Research on Optical Remote Sensing Image Target Detection Technique Based on DCH-YOLOv7 Algorithm
IEEE ACCESS(2024)
摘要
Aiming at the YOLO (You Only Look Once) algorithm's low detection accuracy caused by the complex background environment and large target scale difference in the detection of optical remote sensing images, the Deformable Convolutional Fusion Attention mechanism based DCH-YOLOv7 (Deformable Convolutional Hybrid-YOLOv7) target detection algorithm is proposed in this paper. In this algorithm, deformable convolution is introduced in order to meet the detection of optical remote sensing images with different scale, and at the same time, two modules, PELAN and PMP, are added to effectively improve the network's ability to accurately localize the target features; secondly, a hybrid attention module (ACmix) is used, which effectively enhances the network's sensitivity to the small targets and improves the detection accuracy; lastly, the CIoU loss function is replaced by the WIoU loss function, which, through the adjustment of the weights, improves the detection accuracy of the high-quality anchor frames, and reduces the probability of missed and false detection. Finally, experiments were conducted on publicly available datasets, namely DIOR. Experimental results indicate that the DCH-YOLOv7 algorithm achieved an impressive detection accuracy of 90.6% in mAP@0.5, demonstrating a remarkable improvement of 3.1% over YOLOv7. These results demonstrate that DCH-YOLOv7 algorithm has a certain improvement in the effectiveness of target detection in optical remote sensing imagery, and can better cope with the problems of the dense distribution of small targets, the large differences in target scales, and the complex background.
更多查看译文
关键词
Target detection,deformable convolution,attention mechanism,loss function
AI 理解论文
溯源树
样例
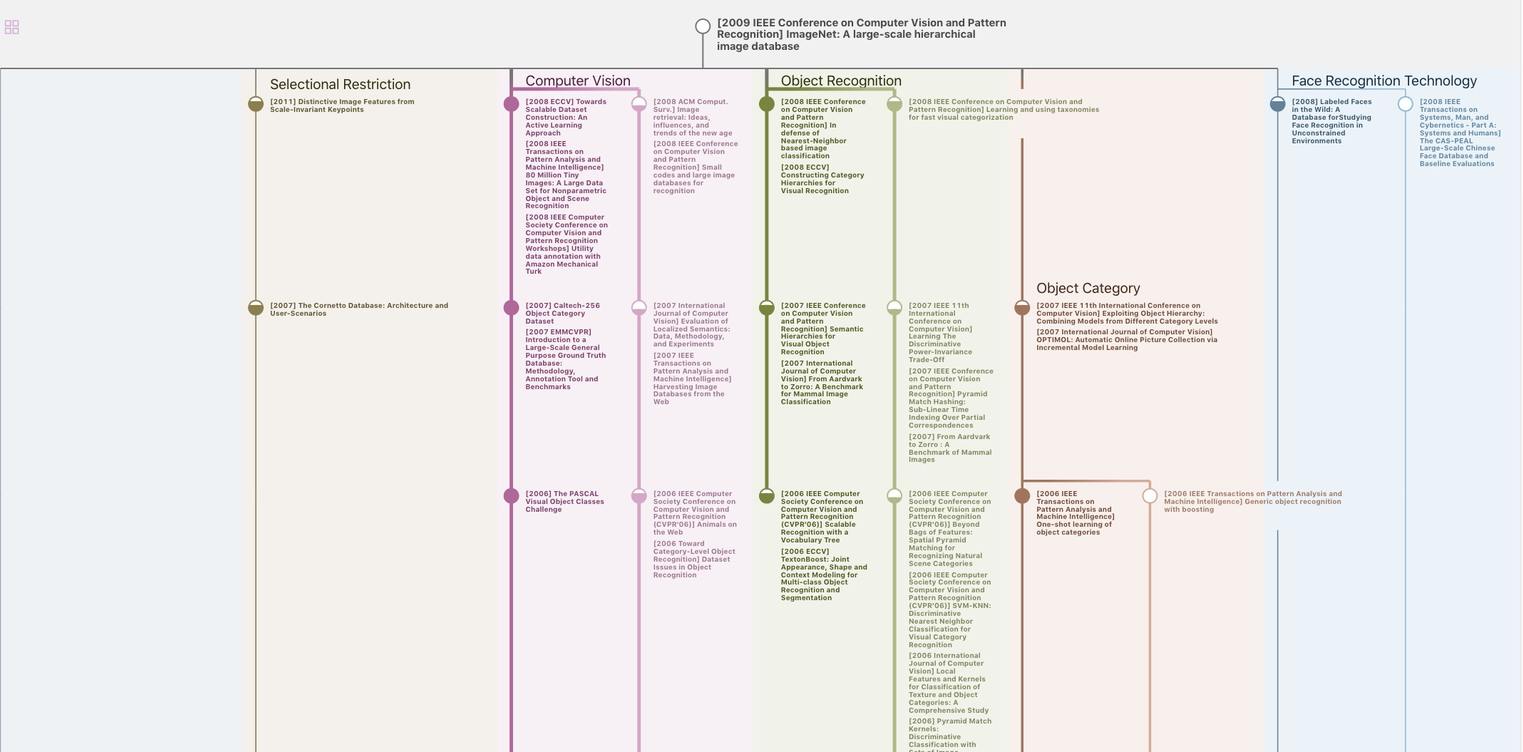
生成溯源树,研究论文发展脉络
Chat Paper
正在生成论文摘要