Medical image editing in the latent space of Generative Adversarial Networks
Intelligence-Based Medicine(2021)
Abstract
We consider a set of arithmetic operations in the latent space of Generative Adversarial Networks (GANs) to edit histopathological images. We analyze thousands of image patches from whole-slide images of breast cancer metastases in histological lymph node sections. Image files were downloaded from the pathology contests CAMELYON 16 and 17. We show that widely known architectures, such as: Deep Convolutional Generative Adversarial Networks (DCGAN) and Conditional Deep Convolutional Generative Adversarial Networks (cDCGAN), allow image editing using semantic concepts that represent underlying visual patterns in histopathological images, expanding GAN's well-known capabilities in medical image editing. We computed the Grad-cam heatmap of real positive images and of generated positive images, validating that the highlighted features both in the real and synthetic images match. We also show that GANs can be used to generate quality images, making GANs a valuable resource for augmenting small medical imaging datasets.
MoreTranslated text
Key words
Deep learning,Generative adversarial networks,Medical image editing,Histopathological images
AI Read Science
Must-Reading Tree
Example
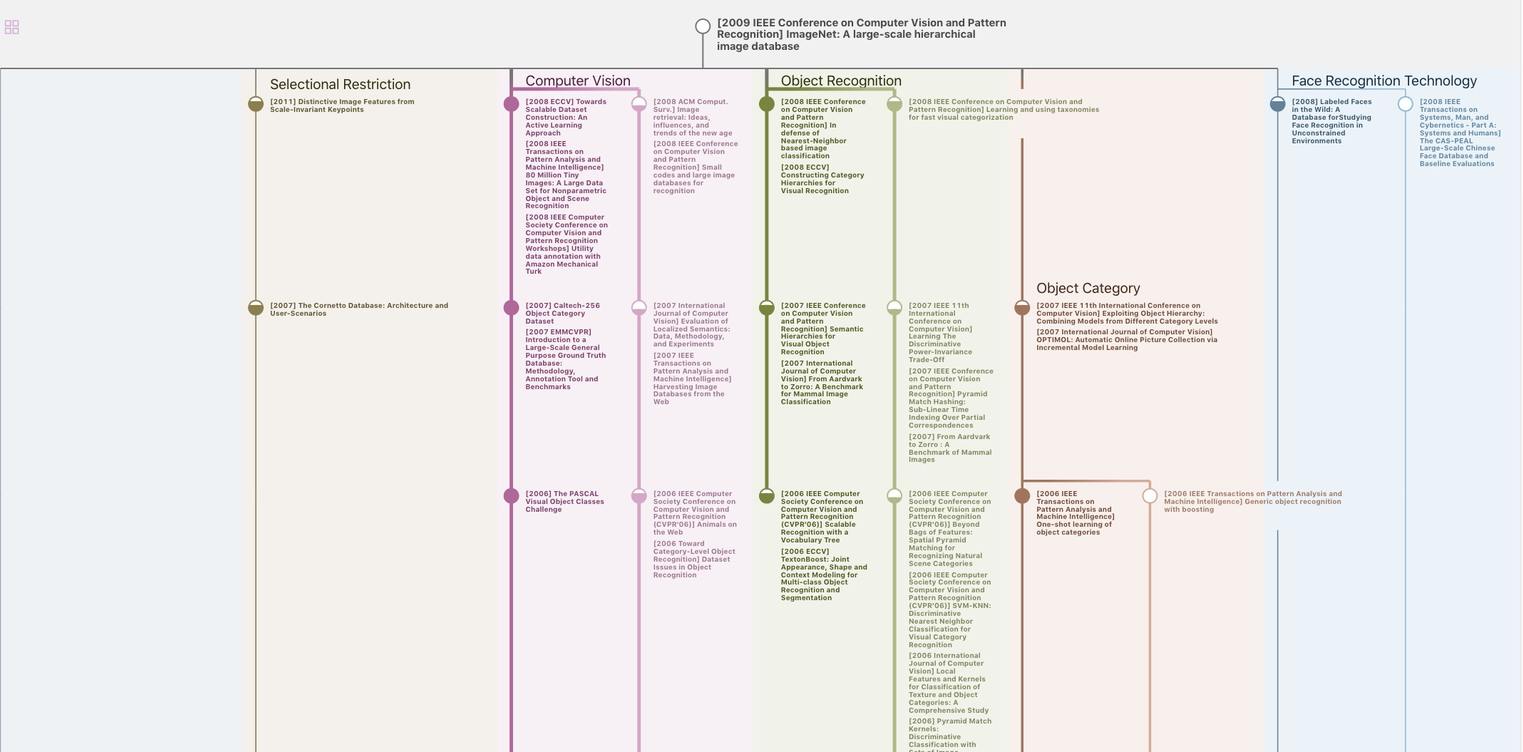
Generate MRT to find the research sequence of this paper
Chat Paper
Summary is being generated by the instructions you defined