Smart anomaly detection in sensor systems: A multi-perspective review
Information Fusion(2021)
摘要
Anomaly detection is concerned with identifying data patterns that deviate remarkably from the expected behavior. This is an important research problem, due to its broad set of application domains, from data analysis to e-health, cybersecurity, predictive maintenance, fault prevention, and industrial automation. Herein, we review state-of-the-art methods that may be employed to detect anomalies in the specific area of sensor systems, which poses hard challenges in terms of information fusion, data volumes, data speed, and network/energy efficiency, to mention but the most pressing ones. In this context, anomaly detection is a particularly hard problem, given the need to find computing-energy-accuracy trade-offs in a constrained environment. We taxonomize methods ranging from conventional techniques (statistical methods, time-series analysis, signal processing, etc.) to data-driven techniques (supervised learning, reinforcement learning, deep learning, etc.). We also look at the impact that different architectural environments (Cloud, Fog, Edge) can have on the sensors ecosystem. The review points to the most promising intelligent-sensing methods, and pinpoints a set of interesting open issues and challenges.
更多查看译文
关键词
Anomaly detection,Machine learning,Sensor systems,Internet of Things,Intelligent sensing
AI 理解论文
溯源树
样例
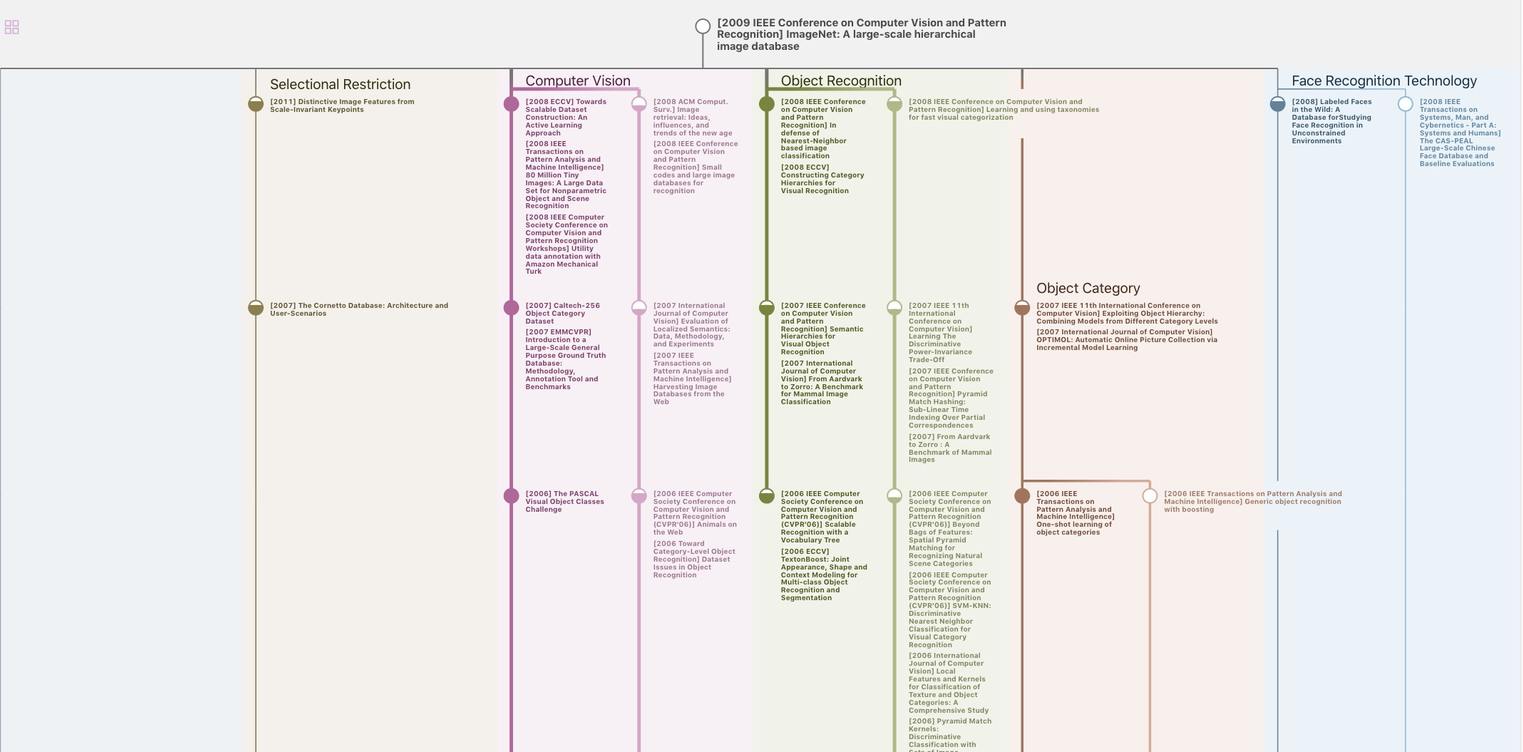
生成溯源树,研究论文发展脉络
Chat Paper
正在生成论文摘要