Parametric modeling of whole-genome sequencing data for CNV identification.
BIOSTATISTICS(2014)
摘要
Copy number variants (CNVs) constitute an important class of genetic variants in human genome and are shown to be associated with complex diseases. Whole-genome sequencing provides an unbiased way of identifying all the CNVs that an individual carries. In this paper, we consider parametric modeling of the read depth (RD) data from whole-genome sequencing with the aim of identifying the CNVs, including both Poisson and negative-binomial modeling of such count data. We propose a unified approach of using a mean-matching variance stabilizing transformation to turn the relatively complicated problem of sparse segment identification for count data into a sparse segment identification problem for a sequence of Gaussian data. We apply the optimal sparse segment identification procedure to the transformed data in order to identify the CNV segments. This provides a computationally efficient approach for RD-based CNV identification. Simulation results show that this approach often results in a small number of false identifications of the CNVs and has similar or better performances in identifying the true CNVs when compared with other RD-based approaches. We demonstrate the methods using the trio data from the 1000 Genomes Project.
更多查看译文
关键词
Natural exponential family,Sparse segment identification,Variance stabilization
AI 理解论文
溯源树
样例
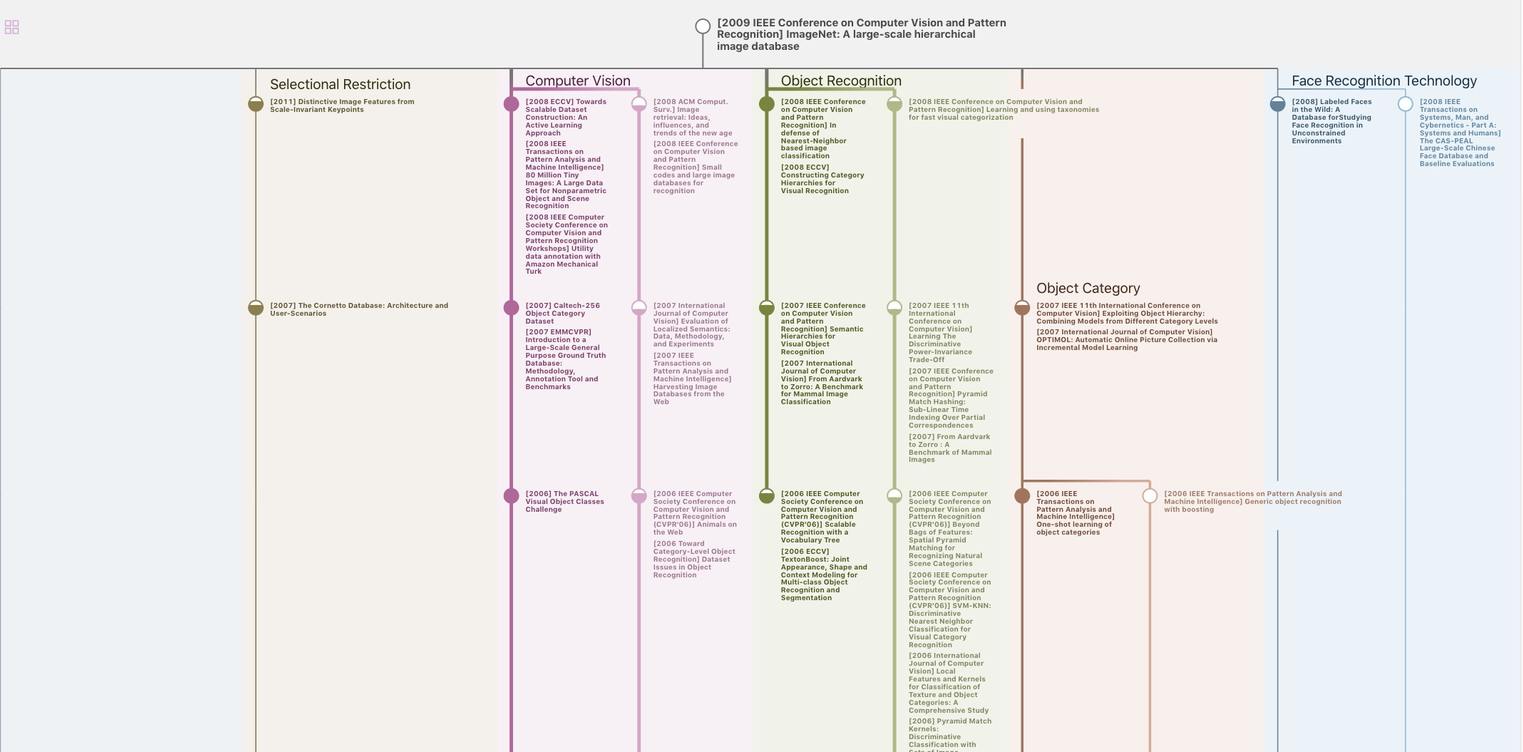
生成溯源树,研究论文发展脉络
Chat Paper
正在生成论文摘要