation between the signals controlling different parts of the tensegrity robot.
更多查看译文
关键词
Evolutionary algorithms,tensegrity,locomotion,coevolution,fitness shaping
AI 理解论文
溯源树
样例
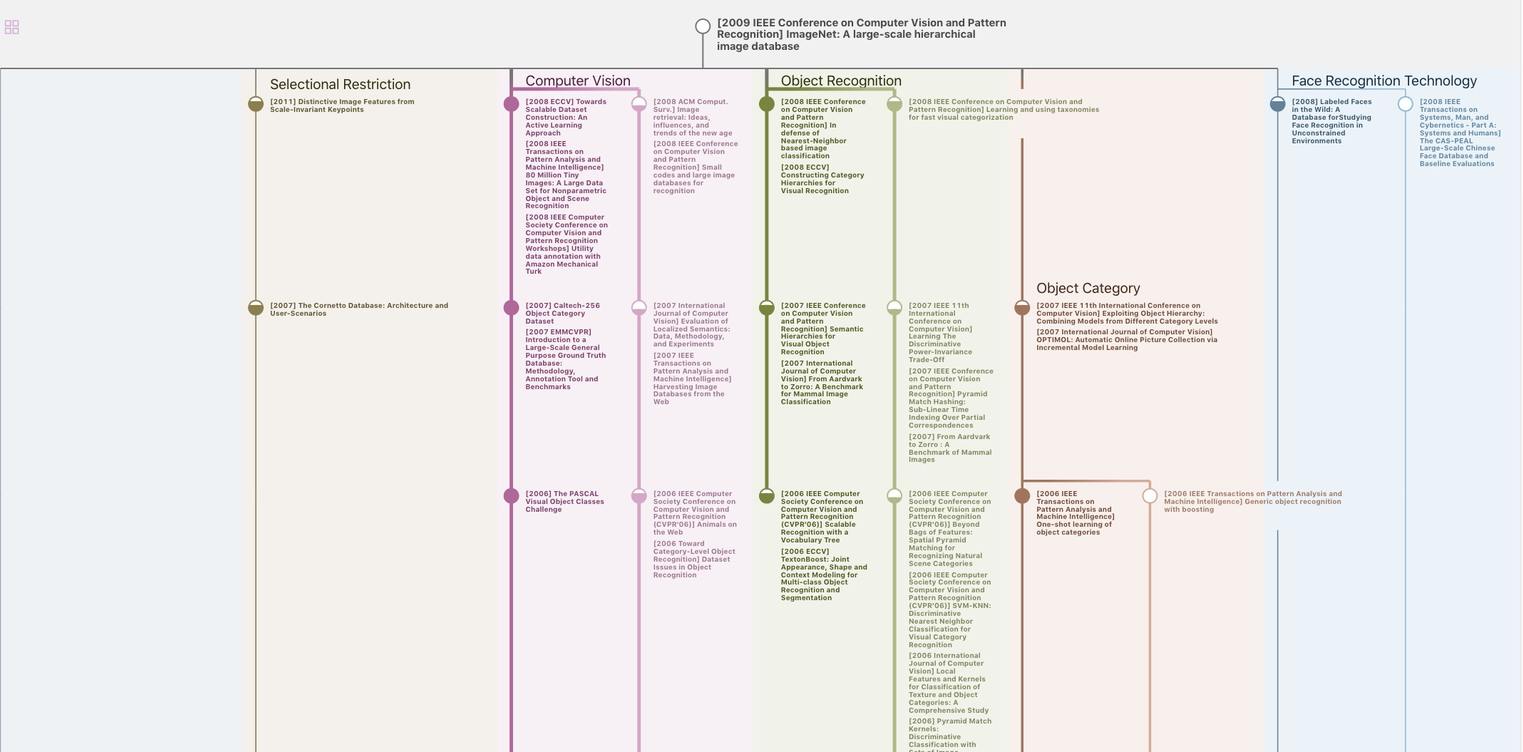
生成溯源树,研究论文发展脉络
Chat Paper
正在生成论文摘要