A case study of conditional deep convolutional generative adversarial networks in machine fault diagnosis
JOURNAL OF INTELLIGENT MANUFACTURING(2020)
摘要
Due to the real working conditions, the collected mechanical fault datasets are actually limited and always highly imbalanced, which restricts the diagnosis accuracy and stability. To solve these problems, we present an imbalanced fault diagnosis method based on the generative model of conditional-deep convolutional generative adversarial network (C-DCGAN) and provide a study in detail. Deep convolutional generative adversarial network (DCGAN), based on traditional generative adversarial networks (GAN), introduces convolutional neural network into the training for unsupervised learning to improve the effect of generative networks. Conditional generative adversarial network (CGAN) is a conditional model obtained through introducing conditional extension into GAN. C-DCGAN is a combination of DCGAN and CGAN. In C-DCGAN, based on the feature extraction ability of convolutional networks, through the structural optimization, conditional auxiliary generative samples are used as augmented data and applied in machine fault diagnosis. Two datasets (Bearing dataset and Planetary gear box dataset) are carried out to validate. The simulation experiments showed that the improved performance is mainly due to the generated signals from C-DCGAN to balance the dataset. The proposed method can deal with imbalanced fault classification problem much more effectively. This model could improve the accuracy of fault diagnosis and the generalization ability of the classifier in the case of small samples and display better fault diagnosis performance.
更多查看译文
关键词
Generative adversarial network, Convolutional neural network, Conditional model, Data augmentation, Machine fault diagnosis
AI 理解论文
溯源树
样例
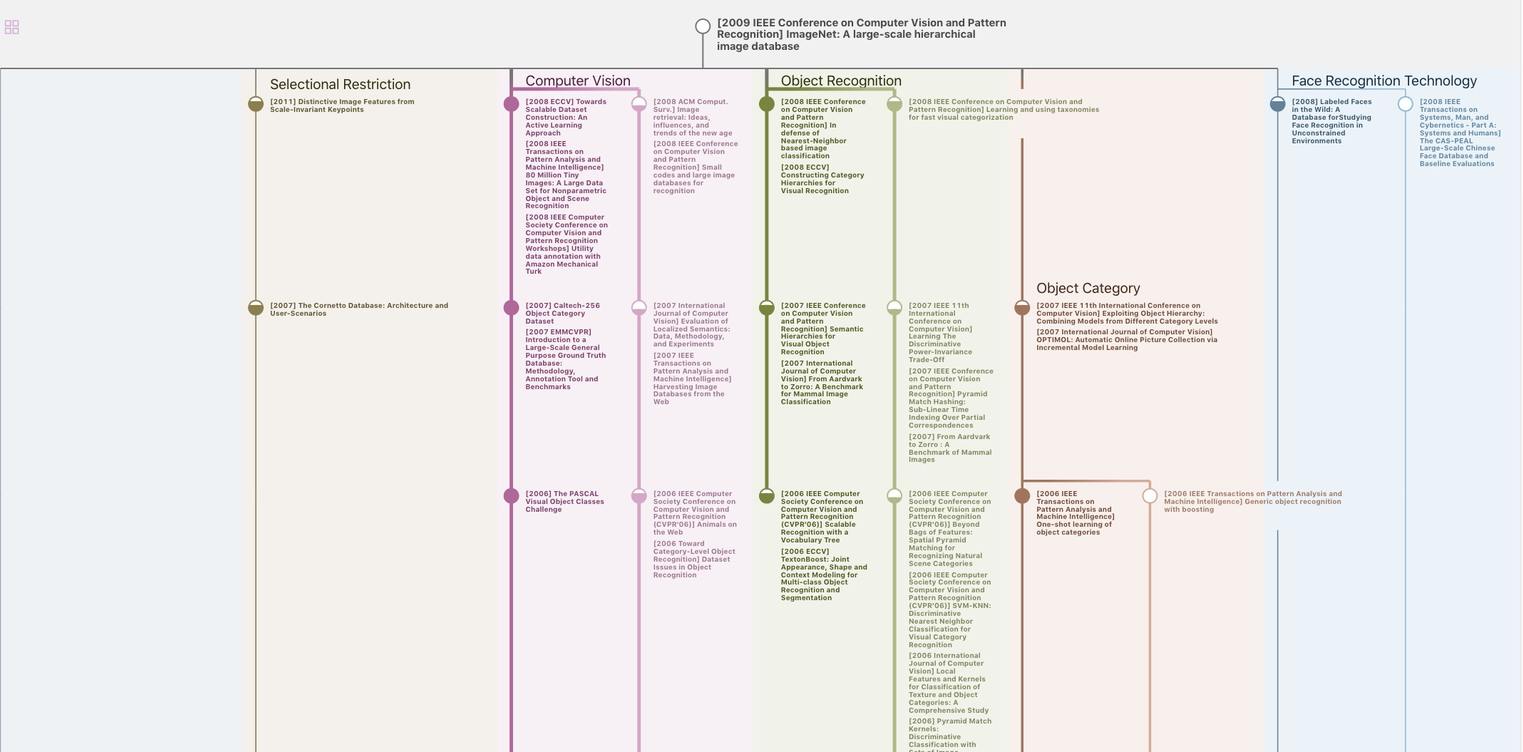
生成溯源树,研究论文发展脉络
Chat Paper
正在生成论文摘要