Human-in-the-Loop (HITL) Augmented Growing Neural Gas for Labeling Aerial Image Datasets
2023 IEEE INTERNATIONAL CONFERENCE ON FUZZY SYSTEMS, FUZZ(2023)
摘要
There is a severe demand for, and shortage of, large accurately labeled datasets to train supervised computational intelligence (CI) algorithms in domains like unmanned aerial systems (UAS) and autonomous vehicles. This has hindered our ability to develop and deploy various computer vision algorithms in/across environments and niche domains for tasks like detection, localization, and tracking. Herein, we propose a new human-in-the-loop (HITL) based growing neural gas (GNG) algorithm to minimize human intervention during labeling large UAS data collections over a shared geospatial area. Specifically, we address human driven events like new class identification and mistake correction. We also address algorithm-centric operations like new pattern discovery and self-supervised labeling. The effectiveness of our algorithm is demonstrated using simulated realistic ray-traced low altitude UAS data from the Unreal Engine. Our results show that it is possible to increase speed and reduce mental fatigue over hand labeling large image datasets.
更多查看译文
关键词
self-supervised learning,human-in-the-loop,growing neural gas,possibilistic nearest neighbor,UAS,UAV
AI 理解论文
溯源树
样例
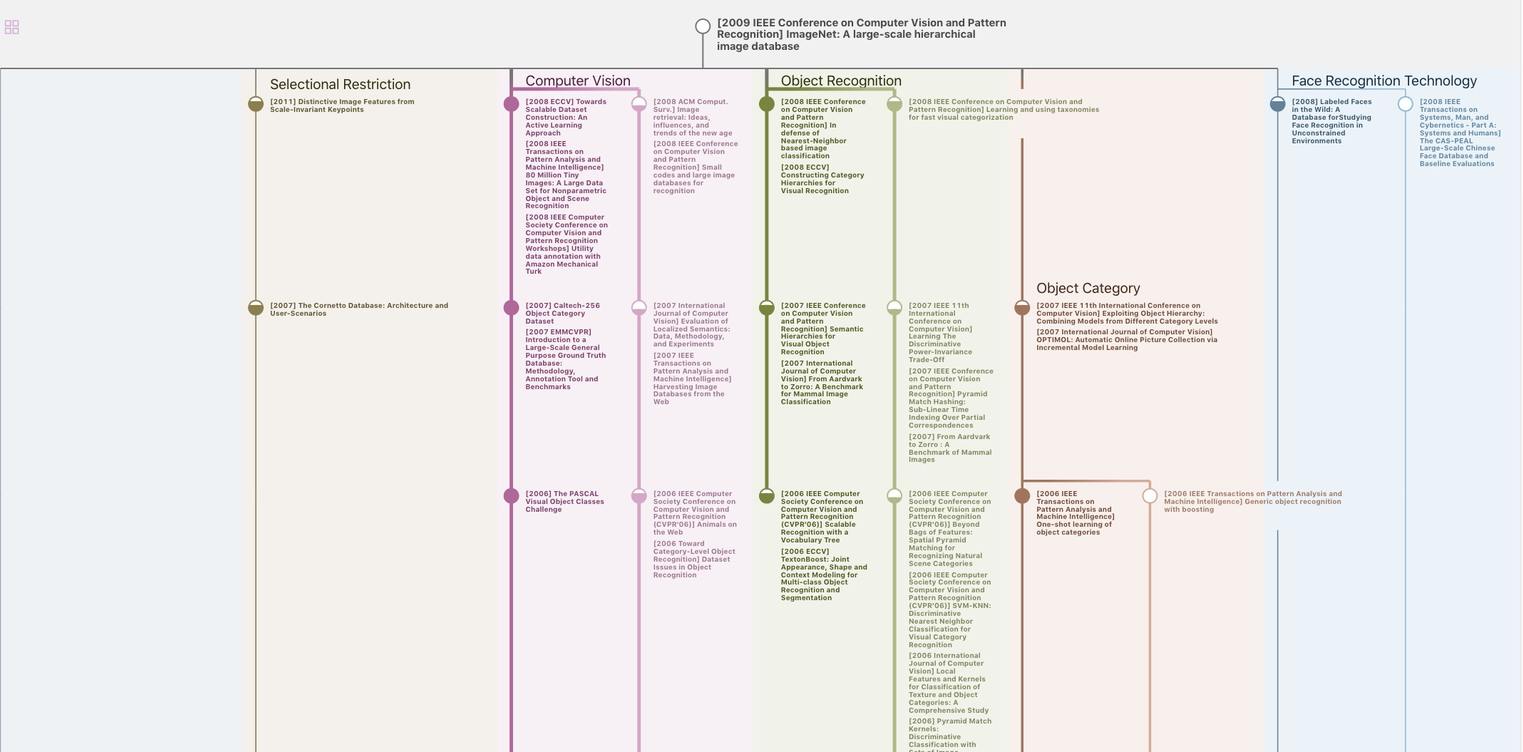
生成溯源树,研究论文发展脉络
Chat Paper
正在生成论文摘要